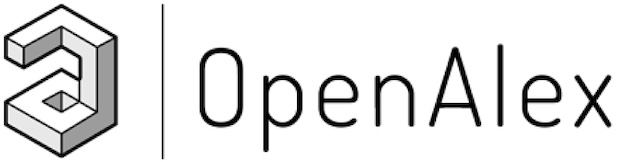
OpenAlex is a bibliographic catalogue of scientific papers, authors and institutions accessible in open access mode, named after the Library of Alexandria. It's citation coverage is excellent and I hope you will find utility in this listing of citing articles!
If you click the article title, you'll navigate to the article, as listed in CrossRef. If you click the Open Access links, you'll navigate to the "best Open Access location". Clicking the citation count will open this listing for that article. Lastly at the bottom of the page, you'll find basic pagination options.
Requested Article:
A practical PINN framework for multi-scale problems with multi-magnitude loss terms
Yong Wang, Yanzhong Yao, Jiawei Guo, et al.
Journal of Computational Physics (2024) Vol. 510, pp. 113112-113112
Open Access | Times Cited: 16
Yong Wang, Yanzhong Yao, Jiawei Guo, et al.
Journal of Computational Physics (2024) Vol. 510, pp. 113112-113112
Open Access | Times Cited: 16
Showing 16 citing articles:
3DMeshNet: A three-dimensional differential neural network for structured mesh generation
Jiaming Peng, Xinhai Chen, Jie Liu
Graphical Models (2025) Vol. 139, pp. 101257-101257
Closed Access | Times Cited: 1
Jiaming Peng, Xinhai Chen, Jie Liu
Graphical Models (2025) Vol. 139, pp. 101257-101257
Closed Access | Times Cited: 1
Constrained or unconstrained? Neural-network-based equation discovery from data
Grant Norman, Jacqueline Wentz, Hemanth Kolla, et al.
Computer Methods in Applied Mechanics and Engineering (2025) Vol. 436, pp. 117684-117684
Open Access
Grant Norman, Jacqueline Wentz, Hemanth Kolla, et al.
Computer Methods in Applied Mechanics and Engineering (2025) Vol. 436, pp. 117684-117684
Open Access
A framework of parallel physics-informed neural network with Laplace transform for well testing interpretation
Dongyan Fan, Can Yang, Dongyan Fan, et al.
Physics of Fluids (2025) Vol. 37, Iss. 1
Closed Access
Dongyan Fan, Can Yang, Dongyan Fan, et al.
Physics of Fluids (2025) Vol. 37, Iss. 1
Closed Access
A physics-informed neural network-based method for dispersion calculations
Zhibao Cheng, Tianxiang Yu, Gaofeng Jia, et al.
International Journal of Mechanical Sciences (2025), pp. 110111-110111
Closed Access
Zhibao Cheng, Tianxiang Yu, Gaofeng Jia, et al.
International Journal of Mechanical Sciences (2025), pp. 110111-110111
Closed Access
Physics-informed neural networks for Kelvin–Helmholtz instability with spatiotemporal and magnitude multiscale
Jiahao Wu, Yuxin Wu, Xin Li, et al.
Physics of Fluids (2025) Vol. 37, Iss. 3
Closed Access
Jiahao Wu, Yuxin Wu, Xin Li, et al.
Physics of Fluids (2025) Vol. 37, Iss. 3
Closed Access
Unsupervised learning with physics informed graph networks for partial differential equations
Lin Lu, Yiye Zou, J.H. Wang, et al.
Applied Intelligence (2025) Vol. 55, Iss. 7
Closed Access
Lin Lu, Yiye Zou, J.H. Wang, et al.
Applied Intelligence (2025) Vol. 55, Iss. 7
Closed Access
Improved physics-informed neural network in mitigating gradient-related failures
Pancheng Niu, Jun Guo, Yongming Chen, et al.
