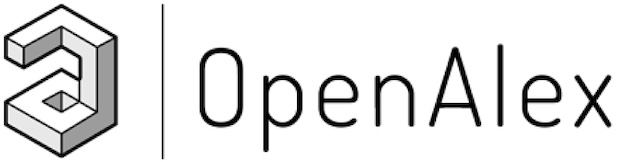
OpenAlex is a bibliographic catalogue of scientific papers, authors and institutions accessible in open access mode, named after the Library of Alexandria. It's citation coverage is excellent and I hope you will find utility in this listing of citing articles!
If you click the article title, you'll navigate to the article, as listed in CrossRef. If you click the Open Access links, you'll navigate to the "best Open Access location". Clicking the citation count will open this listing for that article. Lastly at the bottom of the page, you'll find basic pagination options.
Requested Article:
Concrete compressive strength prediction using an explainable boosting machine model
Gaoyang Liu, Bochao Sun
Case Studies in Construction Materials (2023) Vol. 18, pp. e01845-e01845
Open Access | Times Cited: 36
Gaoyang Liu, Bochao Sun
Case Studies in Construction Materials (2023) Vol. 18, pp. e01845-e01845
Open Access | Times Cited: 36
Showing 1-25 of 36 citing articles:
Hybrid data-driven approaches to predicting the compressive strength of ultra-high-performance concrete using SHAP and PDP analyses
Abul Kashem, Rezaul Karim, Somir Chandra Malo, et al.
Case Studies in Construction Materials (2024) Vol. 20, pp. e02991-e02991
Open Access | Times Cited: 46
Abul Kashem, Rezaul Karim, Somir Chandra Malo, et al.
Case Studies in Construction Materials (2024) Vol. 20, pp. e02991-e02991
Open Access | Times Cited: 46
Compressive strength evaluation of cement-based materials in sulphate environment using optimized deep learning technology
Yang Yu, Chunwei Zhang, Xingyang Xie, et al.
Developments in the Built Environment (2023) Vol. 16, pp. 100298-100298
Open Access | Times Cited: 37
Yang Yu, Chunwei Zhang, Xingyang Xie, et al.
Developments in the Built Environment (2023) Vol. 16, pp. 100298-100298
Open Access | Times Cited: 37
Compressive strength prediction of high-strength concrete using hybrid machine learning approaches by incorporating SHAP analysis
Abul Kashem, Pobithra Das
Asian Journal of Civil Engineering (2023) Vol. 24, Iss. 8, pp. 3243-3263
Closed Access | Times Cited: 36
Abul Kashem, Pobithra Das
Asian Journal of Civil Engineering (2023) Vol. 24, Iss. 8, pp. 3243-3263
Closed Access | Times Cited: 36
A study on the macro-properties of PCB fiber-reinforced concrete from recycled electronic waste and validation of results using RSM and ANN
M. Vishnupriyan, R. Annadurai
Asian Journal of Civil Engineering (2023) Vol. 24, Iss. 6, pp. 1667-1680
Closed Access | Times Cited: 30
M. Vishnupriyan, R. Annadurai
Asian Journal of Civil Engineering (2023) Vol. 24, Iss. 6, pp. 1667-1680
Closed Access | Times Cited: 30
A hybrid strategy of AutoML and SHAP for automated and explainable concrete strength prediction
Bochao Sun, Wenjun Cui, Gaoyang Liu, et al.
Case Studies in Construction Materials (2023) Vol. 19, pp. e02405-e02405
Open Access | Times Cited: 25
Bochao Sun, Wenjun Cui, Gaoyang Liu, et al.
Case Studies in Construction Materials (2023) Vol. 19, pp. e02405-e02405
Open Access | Times Cited: 25
Predicting concrete strength through packing density using machine learning models
Pallapothu Swamy Naga Ratna Giri, Rathish Kumar Pancharathi, Rakesh Janib
Engineering Applications of Artificial Intelligence (2023) Vol. 126, pp. 107177-107177
Closed Access | Times Cited: 25
Pallapothu Swamy Naga Ratna Giri, Rathish Kumar Pancharathi, Rakesh Janib
Engineering Applications of Artificial Intelligence (2023) Vol. 126, pp. 107177-107177
Closed Access | Times Cited: 25
A comparative study of machine learning models for construction costs prediction with natural gradient boosting algorithm and SHAP analysis
Pobithra Das, Abul Kashem, Imrul Hasan, et al.
Asian Journal of Civil Engineering (2024) Vol. 25, Iss. 4, pp. 3301-3316
Closed Access | Times Cited: 13
Pobithra Das, Abul Kashem, Imrul Hasan, et al.
