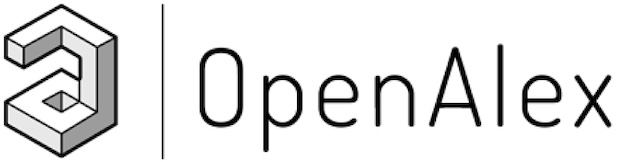
OpenAlex is a bibliographic catalogue of scientific papers, authors and institutions accessible in open access mode, named after the Library of Alexandria. It's citation coverage is excellent and I hope you will find utility in this listing of citing articles!
If you click the article title, you'll navigate to the article, as listed in CrossRef. If you click the Open Access links, you'll navigate to the "best Open Access location". Clicking the citation count will open this listing for that article. Lastly at the bottom of the page, you'll find basic pagination options.
Requested Article:
Artificial neural network application in comparison with modeling allometric equations for predicting above-ground biomass in the Hyrcanian mixed-beech forests of Iran
Ali Asghar Vahedi
Biomass and Bioenergy (2016) Vol. 88, pp. 66-76
Closed Access | Times Cited: 66
Ali Asghar Vahedi
Biomass and Bioenergy (2016) Vol. 88, pp. 66-76
Closed Access | Times Cited: 66
Showing 1-25 of 66 citing articles:
Modeling grassland above-ground biomass based on artificial neural network and remote sensing in the Three-River Headwaters Region
Shuxia Yang, Qisheng Feng, Tiangang Liang, et al.
Remote Sensing of Environment (2017) Vol. 204, pp. 448-455
Closed Access | Times Cited: 215
Shuxia Yang, Qisheng Feng, Tiangang Liang, et al.
Remote Sensing of Environment (2017) Vol. 204, pp. 448-455
Closed Access | Times Cited: 215
Application of machine-learning methods in forest ecology: recent progress and future challenges
Zelin Liu, Changhui Peng, Timothy T. Work, et al.
Environmental Reviews (2018) Vol. 26, Iss. 4, pp. 339-350
Open Access | Times Cited: 147
Zelin Liu, Changhui Peng, Timothy T. Work, et al.
Environmental Reviews (2018) Vol. 26, Iss. 4, pp. 339-350
Open Access | Times Cited: 147
Prognoses of diameter and height of trees of eucalyptus using artificial intelligence
Giovanni Correia Vieira, Adriano Ribeiro de Mendonça, Gilson Fernandes da Silva, et al.
The Science of The Total Environment (2017) Vol. 619-620, pp. 1473-1481
Closed Access | Times Cited: 79
Giovanni Correia Vieira, Adriano Ribeiro de Mendonça, Gilson Fernandes da Silva, et al.
The Science of The Total Environment (2017) Vol. 619-620, pp. 1473-1481
Closed Access | Times Cited: 79
Reduction in Uncertainty in Forest Aboveground Biomass Estimation Using Sentinel-2 Images: A Case Study of Pinus densata Forests in Shangri-La City, China
Lu Li, Boqi Zhou, Yanfeng Liu, et al.
Remote Sensing (2023) Vol. 15, Iss. 3, pp. 559-559
Open Access | Times Cited: 18
Lu Li, Boqi Zhou, Yanfeng Liu, et al.
Remote Sensing (2023) Vol. 15, Iss. 3, pp. 559-559
Open Access | Times Cited: 18
Estimating Spartina alterniflora fractional vegetation cover and aboveground biomass in a coastal wetland using SPOT6 satellite and UAV data
Zhou Zaiming, Yanming Yang, Chen Benqing
Aquatic Botany (2017) Vol. 144, pp. 38-45
Closed Access | Times Cited: 61
Zhou Zaiming, Yanming Yang, Chen Benqing
Aquatic Botany (2017) Vol. 144, pp. 38-45
Closed Access | Times Cited: 61
Artificial neural networks: Modeling tree survival and mortality in the Atlantic Forest biome in Brazil
Samuel José Silva Soares da Rocha, Carlos Moreira Miquelino Eleto Torres, Laércio Antônio Gonçalves Jacovine, et al.
The Science of The Total Environment (2018) Vol. 645, pp. 655-661
Closed Access | Times Cited: 56
Samuel José Silva Soares da Rocha, Carlos Moreira Miquelino Eleto Torres, Laércio Antônio Gonçalves Jacovine, et al.
