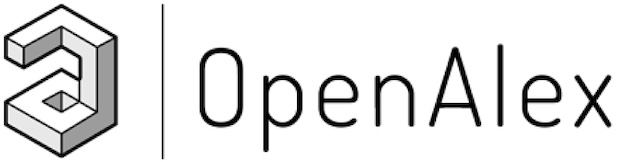
OpenAlex is a bibliographic catalogue of scientific papers, authors and institutions accessible in open access mode, named after the Library of Alexandria. It's citation coverage is excellent and I hope you will find utility in this listing of citing articles!
If you click the article title, you'll navigate to the article, as listed in CrossRef. If you click the Open Access links, you'll navigate to the "best Open Access location". Clicking the citation count will open this listing for that article. Lastly at the bottom of the page, you'll find basic pagination options.
Requested Article:
PhyCRNet: Physics-informed convolutional-recurrent network for solving spatiotemporal PDEs
Pu Ren, Chengping Rao, Yang Liu, et al.
Computer Methods in Applied Mechanics and Engineering (2021) Vol. 389, pp. 114399-114399
Open Access | Times Cited: 149
Pu Ren, Chengping Rao, Yang Liu, et al.
Computer Methods in Applied Mechanics and Engineering (2021) Vol. 389, pp. 114399-114399
Open Access | Times Cited: 149
Showing 1-25 of 149 citing articles:
CAN-PINN: A fast physics-informed neural network based on coupled-automatic–numerical differentiation method
Pao‐Hsiung Chiu, Jian Cheng Wong, Chin Chun Ooi, et al.
Computer Methods in Applied Mechanics and Engineering (2022) Vol. 395, pp. 114909-114909
Open Access | Times Cited: 161
Pao‐Hsiung Chiu, Jian Cheng Wong, Chin Chun Ooi, et al.
Computer Methods in Applied Mechanics and Engineering (2022) Vol. 395, pp. 114909-114909
Open Access | Times Cited: 161
A Review of Physics-Informed Machine Learning in Fluid Mechanics
Pushan Sharma, Wai Tong Chung, Bassem Akoush, et al.
Energies (2023) Vol. 16, Iss. 5, pp. 2343-2343
Open Access | Times Cited: 87
Pushan Sharma, Wai Tong Chung, Bassem Akoush, et al.
Energies (2023) Vol. 16, Iss. 5, pp. 2343-2343
Open Access | Times Cited: 87
Physics-informed PointNet: A deep learning solver for steady-state incompressible flows and thermal fields on multiple sets of irregular geometries
Ali Kashefi, Tapan Mukerji
Journal of Computational Physics (2022) Vol. 468, pp. 111510-111510
Open Access | Times Cited: 80
Ali Kashefi, Tapan Mukerji
Journal of Computational Physics (2022) Vol. 468, pp. 111510-111510
Open Access | Times Cited: 80
Encoding physics to learn reaction–diffusion processes
Chengping Rao, Pu Ren, Qi Wang, et al.
Nature Machine Intelligence (2023) Vol. 5, Iss. 7, pp. 765-779
Closed Access | Times Cited: 52
Chengping Rao, Pu Ren, Qi Wang, et al.
Nature Machine Intelligence (2023) Vol. 5, Iss. 7, pp. 765-779
Closed Access | Times Cited: 52
Physics-Guided, Physics-Informed, and Physics-Encoded Neural Networks and Operators in Scientific Computing: Fluid and Solid Mechanics
Salah A. Faroughi, Nikhil M. Pawar, Célio Fernandes, et al.
Journal of Computing and Information Science in Engineering (2024) Vol. 24, Iss. 4
Closed Access | Times Cited: 45
Salah A. Faroughi, Nikhil M. Pawar, Célio Fernandes, et al.
Journal of Computing and Information Science in Engineering (2024) Vol. 24, Iss. 4
Closed Access | Times Cited: 45
Deep learning in computational mechanics: a review
Leon Herrmann, Stefan Kollmannsberger
Computational Mechanics (2024) Vol. 74, Iss. 2, pp. 281-331
Open Access | Times Cited: 28
Leon Herrmann, Stefan Kollmannsberger
Computational Mechanics (2024) Vol. 74, Iss. 2, pp. 281-331
Open Access | Times Cited: 28
Physics-informed Neural Networks (PINN) for computational solid mechanics: Numerical frameworks and applications
Haoteng Hu, Lehua Qi, Xujiang Chao
Thin-Walled Structures (2024) Vol. 205, pp. 112495-112495
Closed Access | Times Cited: 26
Haoteng Hu, Lehua Qi, Xujiang Chao
Thin-Walled Structures (2024) Vol. 205, pp. 112495-112495
Closed Access | Times Cited: 26
Multi-resolution partial differential equations preserved learning framework for spatiotemporal dynamics
Xin‐Yang Liu, Min Zhu, Lu Lu, et al.
