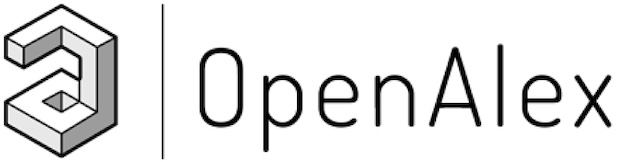
OpenAlex is a bibliographic catalogue of scientific papers, authors and institutions accessible in open access mode, named after the Library of Alexandria. It's citation coverage is excellent and I hope you will find utility in this listing of citing articles!
If you click the article title, you'll navigate to the article, as listed in CrossRef. If you click the Open Access links, you'll navigate to the "best Open Access location". Clicking the citation count will open this listing for that article. Lastly at the bottom of the page, you'll find basic pagination options.
Requested Article:
Physics-guided neural networks with engineering domain knowledge for hybrid process modeling
Ethan R. Gallup, Tyler Gallup, Kody M. Powell
Computers & Chemical Engineering (2023) Vol. 170, pp. 108111-108111
Open Access | Times Cited: 13
Ethan R. Gallup, Tyler Gallup, Kody M. Powell
Computers & Chemical Engineering (2023) Vol. 170, pp. 108111-108111
Open Access | Times Cited: 13
Showing 13 citing articles:
Inverse machine learning framework for optimizing gradient honeycomb structure under impact loading
Xingyu Shen, Ke Yan, Difeng Zhu, et al.
Engineering Structures (2024) Vol. 309, pp. 118079-118079
Closed Access | Times Cited: 8
Xingyu Shen, Ke Yan, Difeng Zhu, et al.
Engineering Structures (2024) Vol. 309, pp. 118079-118079
Closed Access | Times Cited: 8
Law of conservation-guided neural network with gradient aggregation for improved energy efficiency optimization in industrial processes
Santi Bardeeniz, Chanin Panjapornpon, Moonyong Lee
Energy and AI (2025), pp. 100475-100475
Open Access
Santi Bardeeniz, Chanin Panjapornpon, Moonyong Lee
Energy and AI (2025), pp. 100475-100475
Open Access
Physics-informed machine learning algorithms for forecasting sediment yield: an analysis of physical consistency, sensitivity, and interpretability
Ali El Bilali, Youssef Brouziyne, Oumaima Attar, et al.
Environmental Science and Pollution Research (2024) Vol. 31, Iss. 34, pp. 47237-47257
Closed Access | Times Cited: 3
Ali El Bilali, Youssef Brouziyne, Oumaima Attar, et al.
Environmental Science and Pollution Research (2024) Vol. 31, Iss. 34, pp. 47237-47257
Closed Access | Times Cited: 3
Artificial intelligence and machine learning at various stages and scales of process systems engineering
Karthik K. Srinivasan, Anjana Puliyanda, Devavrat Thosar, et al.
The Canadian Journal of Chemical Engineering (2024) Vol. 103, Iss. 3, pp. 1004-1035
Open Access | Times Cited: 2
Karthik K. Srinivasan, Anjana Puliyanda, Devavrat Thosar, et al.
The Canadian Journal of Chemical Engineering (2024) Vol. 103, Iss. 3, pp. 1004-1035
Open Access | Times Cited: 2
The Application of GCN Algorithm in Building Construction Knowledge Graph Updating under the Combination of Artificial Intelligence and Knowledge Management
Lü He, Hu Xu
International Journal of Cognitive Computing in Engineering (2024)
Open Access | Times Cited: 1
Lü He, Hu Xu
International Journal of Cognitive Computing in Engineering (2024)
Open Access | Times Cited: 1
Neural network models for atmospheric residue desulfurization using real plant data with novel training strategies
Yungun Jung, Hyungjun Kim, Gyeonggwan Jeon, et al.
Computers & Chemical Engineering (2023) Vol. 177, pp. 108333-108333
Closed Access | Times Cited: 3
Yungun Jung, Hyungjun Kim, Gyeonggwan Jeon, et al.
Computers & Chemical Engineering (2023) Vol. 177, pp. 108333-108333
Closed Access | Times Cited: 3
Chemical process modelling using the extracted informative data sets based on attenuating excitation inputs
Likun Yuan, Baochang Xu, Zhi‐Shan Liang, et al.
Journal of the Taiwan Institute of Chemical Engineers (2023) Vol. 146, pp. 104872-104872
Closed Access | Times Cited: 2
Likun Yuan, Baochang Xu, Zhi‐Shan Liang, et al.
Journal of the Taiwan Institute of Chemical Engineers (2023) Vol. 146, pp. 104872-104872
Closed Access | Times Cited: 2
Improving Deep Learning on Hyperspectral Images of Grain by Incorporating Domain Knowledge from Chemometrics
Ole‐Christian Galbo Engstrøm, Erik Schou Dreier, Birthe Møller Jespersen, et al.
(2023), pp. 485-494
Closed Access | Times Cited: 2
Ole‐Christian Galbo Engstrøm, Erik Schou Dreier, Birthe Møller Jespersen, et al.
(2023), pp. 485-494
Closed Access | Times Cited: 2
A novel Transformer-based model with large kernel temporal convolution for chemical process fault detection
Zhichao Zhu, Feiyang Chen, Lei Ni, et al.
Computers & Chemical Engineering (2024) Vol. 188, pp. 108762-108762
Closed Access
Zhichao Zhu, Feiyang Chen, Lei Ni, et al.
Computers & Chemical Engineering (2024) Vol. 188, pp. 108762-108762
Closed Access
A physics guided data-driven prediction method for dynamic and static feature fusion modeling of rolling force in steel strip production
Song Yong, Wendan Xiao, Fenjia Wang, et al.
Control Engineering Practice (2024) Vol. 151, pp. 106039-106039
Closed Access
Song Yong, Wendan Xiao, Fenjia Wang, et al.
Control Engineering Practice (2024) Vol. 151, pp. 106039-106039
Closed Access
A physics‐constrained hybrid residual neural network for the prediction of moisture content in a closed‐cycle drying system
Mengfei Zhou, Ruizhen Wang, Cheng Rong, et al.
The Canadian Journal of Chemical Engineering (2024)
Closed Access
Mengfei Zhou, Ruizhen Wang, Cheng Rong, et al.
The Canadian Journal of Chemical Engineering (2024)
Closed Access
Development of physics-guided neural network framework for acid-base treatment prediction using carbon dioxide-based tubular reactor
Chanin Panjapornpon, Patcharapol Chinchalongporn, Santi Bardeeniz, et al.
Engineering Applications of Artificial Intelligence (2024) Vol. 138, pp. 109500-109500
Closed Access
Chanin Panjapornpon, Patcharapol Chinchalongporn, Santi Bardeeniz, et al.
Engineering Applications of Artificial Intelligence (2024) Vol. 138, pp. 109500-109500
Closed Access
Does Kaizen Programming need a physic-informed mechanism to improve the search?
Jimena Ferreira, Ana I. Torres, Martín Pedemonte
(2023), pp. 1-6
Closed Access
Jimena Ferreira, Ana I. Torres, Martín Pedemonte
(2023), pp. 1-6
Closed Access