The Science of The Total Environment (2018) Vol. 645, pp. 655-661
Closed Access | Times Cited: 56
Estimating forest carbon fluxes using four different data-driven techniques based on long-term eddy covariance measurements: Model comparison and evaluation
Xianming Dou, Yongguo Yang
The Science of The Total Environment (2018) Vol. 627, pp. 78-94
Closed Access | Times Cited: 48
Xianming Dou, Yongguo Yang
The Science of The Total Environment (2018) Vol. 627, pp. 78-94
Closed Access | Times Cited: 48
Machine learning models for estimating above ground biomass of fast growing trees
Warakhom Wongchai, Thossaporn Onsree, Natthida Sukkam, et al.
Expert Systems with Applications (2022) Vol. 199, pp. 117186-117186
Closed Access | Times Cited: 22
Warakhom Wongchai, Thossaporn Onsree, Natthida Sukkam, et al.
Expert Systems with Applications (2022) Vol. 199, pp. 117186-117186
Closed Access | Times Cited: 22
Estimation of maize above-ground biomass based on stem-leaf separation strategy integrated with LiDAR and optical remote sensing data
Yaohui Zhu, Chunjiang Zhao, Hao Yang, et al.
PeerJ (2019) Vol. 7, pp. e7593-e7593
Open Access | Times Cited: 40
Yaohui Zhu, Chunjiang Zhao, Hao Yang, et al.
PeerJ (2019) Vol. 7, pp. e7593-e7593
Open Access | Times Cited: 40
Impacts of climatic and edaphic factors on the diversity, structure and biomass of species-poor and structurally-complex forests
Arshad Ali, Anvar Sanaei, Mingshi Li, et al.
The Science of The Total Environment (2019) Vol. 706, pp. 135719-135719
Closed Access | Times Cited: 38
Arshad Ali, Anvar Sanaei, Mingshi Li, et al.
The Science of The Total Environment (2019) Vol. 706, pp. 135719-135719
Closed Access | Times Cited: 38
Modeling and estimating aboveground biomass of Dacrydium pierrei in China using machine learning with climate change
Chunyan Wu, Yongfu Chen, Changhui Peng, et al.
Journal of Environmental Management (2019) Vol. 234, pp. 167-179
Closed Access | Times Cited: 36
Chunyan Wu, Yongfu Chen, Changhui Peng, et al.
Journal of Environmental Management (2019) Vol. 234, pp. 167-179
Closed Access | Times Cited: 36
Big-trees – Energy mechanism underlies forest diversity and aboveground biomass
Arshad Ali, Anvar Sanaei, Mingshi Li, et al.
Forest Ecology and Management (2020) Vol. 461, pp. 117968-117968
Closed Access | Times Cited: 33
Arshad Ali, Anvar Sanaei, Mingshi Li, et al.
Forest Ecology and Management (2020) Vol. 461, pp. 117968-117968
Closed Access | Times Cited: 33
Development of Estimation Models for Individual Tree Aboveground Biomass Based on TLS-Derived Parameters
Fan Wang, Yuman Sun, Weiwei Jia, et al.
Forests (2023) Vol. 14, Iss. 2, pp. 351-351
Open Access | Times Cited: 12
Fan Wang, Yuman Sun, Weiwei Jia, et al.
Forests (2023) Vol. 14, Iss. 2, pp. 351-351
Open Access | Times Cited: 12
Random forest method for analysis of remote sensing inversion of aboveground biomass and grazing intensity of grasslands in Inner Mongolia, China
Shuai Wang, Hasi Tuya, Shengwei Zhang, et al.
International Journal of Remote Sensing (2023) Vol. 44, Iss. 9, pp. 2867-2884
Closed Access | Times Cited: 12
Shuai Wang, Hasi Tuya, Shengwei Zhang, et al.
International Journal of Remote Sensing (2023) Vol. 44, Iss. 9, pp. 2867-2884
Closed Access | Times Cited: 12
Tree-bark volume prediction via machine learning: A case study based on black alder’s tree-bark production
Maria J. Diamantopoulou, Ramazan Özçelík, Hakkı Yavuz
Computers and Electronics in Agriculture (2018) Vol. 151, pp. 431-440
Closed Access | Times Cited: 36
Maria J. Diamantopoulou, Ramazan Özçelík, Hakkı Yavuz
Computers and Electronics in Agriculture (2018) Vol. 151, pp. 431-440
Closed Access | Times Cited: 36
Artificial Neural Network Models: An Alternative Approach for Reliable Aboveground Pine Tree Biomass Prediction
Ramazan Özçelík, Maria J. Diamantopoulou, Mehmet Eker, et al.
Forest Science (2017) Vol. 63, Iss. 3, pp. 291-302
Open Access | Times Cited: 33
Ramazan Özçelík, Maria J. Diamantopoulou, Mehmet Eker, et al.
Forest Science (2017) Vol. 63, Iss. 3, pp. 291-302
Open Access | Times Cited: 33
Machine learning: Modeling increment in diameter of individual trees on Atlantic Forest fragments
Ivaldo da Silva Tavares Júnior, Carlos Moreira Miquelino Eleto Torres, Hélio Garcia Leite, et al.
Ecological Indicators (2020) Vol. 117, pp. 106685-106685
Open Access | Times Cited: 28
Ivaldo da Silva Tavares Júnior, Carlos Moreira Miquelino Eleto Torres, Hélio Garcia Leite, et al.
Ecological Indicators (2020) Vol. 117, pp. 106685-106685
Open Access | Times Cited: 28
LIDAR-Based Forest Biomass Remote Sensing: A Review of Metrics, Methods, and Assessment Criteria for the Selection of Allometric Equations
Abraham Aidoo Borsah, Majid Nazeer, Man Sing Wong
Forests (2023) Vol. 14, Iss. 10, pp. 2095-2095
Open Access | Times Cited: 9
Abraham Aidoo Borsah, Majid Nazeer, Man Sing Wong
Forests (2023) Vol. 14, Iss. 10, pp. 2095-2095
Open Access | Times Cited: 9
An alternative approach for estimating above ground biomass using Resourcesat-2 satellite data and artificial neural network in Bundelkhand region of India
Dibyendu Deb, J. P. Singh, Shovik Deb, et al.
Environmental Monitoring and Assessment (2017) Vol. 189, Iss. 11
Closed Access | Times Cited: 31
Dibyendu Deb, J. P. Singh, Shovik Deb, et al.
Environmental Monitoring and Assessment (2017) Vol. 189, Iss. 11
Closed Access | Times Cited: 31
Monitoring soil carbon pool in the Hyrcanian coastal plain forest of Iran: Artificial neural network application in comparison with developing traditional models
Ali Asghar Vahedi
CATENA (2017) Vol. 152, pp. 182-189
Closed Access | Times Cited: 29
Ali Asghar Vahedi
CATENA (2017) Vol. 152, pp. 182-189
Closed Access | Times Cited: 29
Remote-sensing inversion method for aboveground biomass of typical steppe in Inner Mongolia, China
Xin Lyu, Xiaobing Li, Jirui Gong, et al.
Ecological Indicators (2020) Vol. 120, pp. 106883-106883
Open Access | Times Cited: 27
Xin Lyu, Xiaobing Li, Jirui Gong, et al.
Ecological Indicators (2020) Vol. 120, pp. 106883-106883
Open Access | Times Cited: 27
Artificial Neural Networks and Linear Regression Reduce Sample Intensity to Predict the Commercial Volume of Eucalyptus Clones
Ivaldo da Silva Tavares Júnior, Jonas Elias Castro da Rocha, Ângelo Augusto Ebling, et al.
Forests (2019) Vol. 10, Iss. 3, pp. 268-268
Open Access | Times Cited: 26
Ivaldo da Silva Tavares Júnior, Jonas Elias Castro da Rocha, Ângelo Augusto Ebling, et al.
Forests (2019) Vol. 10, Iss. 3, pp. 268-268
Open Access | Times Cited: 26
Tree-size dimension inequality shapes aboveground carbon stock across temperate forest strata along environmental gradients
Maryam Kazempour Larsary, Hassan Pourbabaei, Anvar Sanaei, et al.
Forest Ecology and Management (2021) Vol. 496, pp. 119482-119482
Closed Access | Times Cited: 23
Maryam Kazempour Larsary, Hassan Pourbabaei, Anvar Sanaei, et al.
Forest Ecology and Management (2021) Vol. 496, pp. 119482-119482
Closed Access | Times Cited: 23
STEM TAPER ESTIMATIONS WITH ARTIFICIAL NEURAL NETWORKS FOR MIXED ORIENTAL BEECH AND KAZDAĞI FIR STANDS IN KARABÜK REGION, TURKEY
Oytun Emre Sakıcı, Gülay Özdemir
CERNE (2018) Vol. 24, Iss. 4, pp. 439-451
Open Access | Times Cited: 25
Oytun Emre Sakıcı, Gülay Özdemir
CERNE (2018) Vol. 24, Iss. 4, pp. 439-451
Open Access | Times Cited: 25
Comprehensive Evaluation of Machine Learning Techniques for Estimating the Responses of Carbon Fluxes to Climatic Forces in Different Terrestrial Ecosystems
Xianming Dou, Yongguo Yang
Atmosphere (2018) Vol. 9, Iss. 3, pp. 83-83
Open Access | Times Cited: 24
Xianming Dou, Yongguo Yang
Atmosphere (2018) Vol. 9, Iss. 3, pp. 83-83
Open Access | Times Cited: 24