Communications Physics (2024) Vol. 7, Iss. 1
Open Access | Times Cited: 19
Xin‐Yang Liu, Min Zhu, Lu Lu, et al.
Communications Physics (2024) Vol. 7, Iss. 1
Open Access | Times Cited: 19
Physics-informed ConvNet: Learning physical field from a shallow neural network
Pengpeng Shi, Zhi Zeng, Tianshou Liang
Communications in Nonlinear Science and Numerical Simulation (2024) Vol. 132, pp. 107911-107911
Open Access | Times Cited: 17
Pengpeng Shi, Zhi Zeng, Tianshou Liang
Communications in Nonlinear Science and Numerical Simulation (2024) Vol. 132, pp. 107911-107911
Open Access | Times Cited: 17
KAN-ODEs: Kolmogorov–Arnold network ordinary differential equations for learning dynamical systems and hidden physics
Benjamin C. Koenig, Suyong Kim, Sili Deng
Computer Methods in Applied Mechanics and Engineering (2024) Vol. 432, pp. 117397-117397
Closed Access | Times Cited: 16
Benjamin C. Koenig, Suyong Kim, Sili Deng
Computer Methods in Applied Mechanics and Engineering (2024) Vol. 432, pp. 117397-117397
Closed Access | Times Cited: 16
SeismicNet: Physics-informed neural networks for seismic wave modeling in semi-infinite domain
Pu Ren, Chengping Rao, Su Chen, et al.
Computer Physics Communications (2023) Vol. 295, pp. 109010-109010
Open Access | Times Cited: 40
Pu Ren, Chengping Rao, Su Chen, et al.
Computer Physics Communications (2023) Vol. 295, pp. 109010-109010
Open Access | Times Cited: 40
Physics-informed neural network-based surrogate model for a virtual thermal sensor with real-time simulation
Myeong‐Seok Go, Jae Hyuk Lim, Seung‐Chul Lee
International Journal of Heat and Mass Transfer (2023) Vol. 214, pp. 124392-124392
Closed Access | Times Cited: 31
Myeong‐Seok Go, Jae Hyuk Lim, Seung‐Chul Lee
International Journal of Heat and Mass Transfer (2023) Vol. 214, pp. 124392-124392
Closed Access | Times Cited: 31
The Application of Physics-Informed Machine Learning in Multiphysics Modeling in Chemical Engineering
Zhi‐Yong Wu, Huan Wang, Chang He, et al.
Industrial & Engineering Chemistry Research (2023) Vol. 62, Iss. 44, pp. 18178-18204
Closed Access | Times Cited: 24
Zhi‐Yong Wu, Huan Wang, Chang He, et al.
Industrial & Engineering Chemistry Research (2023) Vol. 62, Iss. 44, pp. 18178-18204
Closed Access | Times Cited: 24
An unsupervised latent/output physics-informed convolutional-LSTM network for solving partial differential equations using peridynamic differential operator
Arda Mavi, Ali Can Bekar, Ehsan Haghighat, et al.
Computer Methods in Applied Mechanics and Engineering (2023) Vol. 407, pp. 115944-115944
Open Access | Times Cited: 23
Arda Mavi, Ali Can Bekar, Ehsan Haghighat, et al.
Computer Methods in Applied Mechanics and Engineering (2023) Vol. 407, pp. 115944-115944
Open Access | Times Cited: 23
Fast fluid–structure interaction simulation method based on deep learning flow field modeling
Jiawei Hu, Zihao Dou, Weiwei Zhang
Physics of Fluids (2024) Vol. 36, Iss. 4
Open Access | Times Cited: 13
Jiawei Hu, Zihao Dou, Weiwei Zhang
Physics of Fluids (2024) Vol. 36, Iss. 4
Open Access | Times Cited: 13
Neural Network-augmented Differentiable Finite Element Method for Boundary Value Problems
Xi Wang, Zhen‐Yu Yin, Wei Wu, et al.
