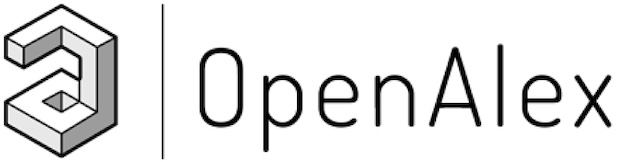
OpenAlex is a bibliographic catalogue of scientific papers, authors and institutions accessible in open access mode, named after the Library of Alexandria. It's citation coverage is excellent and I hope you will find utility in this listing of citing articles!
If you click the article title, you'll navigate to the article, as listed in CrossRef. If you click the Open Access links, you'll navigate to the "best Open Access location". Clicking the citation count will open this listing for that article. Lastly at the bottom of the page, you'll find basic pagination options.
Requested Article:
Identification of suicidality in adolescent major depressive disorder patients using sMRI: A machine learning approach.
Su Hong, Yang S. Liu, Bo Cao, et al.
Journal of Affective Disorders (2020) Vol. 280, pp. 72-76
Closed Access | Times Cited: 48
Su Hong, Yang S. Liu, Bo Cao, et al.
Journal of Affective Disorders (2020) Vol. 280, pp. 72-76
Closed Access | Times Cited: 48
Showing 1-25 of 48 citing articles:
Machine learning and the prediction of suicide in psychiatric populations: a systematic review
Alessandro Pigoni, Giuseppe Delvecchio, Nunzio Turtulici, et al.
Translational Psychiatry (2024) Vol. 14, Iss. 1
Open Access | Times Cited: 20
Alessandro Pigoni, Giuseppe Delvecchio, Nunzio Turtulici, et al.
Translational Psychiatry (2024) Vol. 14, Iss. 1
Open Access | Times Cited: 20
An insight into diagnosis of depression using machine learning techniques: a systematic review
Sweta Bhadra, Chandan Jyoti Kumar
Current Medical Research and Opinion (2022) Vol. 38, Iss. 5, pp. 749-771
Closed Access | Times Cited: 51
Sweta Bhadra, Chandan Jyoti Kumar
Current Medical Research and Opinion (2022) Vol. 38, Iss. 5, pp. 749-771
Closed Access | Times Cited: 51
Enhancing the efficacy of depression detection system using optimal feature selection from EHR
Sweta Bhadra, Chandan Jyoti Kumar
Computer Methods in Biomechanics & Biomedical Engineering (2023) Vol. 27, Iss. 2, pp. 222-236
Closed Access | Times Cited: 26
Sweta Bhadra, Chandan Jyoti Kumar
Computer Methods in Biomechanics & Biomedical Engineering (2023) Vol. 27, Iss. 2, pp. 222-236
Closed Access | Times Cited: 26
Understanding complex functional wiring patterns in major depressive disorder through brain functional connectome
Zhiyun Yang, Lingyu Jian, Hui Qiu, et al.
Translational Psychiatry (2021) Vol. 11, Iss. 1
Open Access | Times Cited: 37
Zhiyun Yang, Lingyu Jian, Hui Qiu, et al.
Translational Psychiatry (2021) Vol. 11, Iss. 1
Open Access | Times Cited: 37
Application of Artificial Intelligence in the MRI Classification Task of Human Brain Neurological and Psychiatric Diseases: A Scoping Review
Zhao Zhang, Guangfei Li, Yong Xu, et al.
Diagnostics (2021) Vol. 11, Iss. 8, pp. 1402-1402
Open Access | Times Cited: 36
Zhao Zhang, Guangfei Li, Yong Xu, et al.
Diagnostics (2021) Vol. 11, Iss. 8, pp. 1402-1402
Open Access | Times Cited: 36
Identification of suicidality in patients with major depressive disorder via dynamic functional network connectivity signatures and machine learning
Manxi Xu, Xiaojing Zhang, Yanqing Li, et al.
Translational Psychiatry (2022) Vol. 12, Iss. 1
Open Access | Times Cited: 23
Manxi Xu, Xiaojing Zhang, Yanqing Li, et al.
Translational Psychiatry (2022) Vol. 12, Iss. 1
Open Access | Times Cited: 23
Identifying suicide attempts, ideation, and non-ideation in major depressive disorder from structural MRI data using deep learning
Jinlong Hu, Yangmin Huang, Xiaojing Zhang, et al.
Asian Journal of Psychiatry (2023) Vol. 82, pp. 103511-103511
Closed Access | Times Cited: 14
Jinlong Hu, Yangmin Huang, Xiaojing Zhang, et al.
