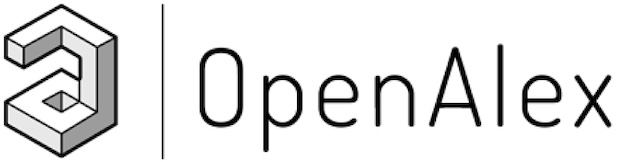
OpenAlex is a bibliographic catalogue of scientific papers, authors and institutions accessible in open access mode, named after the Library of Alexandria. It's citation coverage is excellent and I hope you will find utility in this listing of citing articles!
If you click the article title, you'll navigate to the article, as listed in CrossRef. If you click the Open Access links, you'll navigate to the "best Open Access location". Clicking the citation count will open this listing for that article. Lastly at the bottom of the page, you'll find basic pagination options.
Requested Article:
Runoff Forecasting using Convolutional Neural Networks and optimized Bi-directional Long Short-term Memory
Tunhua Wu, Zhaocai Wang, Yuan Hu, et al.
Water Resources Management (2023) Vol. 37, Iss. 2, pp. 937-953
Closed Access | Times Cited: 76
Tunhua Wu, Zhaocai Wang, Yuan Hu, et al.
Water Resources Management (2023) Vol. 37, Iss. 2, pp. 937-953
Closed Access | Times Cited: 76
Showing 1-25 of 76 citing articles:
An ensemble CNN-LSTM and GRU adaptive weighting model based improved sparrow search algorithm for predicting runoff using historical meteorological and runoff data as input
Zhiyuan Yao, Zhaocai Wang, Dangwei Wang, et al.
Journal of Hydrology (2023) Vol. 625, pp. 129977-129977
Closed Access | Times Cited: 92
Zhiyuan Yao, Zhaocai Wang, Dangwei Wang, et al.
Journal of Hydrology (2023) Vol. 625, pp. 129977-129977
Closed Access | Times Cited: 92
A review of hybrid deep learning applications for streamflow forecasting
Kin‐Wang Ng, Yuk Feng Huang, Chai Hoon Koo, et al.
Journal of Hydrology (2023) Vol. 625, pp. 130141-130141
Closed Access | Times Cited: 75
Kin‐Wang Ng, Yuk Feng Huang, Chai Hoon Koo, et al.
Journal of Hydrology (2023) Vol. 625, pp. 130141-130141
Closed Access | Times Cited: 75
A deep learning interpretable model for river dissolved oxygen multi-step and interval prediction based on multi-source data fusion
Zhaocai Wang, Qingyu Wang, Zhixiang Liu, et al.
Journal of Hydrology (2024) Vol. 629, pp. 130637-130637
Closed Access | Times Cited: 63
Zhaocai Wang, Qingyu Wang, Zhixiang Liu, et al.
Journal of Hydrology (2024) Vol. 629, pp. 130637-130637
Closed Access | Times Cited: 63
Single-Objective and Multi-Objective Flood Interval Forecasting Considering Interval Fitting Coefficients
Xinyu Chang, Jun Guo, Hui Qin, et al.
Water Resources Management (2024) Vol. 38, Iss. 10, pp. 3953-3972
Closed Access | Times Cited: 51
Xinyu Chang, Jun Guo, Hui Qin, et al.
Water Resources Management (2024) Vol. 38, Iss. 10, pp. 3953-3972
Closed Access | Times Cited: 51
Spatio-temporal deep learning model for accurate streamflow prediction with multi-source data fusion
Zhaocai Wang, Nannan Xu, Xiaoguang Bao, et al.
Environmental Modelling & Software (2024) Vol. 178, pp. 106091-106091
Closed Access | Times Cited: 33
Zhaocai Wang, Nannan Xu, Xiaoguang Bao, et al.
Environmental Modelling & Software (2024) Vol. 178, pp. 106091-106091
Closed Access | Times Cited: 33
A Novel Runoff Prediction Model Based on Support Vector Machine and Gate Recurrent unit with Secondary Mode Decomposition
Jinghan Dong, Zhaocai Wang, Tunhua Wu, et al.
Water Resources Management (2024) Vol. 38, Iss. 5, pp. 1655-1674
Closed Access | Times Cited: 27
Jinghan Dong, Zhaocai Wang, Tunhua Wu, et al.
Water Resources Management (2024) Vol. 38, Iss. 5, pp. 1655-1674
Closed Access | Times Cited: 27
A secondary modal decomposition ensemble deep learning model for groundwater level prediction using multi-data
Xuefei Cui, Zhaocai Wang, Nannan Xu, et al.