Asian Journal of Civil Engineering (2024) Vol. 25, Iss. 4, pp. 3301-3316
Closed Access | Times Cited: 13
Optimized machine learning models for prediction of effective stiffness of rectangular reinforced concrete column sections
Sanjog Chhetri Sapkota, Sourav Das, Prasenjit Saha
Structures (2024) Vol. 62, pp. 106155-106155
Closed Access | Times Cited: 9
Sanjog Chhetri Sapkota, Sourav Das, Prasenjit Saha
Structures (2024) Vol. 62, pp. 106155-106155
Closed Access | Times Cited: 9
Innovative machine learning approaches to predict the compressive strength of recycled plastic aggregate self-compacting concrete incorporating different waste ashes
Brwa Hamah Saeed Hamah Ali, Rabar H. Faraj, Mariwan Hama Saeed, et al.
Multiscale and Multidisciplinary Modeling Experiments and Design (2024) Vol. 7, Iss. 3, pp. 2585-2604
Closed Access | Times Cited: 6
Brwa Hamah Saeed Hamah Ali, Rabar H. Faraj, Mariwan Hama Saeed, et al.
Multiscale and Multidisciplinary Modeling Experiments and Design (2024) Vol. 7, Iss. 3, pp. 2585-2604
Closed Access | Times Cited: 6
Machine learning-based compressive strength estimation in nanomaterial-modified lightweight concrete
Nashat S. Alghrairi, Farah Nora Aznieta Abdul Aziz, Suraya Abdul Rashid, et al.
Open Engineering (2024) Vol. 14, Iss. 1
Open Access | Times Cited: 6
Nashat S. Alghrairi, Farah Nora Aznieta Abdul Aziz, Suraya Abdul Rashid, et al.
Open Engineering (2024) Vol. 14, Iss. 1
Open Access | Times Cited: 6
Machine learning-enabled characterization of concrete mechanical strength through correlation of flexural and torsional resonance frequencies
Bai Li, Majid Samavatian, Vahid Samavatian
Physica Scripta (2024) Vol. 99, Iss. 7, pp. 076002-076002
Closed Access | Times Cited: 6
Bai Li, Majid Samavatian, Vahid Samavatian
Physica Scripta (2024) Vol. 99, Iss. 7, pp. 076002-076002
Closed Access | Times Cited: 6
Hybrid machine learning model to predict the mechanical properties of ultra-high-performance concrete (UHPC) with experimental validation
Ajad Shrestha, Sanjog Chhetri Sapkota
Asian Journal of Civil Engineering (2024) Vol. 25, Iss. 7, pp. 5227-5244
Closed Access | Times Cited: 5
Ajad Shrestha, Sanjog Chhetri Sapkota
Asian Journal of Civil Engineering (2024) Vol. 25, Iss. 7, pp. 5227-5244
Closed Access | Times Cited: 5
Prediction of compressive strength of high-performance concrete using optimization machine learning approaches with SHAP analysis
Md Mahamodul Islam, Pobithra Das, Md Mahbubur Rahman, et al.
Journal of Building Pathology and Rehabilitation (2024) Vol. 9, Iss. 2
Closed Access | Times Cited: 4
Md Mahamodul Islam, Pobithra Das, Md Mahbubur Rahman, et al.
Journal of Building Pathology and Rehabilitation (2024) Vol. 9, Iss. 2
Closed Access | Times Cited: 4
Explainable AutoML models for predicting the strength of high-performance concrete using Optuna, SHAP and ensemble learning
Muhammad Salman Khan, Tianbo Peng, Muhammad Adeel Khan, et al.
Frontiers in Materials (2025) Vol. 12
Open Access
Muhammad Salman Khan, Tianbo Peng, Muhammad Adeel Khan, et al.
Frontiers in Materials (2025) Vol. 12
Open Access
An explainable boosting-based ensemble machine learning model for predicting the properties of date palm fiber reinforced concrete
Musa Adamu, Sanjog Chhetri Sapkota, Sourav Das, et al.
Sustainable Chemistry and Pharmacy (2025) Vol. 44, pp. 101949-101949
Closed Access
Musa Adamu, Sanjog Chhetri Sapkota, Sourav Das, et al.
Sustainable Chemistry and Pharmacy (2025) Vol. 44, pp. 101949-101949
Closed Access
Intelligent Prediction of Compressive Strength of Concrete Based on CNN-BiLSTM-MA
Yuqiao Liu, Hongling Yu, Tao Guan, et al.
Case Studies in Construction Materials (2025), pp. e04486-e04486
Open Access
Yuqiao Liu, Hongling Yu, Tao Guan, et al.
Case Studies in Construction Materials (2025), pp. e04486-e04486
Open Access
Machine learning applications in designing cementitious materials
Shichen Dang, Hu Fang, Yao Yao
Automation in Construction (2025) Vol. 174, pp. 106125-106125
Closed Access
Shichen Dang, Hu Fang, Yao Yao
Automation in Construction (2025) Vol. 174, pp. 106125-106125
Closed Access
Prediction of gross calorific value from coal analysis using decision tree-based bagging and boosting techniques
Tanveer Alam Munshi, Labiba Nusrat Jahan, M. Farhad Howladar, et al.
Heliyon (2023) Vol. 10, Iss. 1, pp. e23395-e23395
Open Access | Times Cited: 11
Tanveer Alam Munshi, Labiba Nusrat Jahan, M. Farhad Howladar, et al.
Heliyon (2023) Vol. 10, Iss. 1, pp. e23395-e23395
Open Access | Times Cited: 11
Explainable AI Frameworks: Navigating the Present Challenges and Unveiling Innovative Applications
Neeraj Anand Sharma, Rishal Ravikesh Chand, Zain Buksh, et al.
Algorithms (2024) Vol. 17, Iss. 6, pp. 227-227
Open Access | Times Cited: 3
Neeraj Anand Sharma, Rishal Ravikesh Chand, Zain Buksh, et al.
Algorithms (2024) Vol. 17, Iss. 6, pp. 227-227
Open Access | Times Cited: 3
Exploring the interrelationships between composition, rheology, and compressive strength of self-compacting concrete: An exploration of explainable boosting algorithms
Sarmed Wahab, Babatunde Abiodun Salami, Ali H. AlAteah, et al.
Case Studies in Construction Materials (2024) Vol. 20, pp. e03084-e03084
Open Access | Times Cited: 2
Sarmed Wahab, Babatunde Abiodun Salami, Ali H. AlAteah, et al.
Case Studies in Construction Materials (2024) Vol. 20, pp. e03084-e03084
Open Access | Times Cited: 2
Applications of machine learning method in high-performance materials design: a review
Junhao Yuan, Zhen Li, Yujia Yang, et al.
Journal of Materials Informatics (2024) Vol. 4, Iss. 3
Open Access | Times Cited: 2
Junhao Yuan, Zhen Li, Yujia Yang, et al.
Journal of Materials Informatics (2024) Vol. 4, Iss. 3
Open Access | Times Cited: 2
Explainable Boosting Machine for Predicting Wind Shear-Induced Aircraft Go-around based on Pilot Reports
Afaq Khattak, Pak Wai Chan, Feng Chen, et al.
KSCE Journal of Civil Engineering (2023) Vol. 27, Iss. 10, pp. 4115-4129
Open Access | Times Cited: 6
Afaq Khattak, Pak Wai Chan, Feng Chen, et al.
KSCE Journal of Civil Engineering (2023) Vol. 27, Iss. 10, pp. 4115-4129
Open Access | Times Cited: 6
Prediction of frost resistance and multiobjective optimisation of low-carbon concrete on the basis of machine learning
Jinpeng Dai, Zhijie Zhang, Xuwei Dong, et al.
Materials Today Communications (2024) Vol. 40, pp. 109525-109525
Closed Access | Times Cited: 1
Jinpeng Dai, Zhijie Zhang, Xuwei Dong, et al.
Materials Today Communications (2024) Vol. 40, pp. 109525-109525
Closed Access | Times Cited: 1
Prediction of compressive strength of concrete under various curing conditions: a comparison of machine learning models and empirical mathematical models
Bochao Sun, Yuxiang Huang, Gaoyang Liu, et al.
Innovative Infrastructure Solutions (2024) Vol. 9, Iss. 7
Closed Access | Times Cited: 1
Bochao Sun, Yuxiang Huang, Gaoyang Liu, et al.
Innovative Infrastructure Solutions (2024) Vol. 9, Iss. 7
Closed Access | Times Cited: 1
Interpretable and explainable predictive machine learning models for data-driven protein engineering
David Medina-Ortiz, Ashkan Khalifeh, Hoda Anvari-Kazemabad, et al.
Biotechnology Advances (2024) Vol. 79, pp. 108495-108495
Open Access | Times Cited: 1
David Medina-Ortiz, Ashkan Khalifeh, Hoda Anvari-Kazemabad, et al.
Biotechnology Advances (2024) Vol. 79, pp. 108495-108495
Open Access | Times Cited: 1