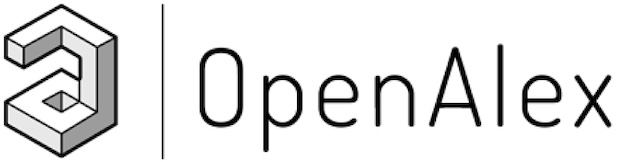
OpenAlex is a bibliographic catalogue of scientific papers, authors and institutions accessible in open access mode, named after the Library of Alexandria. It's citation coverage is excellent and I hope you will find utility in this listing of citing articles!
If you click the article title, you'll navigate to the article, as listed in CrossRef. If you click the Open Access links, you'll navigate to the "best Open Access location". Clicking the citation count will open this listing for that article. Lastly at the bottom of the page, you'll find basic pagination options.
Requested Article:
Sleep stage classification based on multi-level feature learning and recurrent neural networks via wearable device
Xin Zhang, Weixuan Kou, Eric Chang, et al.
Computers in Biology and Medicine (2018) Vol. 103, pp. 71-81
Open Access | Times Cited: 70
Xin Zhang, Weixuan Kou, Eric Chang, et al.
Computers in Biology and Medicine (2018) Vol. 103, pp. 71-81
Open Access | Times Cited: 70
Showing 1-25 of 70 citing articles:
The future of sleep health: a data-driven revolution in sleep science and medicine
Ignacio Perez-Pozuelo, Bing Zhai, João Palotti, et al.
npj Digital Medicine (2020) Vol. 3, Iss. 1
Open Access | Times Cited: 223
Ignacio Perez-Pozuelo, Bing Zhai, João Palotti, et al.
npj Digital Medicine (2020) Vol. 3, Iss. 1
Open Access | Times Cited: 223
A Systematic Review of Sensing Technologies for Wearable Sleep Staging
Syed Anas Imtiaz
Sensors (2021) Vol. 21, Iss. 5, pp. 1562-1562
Open Access | Times Cited: 125
Syed Anas Imtiaz
Sensors (2021) Vol. 21, Iss. 5, pp. 1562-1562
Open Access | Times Cited: 125
State of the science and recommendations for using wearable technology in sleep and circadian research
Massimiliano de Zambotti, Cathy Goldstein, Jesse D. Cook, et al.
SLEEP (2023) Vol. 47, Iss. 4
Closed Access | Times Cited: 51
Massimiliano de Zambotti, Cathy Goldstein, Jesse D. Cook, et al.
SLEEP (2023) Vol. 47, Iss. 4
Closed Access | Times Cited: 51
Intelligent Wearable Systems: Opportunities and Challenges in Health and Sports
Luyao Yang, Osama Amin, Basem Shihada
ACM Computing Surveys (2024) Vol. 56, Iss. 7, pp. 1-42
Closed Access | Times Cited: 19
Luyao Yang, Osama Amin, Basem Shihada
ACM Computing Surveys (2024) Vol. 56, Iss. 7, pp. 1-42
Closed Access | Times Cited: 19
Sleep staging from electrocardiography and respiration with deep learning
Haoqi Sun, Wolfgang Ganglberger, Ezhil Panneerselvam, et al.
SLEEP (2019) Vol. 43, Iss. 7
Open Access | Times Cited: 89
Haoqi Sun, Wolfgang Ganglberger, Ezhil Panneerselvam, et al.
SLEEP (2019) Vol. 43, Iss. 7
Open Access | Times Cited: 89
A deep transfer learning approach for wearable sleep stage classification with photoplethysmography
Mustafa Radha, Pedro Fonseca, Arnaud Moreau, et al.
npj Digital Medicine (2021) Vol. 4, Iss. 1
Open Access | Times Cited: 85
Mustafa Radha, Pedro Fonseca, Arnaud Moreau, et al.
npj Digital Medicine (2021) Vol. 4, Iss. 1
Open Access | Times Cited: 85
EOGNET: A Novel Deep Learning Model for Sleep Stage Classification Based on Single-Channel EOG Signal
Jiahao Fan, Chenglu Sun, Long Meng, et al.
Frontiers in Neuroscience (2021) Vol. 15
Open Access | Times Cited: 56
Jiahao Fan, Chenglu Sun, Long Meng, et al.
