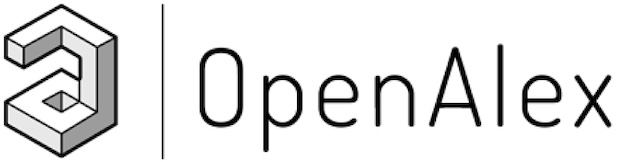
OpenAlex is a bibliographic catalogue of scientific papers, authors and institutions accessible in open access mode, named after the Library of Alexandria. It's citation coverage is excellent and I hope you will find utility in this listing of citing articles!
If you click the article title, you'll navigate to the article, as listed in CrossRef. If you click the Open Access links, you'll navigate to the "best Open Access location". Clicking the citation count will open this listing for that article. Lastly at the bottom of the page, you'll find basic pagination options.
Requested Article:
A fully Bayesian sparse polynomial chaos expansion approach with joint priors on the coefficients and global selection of terms
Paul‐Christian Bürkner, Ilja Kröker, Sergey Oladyshkin, et al.
Journal of Computational Physics (2023) Vol. 488, pp. 112210-112210
Open Access | Times Cited: 8
Paul‐Christian Bürkner, Ilja Kröker, Sergey Oladyshkin, et al.
Journal of Computational Physics (2023) Vol. 488, pp. 112210-112210
Open Access | Times Cited: 8
Showing 8 citing articles:
A hybrid polynomial chaos expansion – Gaussian process regression method for Bayesian uncertainty quantification and sensitivity analysis
Paolo Manfredi
Computer Methods in Applied Mechanics and Engineering (2025) Vol. 436, pp. 117693-117693
Open Access | Times Cited: 1
Paolo Manfredi
Computer Methods in Applied Mechanics and Engineering (2025) Vol. 436, pp. 117693-117693
Open Access | Times Cited: 1
Uncertainty quantification and propagation in surrogate-based Bayesian inference
Philipp Reiser, Javier Enrique Aguilar, Anneli Guthke, et al.
Statistics and Computing (2025) Vol. 35, Iss. 3
Open Access
Philipp Reiser, Javier Enrique Aguilar, Anneli Guthke, et al.
Statistics and Computing (2025) Vol. 35, Iss. 3
Open Access
Nonparametric formulation of polynomial chaos expansion based on least-square support-vector machines
Paolo Manfredi, Riccardo Trinchero
Engineering Applications of Artificial Intelligence (2024) Vol. 133, pp. 108182-108182
Open Access | Times Cited: 3
Paolo Manfredi, Riccardo Trinchero
Engineering Applications of Artificial Intelligence (2024) Vol. 133, pp. 108182-108182
Open Access | Times Cited: 3
Some models are useful, but how do we know which ones? Towards a unified Bayesian model taxonomy
Paul‐Christian Bürkner, Maximilian Scholz, Stefan T. Radev
Statistics Surveys (2023) Vol. 17, Iss. none
Open Access | Times Cited: 9
Paul‐Christian Bürkner, Maximilian Scholz, Stefan T. Radev
Statistics Surveys (2023) Vol. 17, Iss. none
Open Access | Times Cited: 9
Contaminant source identification in an aquifer using a Bayesian framework with arbitrary polynomial chaos expansion
Guodong Zhang, Teng Xu, Chunhui Lu, et al.
Stochastic Environmental Research and Risk Assessment (2024) Vol. 38, Iss. 5, pp. 2007-2018
Closed Access | Times Cited: 1
Guodong Zhang, Teng Xu, Chunhui Lu, et al.
Stochastic Environmental Research and Risk Assessment (2024) Vol. 38, Iss. 5, pp. 2007-2018
Closed Access | Times Cited: 1
Shrinkage-based Bayesian variable selection for species distribution modelling in complex environments: An application to urban biodiversity
Andreas Dietzel, Marco Moretti, Lauren M. Cook
Ecological Informatics (2024) Vol. 81, pp. 102561-102561
Open Access | Times Cited: 1
Andreas Dietzel, Marco Moretti, Lauren M. Cook
Ecological Informatics (2024) Vol. 81, pp. 102561-102561
Open Access | Times Cited: 1
The deep arbitrary polynomial chaos neural network or how Deep Artificial Neural Networks could benefit from data-driven homogeneous chaos theory
Sergey Oladyshkin, Timothy Praditia, Ilja Kroeker, et al.
Neural Networks (2023) Vol. 166, pp. 85-104
Open Access | Times Cited: 3
Sergey Oladyshkin, Timothy Praditia, Ilja Kroeker, et al.
Neural Networks (2023) Vol. 166, pp. 85-104
Open Access | Times Cited: 3