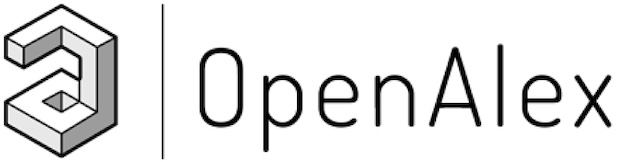
OpenAlex is a bibliographic catalogue of scientific papers, authors and institutions accessible in open access mode, named after the Library of Alexandria. It's citation coverage is excellent and I hope you will find utility in this listing of citing articles!
If you click the article title, you'll navigate to the article, as listed in CrossRef. If you click the Open Access links, you'll navigate to the "best Open Access location". Clicking the citation count will open this listing for that article. Lastly at the bottom of the page, you'll find basic pagination options.
Requested Article:
Predicting Formation Pore-Pressure from Well-Log Data with Hybrid Machine-Learning Optimization Algorithms
Mohammad Farsi, Nima Mohamadian, Hamzeh Ghorbani, et al.
Natural Resources Research (2021) Vol. 30, Iss. 5, pp. 3455-3481
Closed Access | Times Cited: 54
Mohammad Farsi, Nima Mohamadian, Hamzeh Ghorbani, et al.
Natural Resources Research (2021) Vol. 30, Iss. 5, pp. 3455-3481
Closed Access | Times Cited: 54
Showing 1-25 of 54 citing articles:
Predicting shear wave velocity from conventional well logs with deep and hybrid machine learning algorithms
Meysam Rajabi, Omid Hazbeh, Shadfar Davoodi, et al.
Journal of Petroleum Exploration and Production Technology (2022) Vol. 13, Iss. 1, pp. 19-42
Open Access | Times Cited: 54
Meysam Rajabi, Omid Hazbeh, Shadfar Davoodi, et al.
Journal of Petroleum Exploration and Production Technology (2022) Vol. 13, Iss. 1, pp. 19-42
Open Access | Times Cited: 54
Optimized machine learning models for natural fractures prediction using conventional well logs
Somayeh Tabasi, Pezhman Soltani Tehrani, Meysam Rajabi, et al.
Fuel (2022) Vol. 326, pp. 124952-124952
Closed Access | Times Cited: 49
Somayeh Tabasi, Pezhman Soltani Tehrani, Meysam Rajabi, et al.
Fuel (2022) Vol. 326, pp. 124952-124952
Closed Access | Times Cited: 49
Machine learning and data-driven prediction of pore pressure from geophysical logs: A case study for the Mangahewa gas field, New Zealand
Ahmed E. Radwan, David A. Wood, Ahmed A. Radwan
Journal of Rock Mechanics and Geotechnical Engineering (2022) Vol. 14, Iss. 6, pp. 1799-1809
Closed Access | Times Cited: 45
Ahmed E. Radwan, David A. Wood, Ahmed A. Radwan
Journal of Rock Mechanics and Geotechnical Engineering (2022) Vol. 14, Iss. 6, pp. 1799-1809
Closed Access | Times Cited: 45
A robust approach to pore pressure prediction applying petrophysical log data aided by machine learning techniques
Guodao Zhang, Shadfar Davoodi, Shahab S. Band, et al.
Energy Reports (2022) Vol. 8, pp. 2233-2247
Open Access | Times Cited: 38
Guodao Zhang, Shadfar Davoodi, Shahab S. Band, et al.
Energy Reports (2022) Vol. 8, pp. 2233-2247
Open Access | Times Cited: 38
Machine learning - a novel approach to predict the porosity curve using geophysical logs data: An example from the Lower Goru sand reservoir in the Southern Indus Basin, Pakistan
Wakeel Hussain, Miao Luo, Muhammad Ali, et al.
Journal of Applied Geophysics (2023) Vol. 214, pp. 105067-105067
Closed Access | Times Cited: 27
Wakeel Hussain, Miao Luo, Muhammad Ali, et al.