Asian Journal of Psychiatry (2023) Vol. 82, pp. 103511-103511
Closed Access | Times Cited: 14
Classification of suicidality by training supervised machine learning models with brain MRI findings: A systematic review
Mohammadamin Parsaei, Fateme Taghavizanjani, Giulia Cattarinussi, et al.
Journal of Affective Disorders (2023) Vol. 340, pp. 766-791
Closed Access | Times Cited: 14
Mohammadamin Parsaei, Fateme Taghavizanjani, Giulia Cattarinussi, et al.
Journal of Affective Disorders (2023) Vol. 340, pp. 766-791
Closed Access | Times Cited: 14
Correlates of suicidality in young people with depressive disorders: A systematic review
Carl I. Moller, Christopher G. Davey, Paul B. Badcock, et al.
Australian & New Zealand Journal of Psychiatry (2022) Vol. 56, Iss. 8, pp. 910-948
Closed Access | Times Cited: 20
Carl I. Moller, Christopher G. Davey, Paul B. Badcock, et al.
Australian & New Zealand Journal of Psychiatry (2022) Vol. 56, Iss. 8, pp. 910-948
Closed Access | Times Cited: 20
Polygonum sibiricum polysaccharides alleviate chronic unpredictable mild stress-induced depressive-like behaviors by regulating the gut microbiota composition and SCFAs levels
Yingyu Zhang, Yuan Sun, Yupei Liu, et al.
Journal of Functional Foods (2023) Vol. 101, pp. 105411-105411
Open Access | Times Cited: 12
Yingyu Zhang, Yuan Sun, Yupei Liu, et al.
Journal of Functional Foods (2023) Vol. 101, pp. 105411-105411
Open Access | Times Cited: 12
Differential gray matter correlates and machine learning prediction of abuse and internalizing psychopathology in adolescent females
Sara A. Heyn, Taylor J. Keding, Josh M. Cisler, et al.
Scientific Reports (2025) Vol. 15, Iss. 1
Open Access
Sara A. Heyn, Taylor J. Keding, Josh M. Cisler, et al.
Scientific Reports (2025) Vol. 15, Iss. 1
Open Access
The Potential Impact of Adjunct Digital Tools and Technology to Help Distressed and Suicidal Men: An Integrative Review
Luke Balcombe, Diego De Leo
Frontiers in Psychology (2022) Vol. 12
Open Access | Times Cited: 17
Luke Balcombe, Diego De Leo
Frontiers in Psychology (2022) Vol. 12
Open Access | Times Cited: 17
Childhood trauma and social support affect symptom profiles through cortical thickness abnormalities in major depressive disorder: A structural equation modeling analysis
Chenguang Jiang, Wenhao Jiang, Gang Chen, et al.
Asian Journal of Psychiatry (2023) Vol. 88, pp. 103744-103744
Closed Access | Times Cited: 9
Chenguang Jiang, Wenhao Jiang, Gang Chen, et al.
Asian Journal of Psychiatry (2023) Vol. 88, pp. 103744-103744
Closed Access | Times Cited: 9
Effectiveness of artificial intelligence in detecting and managing depressive disorders: Systematic review
Yoonseo Park, Sewon Park, Munjae Lee
Journal of Affective Disorders (2024) Vol. 361, pp. 445-456
Closed Access | Times Cited: 3
Yoonseo Park, Sewon Park, Munjae Lee
Journal of Affective Disorders (2024) Vol. 361, pp. 445-456
Closed Access | Times Cited: 3
A review and meta-analysis of machine intelligence approaches for mental health issues and depression detection
Ravita Chahar, Ashutosh Kumar Dubey, Sushil Kumar Narang
International Journal of Advanced Technology and Engineering Exploration (2021) Vol. 8, Iss. 83
Open Access | Times Cited: 18
Ravita Chahar, Ashutosh Kumar Dubey, Sushil Kumar Narang
International Journal of Advanced Technology and Engineering Exploration (2021) Vol. 8, Iss. 83
Open Access | Times Cited: 18
Machine learning based identification of structural brain alterations underlying suicide risk in adolescents
Sahil Bajaj, Karina S. Blair, Matthew Dobbertin, et al.
Discover Mental Health (2023) Vol. 3, Iss. 1
Open Access | Times Cited: 6
Sahil Bajaj, Karina S. Blair, Matthew Dobbertin, et al.