Neurocomputing (2025), pp. 130167-130167
Closed Access
Pancheng Niu, Jun Guo, Yongming Chen, et al.
Neurocomputing (2025), pp. 130167-130167
Closed Access
IPFLSTM: Enhancing physics-informed neural networks with LSTM and Informer for efficient long-term prediction of dynamic multiphysics fields
Chen Bai, Quan Qian
Computational Materials Science (2025) Vol. 253, pp. 113874-113874
Closed Access
Chen Bai, Quan Qian
Computational Materials Science (2025) Vol. 253, pp. 113874-113874
Closed Access
Unveiling the optimization process of Physics Informed Neural Networks: How accurate and competitive can PINNs be?
Jorge F. Urbán, Petros Stefanou, J. A. Pons
Journal of Computational Physics (2024), pp. 113656-113656
Open Access | Times Cited: 4
Jorge F. Urbán, Petros Stefanou, J. A. Pons
Journal of Computational Physics (2024), pp. 113656-113656
Open Access | Times Cited: 4
Online multi-scenario impedance spectra generation for batteries based on small-sample learning
Jiajun Zhu, Xin Lai, Xiaopeng Tang, et al.
Cell Reports Physical Science (2024) Vol. 5, Iss. 8, pp. 102134-102134
Open Access | Times Cited: 3
Jiajun Zhu, Xin Lai, Xiaopeng Tang, et al.
Cell Reports Physical Science (2024) Vol. 5, Iss. 8, pp. 102134-102134
Open Access | Times Cited: 3
Physics-specialized neural network with hard constraints for solving multi-material diffusion problems
Yuchen Xie, Honghang Chi, Yahui Wang, et al.
Computer Methods in Applied Mechanics and Engineering (2024) Vol. 430, pp. 117223-117223
Closed Access | Times Cited: 1
Yuchen Xie, Honghang Chi, Yahui Wang, et al.
Computer Methods in Applied Mechanics and Engineering (2024) Vol. 430, pp. 117223-117223
Closed Access | Times Cited: 1
Integration and Innovation of Higher Education Management and Student Training Mechanisms Based on Multi-scale Feature Fusion
Yihui Hao
Applied Mathematics and Nonlinear Sciences (2024) Vol. 9, Iss. 1
Open Access
Yihui Hao
Applied Mathematics and Nonlinear Sciences (2024) Vol. 9, Iss. 1
Open Access
Physics-Informed Neural Network for the Calculation of Complex Dispersion Relations of Periodic Structures
Zhibao Cheng, Tianxiang Yu, Gaofeng Jia, et al.
(2024)
Closed Access
Zhibao Cheng, Tianxiang Yu, Gaofeng Jia, et al.
(2024)
Closed Access
Neuromorphic, physics-informed spiking neural network for molecular dynamics
Vuong Van Pham, Temoor Muther, Amirmasoud Kalantari Dahaghi
Machine Learning Science and Technology (2024) Vol. 5, Iss. 4, pp. 045079-045079
Open Access
Vuong Van Pham, Temoor Muther, Amirmasoud Kalantari Dahaghi
Machine Learning Science and Technology (2024) Vol. 5, Iss. 4, pp. 045079-045079
Open Access
wbPINN: Weight balanced physics-informed neural networks for multi-objective learning
Fujun Cao, Xiaobin Guo, Xinzheng Dong, et al.
Applied Soft Computing (2024), pp. 112632-112632
Closed Access
Fujun Cao, Xiaobin Guo, Xinzheng Dong, et al.
Applied Soft Computing (2024), pp. 112632-112632
Closed Access
An extrapolation-driven network architecture for physics-informed deep learning
Yong Wang, Yanzhong Yao, Zhiming Gao
Neural Networks (2024) Vol. 183, pp. 106998-106998
Open Access
Yong Wang, Yanzhong Yao, Zhiming Gao
Neural Networks (2024) Vol. 183, pp. 106998-106998
Open Access