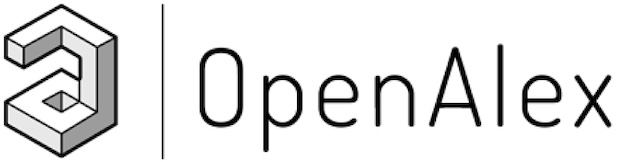
OpenAlex is a bibliographic catalogue of scientific papers, authors and institutions accessible in open access mode, named after the Library of Alexandria. It's citation coverage is excellent and I hope you will find utility in this listing of citing articles!
If you click the article title, you'll navigate to the article, as listed in CrossRef. If you click the Open Access links, you'll navigate to the "best Open Access location". Clicking the citation count will open this listing for that article. Lastly at the bottom of the page, you'll find basic pagination options.
Requested Article:
Day-ahead spatiotemporal wind speed forecasting using robust design-based deep learning neural network
Ying‐Yi Hong, Thursy Rienda Aulia Satriani
Energy (2020) Vol. 209, pp. 118441-118441
Closed Access | Times Cited: 93
Ying‐Yi Hong, Thursy Rienda Aulia Satriani
Energy (2020) Vol. 209, pp. 118441-118441
Closed Access | Times Cited: 93
Showing 1-25 of 93 citing articles:
A review of wind speed and wind power forecasting with deep neural networks
Yun Wang, Runmin Zou, Fang Liu, et al.
Applied Energy (2021) Vol. 304, pp. 117766-117766
Closed Access | Times Cited: 545
Yun Wang, Runmin Zou, Fang Liu, et al.
Applied Energy (2021) Vol. 304, pp. 117766-117766
Closed Access | Times Cited: 545
A review and taxonomy of wind and solar energy forecasting methods based on deep learning
Ghadah Alkhayat, Rashid Mehmood
Energy and AI (2021) Vol. 4, pp. 100060-100060
Open Access | Times Cited: 204
Ghadah Alkhayat, Rashid Mehmood
Energy and AI (2021) Vol. 4, pp. 100060-100060
Open Access | Times Cited: 204
Short-term wind power forecasting using the hybrid model of improved variational mode decomposition and Correntropy Long Short -term memory neural network
Jiandong Duan, Peng Wang, Wentao Ma, et al.
Energy (2020) Vol. 214, pp. 118980-118980
Closed Access | Times Cited: 177
Jiandong Duan, Peng Wang, Wentao Ma, et al.
Energy (2020) Vol. 214, pp. 118980-118980
Closed Access | Times Cited: 177
Machine Learning and Deep Learning in Energy Systems: A Review
Mohammad Mahdi Forootan, Iman Larki, Rahim Zahedi, et al.
Sustainability (2022) Vol. 14, Iss. 8, pp. 4832-4832
Open Access | Times Cited: 153
Mohammad Mahdi Forootan, Iman Larki, Rahim Zahedi, et al.
Sustainability (2022) Vol. 14, Iss. 8, pp. 4832-4832
Open Access | Times Cited: 153
A novel framework for ultra-short-term interval wind power prediction based on RF-WOA-VMD and BiGRU optimized by the attention mechanism
Min Yu, Dongxiao Niu, Tian Gao, et al.
Energy (2023) Vol. 269, pp. 126738-126738
Closed Access | Times Cited: 122
Min Yu, Dongxiao Niu, Tian Gao, et al.
Energy (2023) Vol. 269, pp. 126738-126738
Closed Access | Times Cited: 122
Forecasting Renewable Energy Generation with Machine Learning and Deep Learning: Current Advances and Future Prospects
Natei Ermias Benti, Mesfin Diro Chaka, Addisu Gezahegn Semie
Sustainability (2023) Vol. 15, Iss. 9, pp. 7087-7087
Open Access | Times Cited: 84
Natei Ermias Benti, Mesfin Diro Chaka, Addisu Gezahegn Semie
Sustainability (2023) Vol. 15, Iss. 9, pp. 7087-7087
Open Access | Times Cited: 84
Short-term wind power forecasting model based on temporal convolutional network and Informer
Mingju Gong, Changcheng Yan, Wei Xu, et al.
Energy (2023) Vol. 283, pp. 129171-129171
Closed Access | Times Cited: 48
Mingju Gong, Changcheng Yan, Wei Xu, et al.