Frontiers in Neuroscience (2021) Vol. 15
Open Access | Times Cited: 56
SleepFCN: A Fully Convolutional Deep Learning Framework for Sleep Stage Classification Using Single-Channel Electroencephalograms
Narjes Goshtasbi, Reza Boostani, Saeid Sanei
IEEE Transactions on Neural Systems and Rehabilitation Engineering (2022) Vol. 30, pp. 2088-2096
Open Access | Times Cited: 48
Narjes Goshtasbi, Reza Boostani, Saeid Sanei
IEEE Transactions on Neural Systems and Rehabilitation Engineering (2022) Vol. 30, pp. 2088-2096
Open Access | Times Cited: 48
Machine Learning and AI Technologies for Smart Wearables
Kah Phooi Seng, Li-Minn Ang, Eno Peter, et al.
Electronics (2023) Vol. 12, Iss. 7, pp. 1509-1509
Open Access | Times Cited: 39
Kah Phooi Seng, Li-Minn Ang, Eno Peter, et al.
Electronics (2023) Vol. 12, Iss. 7, pp. 1509-1509
Open Access | Times Cited: 39
Research and application of deep learning-based sleep staging: Data, modeling, validation, and clinical practice
Huijun Yue, Zhuqi Chen, Wenbin Guo, et al.
Sleep Medicine Reviews (2024) Vol. 74, pp. 101897-101897
Open Access | Times Cited: 13
Huijun Yue, Zhuqi Chen, Wenbin Guo, et al.
Sleep Medicine Reviews (2024) Vol. 74, pp. 101897-101897
Open Access | Times Cited: 13
Non-invasive Techniques for Monitoring Different Aspects of Sleep: A Comprehensive Review
Zawar Hussain, Quan Z. Sheng, Wei Emma Zhang, et al.
ACM Transactions on Computing for Healthcare (2022) Vol. 3, Iss. 2, pp. 1-26
Open Access | Times Cited: 29
Zawar Hussain, Quan Z. Sheng, Wei Emma Zhang, et al.
ACM Transactions on Computing for Healthcare (2022) Vol. 3, Iss. 2, pp. 1-26
Open Access | Times Cited: 29
Current status and prospects of automatic sleep stages scoring: Review
Maksym Gaiduk, Ángel Serrano Alarcón, Ralf Seepold, et al.
Biomedical Engineering Letters (2023) Vol. 13, Iss. 3, pp. 247-272
Open Access | Times Cited: 19
Maksym Gaiduk, Ángel Serrano Alarcón, Ralf Seepold, et al.
Biomedical Engineering Letters (2023) Vol. 13, Iss. 3, pp. 247-272
Open Access | Times Cited: 19
Machine Learning for Reliable Network Attack Detection in SCADA Systems
Rocio Lopez Perez, Florian Adamsky, Ridha Soua, et al.
(2018)
Open Access | Times Cited: 52
Rocio Lopez Perez, Florian Adamsky, Ridha Soua, et al.
(2018)
Open Access | Times Cited: 52
Comparative analysis of different characteristics of automatic sleep stages
Dechun Zhao, Yi Wang, Qiangqiang Wang, et al.
Computer Methods and Programs in Biomedicine (2019) Vol. 175, pp. 53-72
Closed Access | Times Cited: 47
Dechun Zhao, Yi Wang, Qiangqiang Wang, et al.
Computer Methods and Programs in Biomedicine (2019) Vol. 175, pp. 53-72
Closed Access | Times Cited: 47
Computational Sleep Behavior Analysis: A Survey
Sarah Fallmann, Liming Chen
IEEE Access (2019) Vol. 7, pp. 142421-142440
Open Access | Times Cited: 47
Sarah Fallmann, Liming Chen
IEEE Access (2019) Vol. 7, pp. 142421-142440
Open Access | Times Cited: 47
CCRRSleepNet: A Hybrid Relational Inductive Biases Network for Automatic Sleep Stage Classification on Raw Single-Channel EEG
Wenpeng Neng, Jun Lu, Lei Xu
Brain Sciences (2021) Vol. 11, Iss. 4, pp. 456-456
Open Access | Times Cited: 37
Wenpeng Neng, Jun Lu, Lei Xu
Brain Sciences (2021) Vol. 11, Iss. 4, pp. 456-456
Open Access | Times Cited: 37
Quality of Sleep Data Validation From the Xiaomi Mi Band 5 Against Polysomnography: Comparison Study
Patricia Concheiro-Moscoso, Betania Groba, Diego Álvarez-Estévez, et al.