Discover Mental Health (2023) Vol. 3, Iss. 1
Open Access | Times Cited: 6
Pain avoidance and functional connectivity between insula and amygdala identifies suicidal attempters in patients with major depressive disorder using machine learning
Ziyu Hao, Huanhuan Li, Lisheng Ouyang, et al.
Psychophysiology (2022) Vol. 60, Iss. 1
Closed Access | Times Cited: 10
Ziyu Hao, Huanhuan Li, Lisheng Ouyang, et al.
Psychophysiology (2022) Vol. 60, Iss. 1
Closed Access | Times Cited: 10
Incidence Trends and Risk Prediction Nomogram for Suicidal Attempts in Patients With Major Depressive Disorder
Sixiang Liang, Jinhe Zhang, Qian Zhao, et al.
Frontiers in Psychiatry (2021) Vol. 12
Open Access | Times Cited: 14
Sixiang Liang, Jinhe Zhang, Qian Zhao, et al.
Frontiers in Psychiatry (2021) Vol. 12
Open Access | Times Cited: 14
A Systematic Review of MRI Studies and the “Emotional paiN and social Disconnect (END)” Brain Model of Suicidal Behavior in Youth
Olga Tymofiyeva, Katherine W. Reeves, Chace Shaw, et al.
Behavioural Neurology (2023) Vol. 2023, pp. 1-21
Open Access | Times Cited: 4
Olga Tymofiyeva, Katherine W. Reeves, Chace Shaw, et al.
Behavioural Neurology (2023) Vol. 2023, pp. 1-21
Open Access | Times Cited: 4
Identifying suicide attempter in major depressive disorder through machine learning: the importance of pain avoidance, event-related potential features of affective processing
Huanhuan Li, Shijie Wei, Fang Sun, et al.
Cerebral Cortex (2024) Vol. 34, Iss. 4
Open Access | Times Cited: 1
Huanhuan Li, Shijie Wei, Fang Sun, et al.
Cerebral Cortex (2024) Vol. 34, Iss. 4
Open Access | Times Cited: 1
Predictive Models for Suicide Attempts in Major Depressive Disorder and the Contribution of EPHX2: A Pilot Integrative Machine Learning Study
Shuqiong Zheng, Weixiong Zeng, Qianyun Wu, et al.
Depression and Anxiety (2024) Vol. 2024, pp. 1-16
Open Access | Times Cited: 1
Shuqiong Zheng, Weixiong Zeng, Qianyun Wu, et al.
Depression and Anxiety (2024) Vol. 2024, pp. 1-16
Open Access | Times Cited: 1
Artificial Intelligence-Based Suicide Prevention and Prediction: A Systematic Review (2019-2023)
Anirudh Atmakuru, Alen Shahini, Subrata Chakraborty, et al.
(2024)
Closed Access | Times Cited: 1
Anirudh Atmakuru, Alen Shahini, Subrata Chakraborty, et al.
(2024)
Closed Access | Times Cited: 1
Impulsivity in ADHD and Borderline Personality Disorder: A Systematic Review of Gray and White Matter Variations
Łukasz Franczak, Piotr Podwalski, Patryk Wysocki, et al.
Journal of Clinical Medicine (2024) Vol. 13, Iss. 22, pp. 6906-6906
Open Access | Times Cited: 1
Łukasz Franczak, Piotr Podwalski, Patryk Wysocki, et al.
Journal of Clinical Medicine (2024) Vol. 13, Iss. 22, pp. 6906-6906
Open Access | Times Cited: 1
Aberrant Dynamic Functional Connectivity of Posterior Cingulate Cortex Subregions in Major Depressive Disorder With Suicidal Ideation
Weicheng Li, Chengyu Wang, Xiaofeng Lan, et al.
Frontiers in Neuroscience (2022) Vol. 16
Open Access | Times Cited: 7
Weicheng Li, Chengyu Wang, Xiaofeng Lan, et al.
Frontiers in Neuroscience (2022) Vol. 16
Open Access | Times Cited: 7
Suicide risk stratification among major depressed patients based on a machine learning approach and whole-brain functional connectivity
Shengli Chen, Xiaojing Zhang, Shiwei Lin, et al.
Journal of Affective Disorders (2022) Vol. 322, pp. 173-179
Closed Access | Times Cited: 7
Shengli Chen, Xiaojing Zhang, Shiwei Lin, et al.
Journal of Affective Disorders (2022) Vol. 322, pp. 173-179
Closed Access | Times Cited: 7