Energy (2023) Vol. 283, pp. 129171-129171
Closed Access | Times Cited: 48
A short-term wind power forecasting method based on multivariate signal decomposition and variable selection
Ting Yang, Zhenning Yang, Fei Li, et al.
Applied Energy (2024) Vol. 360, pp. 122759-122759
Closed Access | Times Cited: 25
Ting Yang, Zhenning Yang, Fei Li, et al.
Applied Energy (2024) Vol. 360, pp. 122759-122759
Closed Access | Times Cited: 25
A combination forecasting model of wind speed based on decomposition
Zhongda Tian, Hao Li, Feihong Li
Energy Reports (2021) Vol. 7, pp. 1217-1233
Open Access | Times Cited: 77
Zhongda Tian, Hao Li, Feihong Li
Energy Reports (2021) Vol. 7, pp. 1217-1233
Open Access | Times Cited: 77
A novel loss function of deep learning in wind speed forecasting
Xi Chen, Ruyi Yu, Sajid Ullah, et al.
Energy (2021) Vol. 238, pp. 121808-121808
Closed Access | Times Cited: 65
Xi Chen, Ruyi Yu, Sajid Ullah, et al.
Energy (2021) Vol. 238, pp. 121808-121808
Closed Access | Times Cited: 65
Artificial Intelligence Based Hybrid Forecasting Approaches for Wind Power Generation: Progress, Challenges and Prospects
Molla Shahadat Hossain Lipu, Md. Sazal Miah, M. A. Hannan, et al.
IEEE Access (2021) Vol. 9, pp. 102460-102489
Open Access | Times Cited: 61
Molla Shahadat Hossain Lipu, Md. Sazal Miah, M. A. Hannan, et al.
IEEE Access (2021) Vol. 9, pp. 102460-102489
Open Access | Times Cited: 61
Short-term wind speed forecasting based on spatial-temporal graph transformer networks
Xiaoxin Pan, Long Wang, Zhongju Wang, et al.
Energy (2022) Vol. 253, pp. 124095-124095
Closed Access | Times Cited: 51
Xiaoxin Pan, Long Wang, Zhongju Wang, et al.
Energy (2022) Vol. 253, pp. 124095-124095
Closed Access | Times Cited: 51
Ash determination of coal flotation concentrate by analyzing froth image using a novel hybrid model based on deep learning algorithms and attention mechanism
Xiaolin Yang, Kefei Zhang, Chao Ni, et al.
Energy (2022) Vol. 260, pp. 125027-125027
Closed Access | Times Cited: 41
Xiaolin Yang, Kefei Zhang, Chao Ni, et al.
Energy (2022) Vol. 260, pp. 125027-125027
Closed Access | Times Cited: 41
A hybrid model with combined feature selection based on optimized VMD and improved multi-objective coati optimization algorithm for short-term wind power prediction
Chao Wang, Lin Hon, Heng Hu, et al.
Energy (2024) Vol. 293, pp. 130684-130684
Closed Access | Times Cited: 14
Chao Wang, Lin Hon, Heng Hu, et al.
Energy (2024) Vol. 293, pp. 130684-130684
Closed Access | Times Cited: 14
A dynamic modeling method using channel-selection convolutional neural network: A case study of NOx emission
Zhi Wang, Xianyong Peng, Huaichun Zhou, et al.
Energy (2024) Vol. 290, pp. 130270-130270
Closed Access | Times Cited: 11
Zhi Wang, Xianyong Peng, Huaichun Zhou, et al.
Energy (2024) Vol. 290, pp. 130270-130270
Closed Access | Times Cited: 11
A novel dynamic ensemble of numerical weather prediction for multi-step wind speed forecasting with deep reinforcement learning and error sequence modeling
Jing Zhao, Yiyi Guo, Yihua Lin, et al.
Energy (2024) Vol. 302, pp. 131787-131787
Closed Access | Times Cited: 8
Jing Zhao, Yiyi Guo, Yihua Lin, et al.