International Journal of Mechanical Sciences (2024) Vol. 285, pp. 109783-109783
Closed Access | Times Cited: 11
Xi Wang, Zhen‐Yu Yin, Wei Wu, et al.
International Journal of Mechanical Sciences (2024) Vol. 285, pp. 109783-109783
Closed Access | Times Cited: 11
Variable linear transformation improved physics-informed neural networks to solve thin-layer flow problems
Jiahao Wu, Yuxin Wu, Guihua Zhang, et al.
Journal of Computational Physics (2024) Vol. 500, pp. 112761-112761
Closed Access | Times Cited: 10
Jiahao Wu, Yuxin Wu, Guihua Zhang, et al.
Journal of Computational Physics (2024) Vol. 500, pp. 112761-112761
Closed Access | Times Cited: 10
Solving spatiotemporal partial differential equations with Physics-informed Graph Neural Network
Zixue Xiang, Wei Peng, Wen Yao, et al.
Applied Soft Computing (2024) Vol. 155, pp. 111437-111437
Open Access | Times Cited: 9
Zixue Xiang, Wei Peng, Wen Yao, et al.
Applied Soft Computing (2024) Vol. 155, pp. 111437-111437
Open Access | Times Cited: 9
Physics-informed deep learning for solving phonon Boltzmann transport equation with large temperature non-equilibrium
Ruiyang Li, Jianxun Wang, Eungkyu Lee, et al.
npj Computational Materials (2022) Vol. 8, Iss. 1
Open Access | Times Cited: 32
Ruiyang Li, Jianxun Wang, Eungkyu Lee, et al.
npj Computational Materials (2022) Vol. 8, Iss. 1
Open Access | Times Cited: 32
PhySR: Physics-informed deep super-resolution for spatiotemporal data
Pu Ren, Chengping Rao, Yang Liu, et al.
Journal of Computational Physics (2023) Vol. 492, pp. 112438-112438
Closed Access | Times Cited: 21
Pu Ren, Chengping Rao, Yang Liu, et al.
Journal of Computational Physics (2023) Vol. 492, pp. 112438-112438
Closed Access | Times Cited: 21
Physics-guided recurrent neural network trained with approximate Bayesian computation: A case study on structural response prognostics
Juan Fernández, Juan Chiachío, José A. Cabezas, et al.
Reliability Engineering & System Safety (2023) Vol. 243, pp. 109822-109822
Open Access | Times Cited: 18
Juan Fernández, Juan Chiachío, José A. Cabezas, et al.
Reliability Engineering & System Safety (2023) Vol. 243, pp. 109822-109822
Open Access | Times Cited: 18
Physics-informed neural network for predicting hot-rolled steel temperatures during heating process
Yao Sun, Qianyue Zhang, Samar Raffoul
Journal of Engineering Research (2024)
Open Access | Times Cited: 7
Yao Sun, Qianyue Zhang, Samar Raffoul
Journal of Engineering Research (2024)
Open Access | Times Cited: 7
A finite element-based physics-informed operator learning framework for spatiotemporal partial differential equations on arbitrary domains
Yusuke Yamazaki, Ali M. Harandi, Mayu Muramatsu, et al.
Engineering With Computers (2024)
Open Access | Times Cited: 6
Yusuke Yamazaki, Ali M. Harandi, Mayu Muramatsu, et al.
Engineering With Computers (2024)
Open Access | Times Cited: 6
Physical laws meet machine intelligence: current developments and future directions
Temoor Muther, Amirmasoud Kalantari Dahaghi, Fahad I. Syed, et al.
Artificial Intelligence Review (2022) Vol. 56, Iss. 7, pp. 6947-7013
Closed Access | Times Cited: 27
Temoor Muther, Amirmasoud Kalantari Dahaghi, Fahad I. Syed, et al.
Artificial Intelligence Review (2022) Vol. 56, Iss. 7, pp. 6947-7013
Closed Access | Times Cited: 27
Accelerating hydrodynamic simulations of urban drainage systems with physics-guided machine learning
Rocco Palmitessa, Morten Grum, Allan P. Engsig‐Karup, et al.
Water Research (2022) Vol. 223, pp. 118972-118972
Open Access | Times Cited: 22
Rocco Palmitessa, Morten Grum, Allan P. Engsig‐Karup, et al.
Water Research (2022) Vol. 223, pp. 118972-118972
Open Access | Times Cited: 22