Journal of Applied Geophysics (2023) Vol. 214, pp. 105067-105067
Closed Access | Times Cited: 27
Robust hybrid machine learning algorithms for gas flow rates prediction through wellhead chokes in gas condensate fields
Abouzar Rajabi Behesht Abad, Hamzeh Ghorbani, Nima Mohamadian, et al.
Fuel (2021) Vol. 308, pp. 121872-121872
Open Access | Times Cited: 52
Abouzar Rajabi Behesht Abad, Hamzeh Ghorbani, Nima Mohamadian, et al.
Fuel (2021) Vol. 308, pp. 121872-121872
Open Access | Times Cited: 52
Hybrid machine learning algorithms to predict condensate viscosity in the near wellbore regions of gas condensate reservoirs
Abouzar Rajabi Behesht Abad, Seyedmohammadvahid Mousavi, Nima Mohamadian, et al.
Journal of Natural Gas Science and Engineering (2021) Vol. 95, pp. 104210-104210
Closed Access | Times Cited: 47
Abouzar Rajabi Behesht Abad, Seyedmohammadvahid Mousavi, Nima Mohamadian, et al.
Journal of Natural Gas Science and Engineering (2021) Vol. 95, pp. 104210-104210
Closed Access | Times Cited: 47
Novel hybrid machine learning optimizer algorithms to prediction of fracture density by petrophysical data
Meysam Rajabi, Saeed Beheshtian, Shadfar Davoodi, et al.
Journal of Petroleum Exploration and Production Technology (2021) Vol. 11, Iss. 12, pp. 4375-4397
Open Access | Times Cited: 44
Meysam Rajabi, Saeed Beheshtian, Shadfar Davoodi, et al.
Journal of Petroleum Exploration and Production Technology (2021) Vol. 11, Iss. 12, pp. 4375-4397
Open Access | Times Cited: 44
Prediction method for formation pore pressure based on transfer learning
Yuqiang Xu, Lei Yang, Jiaxing Xu, et al.
Geoenergy Science and Engineering (2024) Vol. 236, pp. 212747-212747
Closed Access | Times Cited: 5
Yuqiang Xu, Lei Yang, Jiaxing Xu, et al.
Geoenergy Science and Engineering (2024) Vol. 236, pp. 212747-212747
Closed Access | Times Cited: 5
Artificial intelligence in geoenergy: bridging petroleum engineering and future-oriented applications
Sungil Kim, Tea-Woo Kim, Suryeom Jo
Journal of Petroleum Exploration and Production Technology (2025) Vol. 15, Iss. 2
Open Access
Sungil Kim, Tea-Woo Kim, Suryeom Jo
Journal of Petroleum Exploration and Production Technology (2025) Vol. 15, Iss. 2
Open Access
Predicting oil flow rate through orifice plate with robust machine learning algorithms
Abouzar Rajabi Behesht Abad, Pezhman Soltani Tehrani, Mohammad Naveshki, et al.
Flow Measurement and Instrumentation (2021) Vol. 81, pp. 102047-102047
Closed Access | Times Cited: 39
Abouzar Rajabi Behesht Abad, Pezhman Soltani Tehrani, Mohammad Naveshki, et al.
Flow Measurement and Instrumentation (2021) Vol. 81, pp. 102047-102047
Closed Access | Times Cited: 39
A novel approach to pore pressure modeling based on conventional well logs using convolutional neural network
Morteza Matinkia, Ali Amraeiniya, Mohammad Mohammadi Behboud, et al.
Journal of Petroleum Science and Engineering (2022) Vol. 211, pp. 110156-110156
Closed Access | Times Cited: 26
Morteza Matinkia, Ali Amraeiniya, Mohammad Mohammadi Behboud, et al.
Journal of Petroleum Science and Engineering (2022) Vol. 211, pp. 110156-110156
Closed Access | Times Cited: 26
Data driven models to predict pore pressure using drilling and petrophysical data
Farshad Jafarizadeh, Meysam Rajabi, Somayeh Tabasi, et al.
Energy Reports (2022) Vol. 8, pp. 6551-6562
Open Access | Times Cited: 25
Farshad Jafarizadeh, Meysam Rajabi, Somayeh Tabasi, et al.