Energy (2024) Vol. 302, pp. 131787-131787
Closed Access | Times Cited: 8
A modified GM(1,1) model to accurately predict wind speed
Muhammad Uzair Yousuf, Ibrahim Al‐Bahadly, Ebubekir Avci
Sustainable Energy Technologies and Assessments (2020) Vol. 43, pp. 100905-100905
Closed Access | Times Cited: 53
Muhammad Uzair Yousuf, Ibrahim Al‐Bahadly, Ebubekir Avci
Sustainable Energy Technologies and Assessments (2020) Vol. 43, pp. 100905-100905
Closed Access | Times Cited: 53
Towards novel deep neuroevolution models: chaotic levy grasshopper optimization for short-term wind speed forecasting
Seyed Mohammad Jafar Jalali, Sajad Ahmadian, Mahdi Khodayar, et al.
Engineering With Computers (2021) Vol. 38, Iss. S3, pp. 1787-1811
Closed Access | Times Cited: 52
Seyed Mohammad Jafar Jalali, Sajad Ahmadian, Mahdi Khodayar, et al.
Engineering With Computers (2021) Vol. 38, Iss. S3, pp. 1787-1811
Closed Access | Times Cited: 52
Short-term wind speed forecasting system based on multivariate time series and multi-objective optimization
Zhihao Shang, Zhaoshuang He, Yao Chen, et al.
Energy (2021) Vol. 238, pp. 122024-122024
Closed Access | Times Cited: 52
Zhihao Shang, Zhaoshuang He, Yao Chen, et al.
Energy (2021) Vol. 238, pp. 122024-122024
Closed Access | Times Cited: 52
New hybrid approach for short-term wind speed predictions based on preprocessing algorithm and optimization theory
Weicheng Hu, Qingshan Yang, Hua-Peng Chen, et al.
Renewable Energy (2021) Vol. 179, pp. 2174-2186
Closed Access | Times Cited: 44
Weicheng Hu, Qingshan Yang, Hua-Peng Chen, et al.
Renewable Energy (2021) Vol. 179, pp. 2174-2186
Closed Access | Times Cited: 44
Forecasting Renewable Energy Generation with Machine learning and Deep Learning: Current Advances and Future Prospects
Natei Ermias Benti, Mesfin Diro Chaka, Addisu Gezahegn Semie
(2023)
Open Access | Times Cited: 21
Natei Ermias Benti, Mesfin Diro Chaka, Addisu Gezahegn Semie
(2023)
Open Access | Times Cited: 21
Probabilistic prediction of wind speed using an integrated deep belief network optimized by a hybrid multi-objective particle swarm algorithm
Snigdha Sarangi, P.K. Dash, Ranjeeta Bisoi
Engineering Applications of Artificial Intelligence (2023) Vol. 126, pp. 107034-107034
Closed Access | Times Cited: 21
Snigdha Sarangi, P.K. Dash, Ranjeeta Bisoi
Engineering Applications of Artificial Intelligence (2023) Vol. 126, pp. 107034-107034
Closed Access | Times Cited: 21
Deep learning techniques for solar tracking systems: A systematic literature review, research challenges, and open research directions
Musa Phiri, Mwenge Mulenga, Aaron Zimba, et al.
Solar Energy (2023) Vol. 262, pp. 111803-111803
Closed Access | Times Cited: 17
Musa Phiri, Mwenge Mulenga, Aaron Zimba, et al.
Solar Energy (2023) Vol. 262, pp. 111803-111803
Closed Access | Times Cited: 17
A Deep Convolutional Neural Network Model for Improving WRF Simulations
Alqamah Sayeed, Yunsoo Choi, Jia Jung, et al.
IEEE Transactions on Neural Networks and Learning Systems (2021) Vol. 34, Iss. 2, pp. 750-760
Open Access | Times Cited: 39
Alqamah Sayeed, Yunsoo Choi, Jia Jung, et al.
IEEE Transactions on Neural Networks and Learning Systems (2021) Vol. 34, Iss. 2, pp. 750-760
Open Access | Times Cited: 39
Deep Spatial-Temporal 2-D CNN-BLSTM Model for Ultrashort-Term LiDAR-Assisted Wind Turbine's Power and Fatigue Load Forecasting
Amirhossein Dolatabadi, Hussein Abdeltawab, Yasser Abdel‐Rady I. Mohamed
IEEE Transactions on Industrial Informatics (2021) Vol. 18, Iss. 4, pp. 2342-2353
Closed Access | Times Cited: 37
Amirhossein Dolatabadi, Hussein Abdeltawab, Yasser Abdel‐Rady I. Mohamed
IEEE Transactions on Industrial Informatics (2021) Vol. 18, Iss. 4, pp. 2342-2353
Closed Access | Times Cited: 37