Journal of Medical Internet Research (2023) Vol. 25, pp. e42073-e42073
Open Access | Times Cited: 14
Patricia Concheiro-Moscoso, Betania Groba, Diego Álvarez-Estévez, et al.
Journal of Medical Internet Research (2023) Vol. 25, pp. e42073-e42073
Open Access | Times Cited: 14
World Sleep Society Recommendations for the Use of Wearable Consumer Health Trackers That Monitor Sleep
Michael W.L. Chee, Mathias Baumert, Hannah Scott, et al.
Sleep Medicine (2025), pp. 106506-106506
Closed Access
Michael W.L. Chee, Mathias Baumert, Hannah Scott, et al.
Sleep Medicine (2025), pp. 106506-106506
Closed Access
SleepOMICS: How Big Data Can Revolutionize Sleep Science
Nicola Luigi Bragazzi, Ottavia Guglielmi, Sergio Garbarino
International Journal of Environmental Research and Public Health (2019) Vol. 16, Iss. 2, pp. 291-291
Open Access | Times Cited: 39
Nicola Luigi Bragazzi, Ottavia Guglielmi, Sergio Garbarino
International Journal of Environmental Research and Public Health (2019) Vol. 16, Iss. 2, pp. 291-291
Open Access | Times Cited: 39
Sleep quality prediction in caregivers using physiological signals
Reza Sadeghi, Tanvi Banerjee, Jennifer Hughes, et al.
Computers in Biology and Medicine (2019) Vol. 110, pp. 276-288
Open Access | Times Cited: 39
Reza Sadeghi, Tanvi Banerjee, Jennifer Hughes, et al.
Computers in Biology and Medicine (2019) Vol. 110, pp. 276-288
Open Access | Times Cited: 39
Neonatal EEG sleep stage classification based on deep learning and HMM
Hojat Ghimatgar, Kamran Kazemi, Mohammad Sadegh Helfroush, et al.
Journal of Neural Engineering (2020) Vol. 17, Iss. 3, pp. 036031-036031
Closed Access | Times Cited: 33
Hojat Ghimatgar, Kamran Kazemi, Mohammad Sadegh Helfroush, et al.
Journal of Neural Engineering (2020) Vol. 17, Iss. 3, pp. 036031-036031
Closed Access | Times Cited: 33
Application of artificial intelligence in active assisted living for aging population in real-world setting with commercial devices – A scoping review
Kang Wang, Moojan Ghafurian, Dmytro Chumachenko, et al.
Computers in Biology and Medicine (2024) Vol. 173, pp. 108340-108340
Open Access | Times Cited: 4
Kang Wang, Moojan Ghafurian, Dmytro Chumachenko, et al.
Computers in Biology and Medicine (2024) Vol. 173, pp. 108340-108340
Open Access | Times Cited: 4
Sleep Stage Classification Using Bidirectional LSTM in Wearable Multi-sensor Systems
Yuezhou Zhang, Zhicheng Yang, Ke Lan, et al.
IEEE INFOCOM 2022 - IEEE Conference on Computer Communications Workshops (INFOCOM WKSHPS) (2019)
Open Access | Times Cited: 33
Yuezhou Zhang, Zhicheng Yang, Ke Lan, et al.
IEEE INFOCOM 2022 - IEEE Conference on Computer Communications Workshops (INFOCOM WKSHPS) (2019)
Open Access | Times Cited: 33
Deep-ACTINet: End-to-End Deep Learning Architecture for Automatic Sleep-Wake Detection Using Wrist Actigraphy
Taeheum Cho, Unang Sunarya, Minsoo Yeo, et al.
Electronics (2019) Vol. 8, Iss. 12, pp. 1461-1461
Open Access | Times Cited: 30
Taeheum Cho, Unang Sunarya, Minsoo Yeo, et al.
Electronics (2019) Vol. 8, Iss. 12, pp. 1461-1461
Open Access | Times Cited: 30
Automatic sleep scoring: A deep learning architecture for multi-modality time series
Rui Yan, Fan Li, Dongdong Zhou, et al.
Journal of Neuroscience Methods (2020) Vol. 348, pp. 108971-108971
Open Access | Times Cited: 30
Rui Yan, Fan Li, Dongdong Zhou, et al.
Journal of Neuroscience Methods (2020) Vol. 348, pp. 108971-108971
Open Access | Times Cited: 30