Energy Reports (2022) Vol. 8, pp. 6551-6562
Open Access | Times Cited: 25
Pore pressure prediction in offshore Niger delta using data-driven approach: Implications on drilling and reservoir quality
Joshua Pwavodi, Ibekwe N. Kelechi, Perekebina Angalabiri, et al.
Energy Geoscience (2023) Vol. 4, Iss. 3, pp. 100194-100194
Open Access | Times Cited: 14
Joshua Pwavodi, Ibekwe N. Kelechi, Perekebina Angalabiri, et al.
Energy Geoscience (2023) Vol. 4, Iss. 3, pp. 100194-100194
Open Access | Times Cited: 14
Application of GMDH to Predict Pore Pressure from Well Logs Data: A Case Study from Southeast Sichuan Basin, China
Melckzedeck Michael Mgimba, Shu Jiang, Edwin E. Nyakilla, et al.
Natural Resources Research (2023) Vol. 32, Iss. 4, pp. 1711-1731
Closed Access | Times Cited: 13
Melckzedeck Michael Mgimba, Shu Jiang, Edwin E. Nyakilla, et al.
Natural Resources Research (2023) Vol. 32, Iss. 4, pp. 1711-1731
Closed Access | Times Cited: 13
Predicting corrosion behaviour of steel reinforcement in eco-friendly coral aggregate concrete based on hybrid machine learning methods
Zhen Sun, Yalin Li, Li Su, et al.
Nondestructive Testing And Evaluation (2024), pp. 1-21
Closed Access | Times Cited: 4
Zhen Sun, Yalin Li, Li Su, et al.
Nondestructive Testing And Evaluation (2024), pp. 1-21
Closed Access | Times Cited: 4
Ensemble learning-based interpretable method for pore pressure prediction using multivariate well logging data of IODP site U1517
Goutami Das, Saumen Maiti
Earth Science Informatics (2025) Vol. 18, Iss. 2
Closed Access
Goutami Das, Saumen Maiti
Earth Science Informatics (2025) Vol. 18, Iss. 2
Closed Access
Evaluating the effectiveness of ensemble machine learning approaches for pore pressure prediction using petrophysical log data in carbonate reservoir
Pydiraju Yalamanchi, Saurabh Datta Gupta, Rajeev Upadhyay
Acta Geophysica (2025)
Closed Access
Pydiraju Yalamanchi, Saurabh Datta Gupta, Rajeev Upadhyay
Acta Geophysica (2025)
Closed Access
Robust computational approach to determine the safe mud weight window using well-log data from a large gas reservoir
Saeed Beheshtian, Meysam Rajabi, Shadfar Davoodi, et al.
Marine and Petroleum Geology (2022) Vol. 142, pp. 105772-105772
Closed Access | Times Cited: 19
Saeed Beheshtian, Meysam Rajabi, Shadfar Davoodi, et al.
Marine and Petroleum Geology (2022) Vol. 142, pp. 105772-105772
Closed Access | Times Cited: 19
Pore Pressure Prediction by Empirical and Machine Learning Methods Using Conventional and Drilling Logs in Carbonate Rocks
Mohammad Reza Delavar, Ahmad Ramezanzadeh
Rock Mechanics and Rock Engineering (2022) Vol. 56, Iss. 1, pp. 535-564
Closed Access | Times Cited: 19
Mohammad Reza Delavar, Ahmad Ramezanzadeh
Rock Mechanics and Rock Engineering (2022) Vol. 56, Iss. 1, pp. 535-564
Closed Access | Times Cited: 19
Prediction of fracture density in a gas reservoir using robust computational approaches
Guozhong Gao, Omid Hazbeh, Shadfar Davoodi, et al.
Frontiers in Earth Science (2023) Vol. 10
Open Access | Times Cited: 10
Guozhong Gao, Omid Hazbeh, Shadfar Davoodi, et al.
Frontiers in Earth Science (2023) Vol. 10
Open Access | Times Cited: 10
Research on prediction methods of formation pore pressure based on machine learning
Honglin Huang, