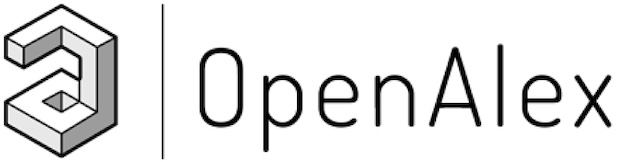
OpenAlex is a bibliographic catalogue of scientific papers, authors and institutions accessible in open access mode, named after the Library of Alexandria. It's citation coverage is excellent and I hope you will find utility in this listing of citing articles!
If you click the article title, you'll navigate to the article, as listed in CrossRef. If you click the Open Access links, you'll navigate to the "best Open Access location". Clicking the citation count will open this listing for that article. Lastly at the bottom of the page, you'll find basic pagination options.
Requested Article:
Prediction of seven-day compressive strength of field concrete
Xueqing Zhang, Muhammad Zeshan Akber, Wei Zheng
Construction and Building Materials (2021) Vol. 305, pp. 124604-124604
Closed Access | Times Cited: 67
Xueqing Zhang, Muhammad Zeshan Akber, Wei Zheng
Construction and Building Materials (2021) Vol. 305, pp. 124604-124604
Closed Access | Times Cited: 67
Showing 1-25 of 67 citing articles:
Hybrid machine learning model and Shapley additive explanations for compressive strength of sustainable concrete
Yanqi Wu, Yisong Zhou
Construction and Building Materials (2022) Vol. 330, pp. 127298-127298
Closed Access | Times Cited: 134
Yanqi Wu, Yisong Zhou
Construction and Building Materials (2022) Vol. 330, pp. 127298-127298
Closed Access | Times Cited: 134
Compressive Strength Prediction of Lightweight Concrete: Machine Learning Models
Aman Kumar, Harish Chandra Arora, Nishant Raj Kapoor, et al.
Sustainability (2022) Vol. 14, Iss. 4, pp. 2404-2404
Open Access | Times Cited: 89
Aman Kumar, Harish Chandra Arora, Nishant Raj Kapoor, et al.
Sustainability (2022) Vol. 14, Iss. 4, pp. 2404-2404
Open Access | Times Cited: 89
Machine learning interpretable-prediction models to evaluate the slump and strength of fly ash-based geopolymer
Sohaib Nazar, Jian Yang, Muhammad Nasir Amin, et al.
Journal of Materials Research and Technology (2023) Vol. 24, pp. 100-124
Open Access | Times Cited: 66
Sohaib Nazar, Jian Yang, Muhammad Nasir Amin, et al.
Journal of Materials Research and Technology (2023) Vol. 24, pp. 100-124
Open Access | Times Cited: 66
Prediction and optimization model of sustainable concrete properties using machine learning, deep learning and swarm intelligence: A review
Shiqi Wang, Peng Xia, Keyu Chen, et al.
Journal of Building Engineering (2023) Vol. 80, pp. 108065-108065
Closed Access | Times Cited: 60
Shiqi Wang, Peng Xia, Keyu Chen, et al.
Journal of Building Engineering (2023) Vol. 80, pp. 108065-108065
Closed Access | Times Cited: 60
Artificial intelligence algorithms for prediction and sensitivity analysis of mechanical properties of recycled aggregate concrete: A review
Tien-Dung Nguyen, Rachid Cherif, Pierre-Yves Mahieux, et al.
Journal of Building Engineering (2023) Vol. 66, pp. 105929-105929
Open Access | Times Cited: 56
Tien-Dung Nguyen, Rachid Cherif, Pierre-Yves Mahieux, et al.
Journal of Building Engineering (2023) Vol. 66, pp. 105929-105929
Open Access | Times Cited: 56
Data-driven multicollinearity-aware multi-objective optimisation of green concrete mixes
Elyas Asadi Shamsabadi, Masoud Salehpour, Peyman Zandifaez, et al.
Journal of Cleaner Production (2023) Vol. 390, pp. 136103-136103
Open Access | Times Cited: 44
Elyas Asadi Shamsabadi, Masoud Salehpour, Peyman Zandifaez, et al.
Journal of Cleaner Production (2023) Vol. 390, pp. 136103-136103
Open Access | Times Cited: 44
Leveraging an integrated multivariate analytical approach towards strength enhancement of fly ash-based concrete
Vikrant S. Vairagade, Shrikrishna A. Dhale, Kavita V. Joshi, et al.
Multiscale and Multidisciplinary Modeling Experiments and Design (2025) Vol. 8, Iss. 1
Closed Access | Times Cited: 2
Vikrant S. Vairagade, Shrikrishna A. Dhale, Kavita V. Joshi, et al.
Multiscale and Multidisciplinary Modeling Experiments and Design (2025) Vol. 8, Iss. 1
Closed Access | Times Cited: 2
Development of machine learning models for the prediction of the compressive strength of calcium-based geopolymers
Wangwen Huo, Zhiduo Zhu, He Sun, et al.
Journal of Cleaner Production (2022) Vol. 380, pp. 135159-135159
Closed Access | Times Cited: 65
Wangwen Huo, Zhiduo Zhu, He Sun, et al.
Journal of Cleaner Production (2022) Vol. 380, pp. 135159-135159
Closed Access | Times Cited: 65
A Comparison of Machine Learning Tools That Model the Splitting Tensile Strength of Self-Compacting Recycled Aggregate Concrete
Jesús de‐Prado‐Gil, Covadonga Palencia, P. Jagadesh, et al.
Materials (2022) Vol. 15, Iss. 12, pp. 4164-4164
Open Access | Times Cited: 43
Jesús de‐Prado‐Gil, Covadonga Palencia, P. Jagadesh, et al.
Materials (2022) Vol. 15, Iss. 12, pp. 4164-4164
Open Access | Times Cited: 43
Predicting the slump of industrially produced concrete using machine learning: A multiclass classification approach
Xueqing Zhang, Muhammad Zeshan Akber, Wei Zheng
Journal of Building Engineering (2022) Vol. 58, pp. 104997-104997
Closed Access | Times Cited: 39
Xueqing Zhang, Muhammad Zeshan Akber, Wei Zheng
Journal of Building Engineering (2022) Vol. 58, pp. 104997-104997
Closed Access | Times Cited: 39
Estimation of rubberized concrete frost resistance using machine learning techniques
Xifeng Gao, Jian Yang, Han Zhu, et al.
Construction and Building Materials (2023) Vol. 371, pp. 130778-130778
Closed Access | Times Cited: 34
Xifeng Gao, Jian Yang, Han Zhu, et al.
Construction and Building Materials (2023) Vol. 371, pp. 130778-130778
Closed Access | Times Cited: 34
Fractionation of dyes/salts using loose nanofiltration membranes: Insight from machine learning prediction
Nadeem Baig, Jamilu Usman, Sani I. Abba, et al.
Journal of Cleaner Production (2023) Vol. 418, pp. 138193-138193
Closed Access | Times Cited: 33
Nadeem Baig, Jamilu Usman, Sani I. Abba, et al.
Journal of Cleaner Production (2023) Vol. 418, pp. 138193-138193
Closed Access | Times Cited: 33
A comparative study of machine learning algorithms for the prediction of compressive strength of rice husk ash-based concrete
Akshita Bassi, Aditya Manchanda, Rajwinder Singh, et al.
Natural Hazards (2023) Vol. 118, Iss. 1, pp. 209-238
Closed Access | Times Cited: 23
Akshita Bassi, Aditya Manchanda, Rajwinder Singh, et al.
Natural Hazards (2023) Vol. 118, Iss. 1, pp. 209-238
Closed Access | Times Cited: 23
Predictive models in machine learning for strength and life cycle assessment of concrete structures
A. Dinesh, B. Rahul Prasad
Automation in Construction (2024) Vol. 162, pp. 105412-105412
Closed Access | Times Cited: 15
A. Dinesh, B. Rahul Prasad
Automation in Construction (2024) Vol. 162, pp. 105412-105412
Closed Access | Times Cited: 15
Prediction of compressive strength and tensile strain of engineered cementitious composite using machine learning
Md Nasir Uddin, N. Shanmugasundaram, S. Praveenkumar, et al.
International Journal of Mechanics and Materials in Design (2024) Vol. 20, Iss. 4, pp. 671-716
Closed Access | Times Cited: 13
Md Nasir Uddin, N. Shanmugasundaram, S. Praveenkumar, et al.
International Journal of Mechanics and Materials in Design (2024) Vol. 20, Iss. 4, pp. 671-716
Closed Access | Times Cited: 13
Prediction of properties of recycled aggregate concrete using machine learning models: A critical review
Zengfeng Zhao, Yajie Liu, Yanyun Lu, et al.
Journal of Building Engineering (2024) Vol. 90, pp. 109516-109516
Closed Access | Times Cited: 13
Zengfeng Zhao, Yajie Liu, Yanyun Lu, et al.
Journal of Building Engineering (2024) Vol. 90, pp. 109516-109516
Closed Access | Times Cited: 13
Bayesian design of concrete with amortized Gaussian processes and multi-objective optimization
Olivia Pfeiffer, Kai Gong, Kristen Severson, et al.
Cement and Concrete Research (2024) Vol. 177, pp. 107406-107406
Closed Access | Times Cited: 9
Olivia Pfeiffer, Kai Gong, Kristen Severson, et al.
Cement and Concrete Research (2024) Vol. 177, pp. 107406-107406
Closed Access | Times Cited: 9
A systematic literature review of AI-based prediction methods for self-compacting, geopolymer, and other eco-friendly concrete types: Advancing sustainable concrete
Tariq Ali, Mohamed Hechmi El Ouni, Muhammad Zeeshan Qureshi, et al.
Construction and Building Materials (2024) Vol. 440, pp. 137370-137370
Closed Access | Times Cited: 8
Tariq Ali, Mohamed Hechmi El Ouni, Muhammad Zeeshan Qureshi, et al.
Construction and Building Materials (2024) Vol. 440, pp. 137370-137370
Closed Access | Times Cited: 8
Mechanical properties prediction of sandstone concrete with varying compaction levels and silica fume ratios using machine-learning approaches
Amr Ghoniem, Louay A. Aboul-Nour
Construction and Building Materials (2025) Vol. 460, pp. 139817-139817
Closed Access | Times Cited: 1
Amr Ghoniem, Louay A. Aboul-Nour
Construction and Building Materials (2025) Vol. 460, pp. 139817-139817
Closed Access | Times Cited: 1
Implementation of nonlinear computing models and classical regression for predicting compressive strength of high-performance concrete
Mahmud M. Jibril, M.A Zayyan, Salim Idris Malami, et al.
Applications in Engineering Science (2023) Vol. 15, pp. 100133-100133
Open Access | Times Cited: 17
Mahmud M. Jibril, M.A Zayyan, Salim Idris Malami, et al.
Applications in Engineering Science (2023) Vol. 15, pp. 100133-100133
Open Access | Times Cited: 17
Accurate compressive strength prediction using machine learning algorithms and optimization techniques
Wenbin Lan
Journal of Engineering and Applied Science (2024) Vol. 71, Iss. 1
Open Access | Times Cited: 6
Wenbin Lan
Journal of Engineering and Applied Science (2024) Vol. 71, Iss. 1
Open Access | Times Cited: 6
Hybridizing Grid Search and Support Vector Regression to Predict the Compressive Strength of Fly Ash Concrete
Fei Tang, Yanqi Wu, Yisong Zhou
Advances in Civil Engineering (2022) Vol. 2022, Iss. 1
Open Access | Times Cited: 27
Fei Tang, Yanqi Wu, Yisong Zhou
Advances in Civil Engineering (2022) Vol. 2022, Iss. 1
Open Access | Times Cited: 27
Prediction and uncertainty quantification of compressive strength of high‐strength concrete using optimized machine learning algorithms
Bing Han, Yanqi Wu, Lulu Liu
Structural Concrete (2022) Vol. 23, Iss. 6, pp. 3772-3785
Closed Access | Times Cited: 26
Bing Han, Yanqi Wu, Lulu Liu
Structural Concrete (2022) Vol. 23, Iss. 6, pp. 3772-3785
Closed Access | Times Cited: 26
A framework for predicting the carbonation depth of concrete incorporating fly ash based on a least squares support vector machine and metaheuristic algorithms
Kai Zhang, Ke Zhang, Rui Bao, et al.
Journal of Building Engineering (2022) Vol. 65, pp. 105772-105772
Closed Access | Times Cited: 24
Kai Zhang, Ke Zhang, Rui Bao, et al.
Journal of Building Engineering (2022) Vol. 65, pp. 105772-105772
Closed Access | Times Cited: 24
Performance evaluation of slag-based concrete at elevated temperatures by a novel machine learning approach
Vahab Toufigh, Soheil Palizi
Construction and Building Materials (2022) Vol. 358, pp. 129357-129357
Closed Access | Times Cited: 23
Vahab Toufigh, Soheil Palizi
Construction and Building Materials (2022) Vol. 358, pp. 129357-129357
Closed Access | Times Cited: 23