Environmental Modelling & Software (2024) Vol. 175, pp. 105969-105969
Closed Access | Times Cited: 23
Xuefei Cui, Zhaocai Wang, Nannan Xu, et al.
Environmental Modelling & Software (2024) Vol. 175, pp. 105969-105969
Closed Access | Times Cited: 23
Enhancing carbon price point-interval multi-step-ahead prediction using a hybrid framework of autoformer and extreme learning machine with multi-factors
Baoli Wang, Zhaocai Wang, Zhiyuan Yao
Expert Systems with Applications (2025), pp. 126467-126467
Closed Access | Times Cited: 4
Baoli Wang, Zhaocai Wang, Zhiyuan Yao
Expert Systems with Applications (2025), pp. 126467-126467
Closed Access | Times Cited: 4
A novel hybrid model based on deep learning and error correction for crude oil futures prices forecast
Tunhua Wu, Jinghan Dong, Zhaocai Wang, et al.
Resources Policy (2023) Vol. 83, pp. 103602-103602
Closed Access | Times Cited: 36
Tunhua Wu, Jinghan Dong, Zhaocai Wang, et al.
Resources Policy (2023) Vol. 83, pp. 103602-103602
Closed Access | Times Cited: 36
A new hybrid model for monthly runoff prediction using ELMAN neural network based on decomposition-integration structure with local error correction method
Dongmei Xu, Xiao-xue Hu, Wenchuan Wang, et al.
Expert Systems with Applications (2023) Vol. 238, pp. 121719-121719
Closed Access | Times Cited: 34
Dongmei Xu, Xiao-xue Hu, Wenchuan Wang, et al.
Expert Systems with Applications (2023) Vol. 238, pp. 121719-121719
Closed Access | Times Cited: 34
Robust Runoff Prediction With Explainable Artificial Intelligence and Meteorological Variables From Deep Learning Ensemble Model
Junhao Wu, Zhaocai Wang, Jinghan Dong, et al.
Water Resources Research (2023) Vol. 59, Iss. 9
Closed Access | Times Cited: 29
Junhao Wu, Zhaocai Wang, Jinghan Dong, et al.
Water Resources Research (2023) Vol. 59, Iss. 9
Closed Access | Times Cited: 29
A data-driven model for water quality prediction in Tai Lake, China, using secondary modal decomposition with multidimensional external features
Rui Tan, Zhaocai Wang, Tunhua Wu, et al.
Journal of Hydrology Regional Studies (2023) Vol. 47, pp. 101435-101435
Open Access | Times Cited: 28
Rui Tan, Zhaocai Wang, Tunhua Wu, et al.
Journal of Hydrology Regional Studies (2023) Vol. 47, pp. 101435-101435
Open Access | Times Cited: 28
An interpretable hybrid deep learning model for flood forecasting based on Transformer and LSTM
Wenzhong Li, Chengshuai Liu, Yingying Xu, et al.
Journal of Hydrology Regional Studies (2024) Vol. 54, pp. 101873-101873
Open Access | Times Cited: 13
Wenzhong Li, Chengshuai Liu, Yingying Xu, et al.
Journal of Hydrology Regional Studies (2024) Vol. 54, pp. 101873-101873
Open Access | Times Cited: 13
Enhancing Flooding Depth Forecasting Accuracy in an Urban Area Using a Novel Trend Forecasting Method
Song-Yue Yang, You-Da Jhong, Bing-Chen Jhong, et al.
Water Resources Management (2024) Vol. 38, Iss. 4, pp. 1359-1380
Closed Access | Times Cited: 9
Song-Yue Yang, You-Da Jhong, Bing-Chen Jhong, et al.
Water Resources Management (2024) Vol. 38, Iss. 4, pp. 1359-1380
Closed Access | Times Cited: 9
Evaluation and Interpretation of Runoff Forecasting Models Based on Hybrid Deep Neural Networks
Xin Yang, Jianzhong Zhou, Qianyi Zhang, et al.
Water Resources Management (2024) Vol. 38, Iss. 6, pp. 1987-2013
Closed Access | Times Cited: 9
Xin Yang, Jianzhong Zhou, Qianyi Zhang, et al.
Water Resources Management (2024) Vol. 38, Iss. 6, pp. 1987-2013
Closed Access | Times Cited: 9
A state-of-the-art review of long short-term memory models with applications in hydrology and water resources
Zhong-kai Feng, J. Zhang, Wen-jing Niu
Applied Soft Computing (2024), pp. 112352-112352
Closed Access | Times Cited: 8
Zhong-kai Feng, J. Zhang, Wen-jing Niu
Applied Soft Computing (2024), pp. 112352-112352
Closed Access | Times Cited: 8
Ensemble empirical mode decomposition based deep learning models for forecasting river flow time series
Reetun Maiti, Balagopal G. Menon, Anand Abraham
Expert Systems with Applications (2024) Vol. 255, pp. 124550-124550
Closed Access | Times Cited: 7
Reetun Maiti, Balagopal G. Menon, Anand Abraham
Expert Systems with Applications (2024) Vol. 255, pp. 124550-124550
Closed Access | Times Cited: 7
Rail Surface Defect Detection Based on Image Enhancement and Improved YOLOX
Chunguang Zhang, Donglin Xu, Lifang Zhang, et al.
Electronics (2023) Vol. 12, Iss. 12, pp. 2672-2672
Open Access | Times Cited: 18
Chunguang Zhang, Donglin Xu, Lifang Zhang, et al.
Electronics (2023) Vol. 12, Iss. 12, pp. 2672-2672
Open Access | Times Cited: 18
A Hybrid Data-Driven Deep Learning Prediction Framework for Lake Water Level Based on Fusion of Meteorological and Hydrological Multi-source Data
Zhiyuan Yao, Zhaocai Wang, Tunhua Wu, et al.
Natural Resources Research (2023) Vol. 33, Iss. 1, pp. 163-190
Closed Access | Times Cited: 17
Zhiyuan Yao, Zhaocai Wang, Tunhua Wu, et al.
Natural Resources Research (2023) Vol. 33, Iss. 1, pp. 163-190
Closed Access | Times Cited: 17
Simulation of Urban Flood Process Based on a Hybrid LSTM-SWMM Model
Chenchen Zhao, Chengshuai Liu, Wenzhong Li, et al.
Water Resources Management (2023) Vol. 37, Iss. 13, pp. 5171-5187
Open Access | Times Cited: 16
Chenchen Zhao, Chengshuai Liu, Wenzhong Li, et al.
Water Resources Management (2023) Vol. 37, Iss. 13, pp. 5171-5187
Open Access | Times Cited: 16
The influence of optimization algorithm on the signal prediction accuracy of VMD-LSTM for the pumped storage hydropower unit
Mingkun Fang, Fangfang Zhang, Yang Yang, et al.
Journal of Energy Storage (2023) Vol. 78, pp. 110187-110187
Closed Access | Times Cited: 16
Mingkun Fang, Fangfang Zhang, Yang Yang, et al.
Journal of Energy Storage (2023) Vol. 78, pp. 110187-110187
Closed Access | Times Cited: 16
A monthly temperature prediction based on the CEEMDAN–BO–BiLSTM coupled model
Xianqi Zhang, He Ren, Jiawen Liu, et al.
Scientific Reports (2024) Vol. 14, Iss. 1
Open Access | Times Cited: 6
Xianqi Zhang, He Ren, Jiawen Liu, et al.
Scientific Reports (2024) Vol. 14, Iss. 1
Open Access | Times Cited: 6
A Rapid Forecast Method for the Process of Flash Flood Based on Hydrodynamic Model and KNN Algorithm
Nie Zhou, Jingming Hou, Hua Chen, et al.
Water Resources Management (2024) Vol. 38, Iss. 6, pp. 1903-1919
Open Access | Times Cited: 6
Nie Zhou, Jingming Hou, Hua Chen, et al.
Water Resources Management (2024) Vol. 38, Iss. 6, pp. 1903-1919
Open Access | Times Cited: 6
SHAP-powered insights into spatiotemporal effects: Unlocking explainable Bayesian-neural-network urban flood forecasting
W. P. Chu, Chunxiao Zhang, Heng Li, et al.
International Journal of Applied Earth Observation and Geoinformation (2024) Vol. 131, pp. 103972-103972
Open Access | Times Cited: 6
W. P. Chu, Chunxiao Zhang, Heng Li, et al.
International Journal of Applied Earth Observation and Geoinformation (2024) Vol. 131, pp. 103972-103972
Open Access | Times Cited: 6
Advanced streamflow forecasting for Central European Rivers: The Cutting-Edge Kolmogorov-Arnold networks compared to Transformers
Francesco Granata, Senlin Zhu, Fabio Di Nunno
Journal of Hydrology (2024), pp. 132175-132175
Closed Access | Times Cited: 6
Francesco Granata, Senlin Zhu, Fabio Di Nunno
Journal of Hydrology (2024), pp. 132175-132175
Closed Access | Times Cited: 6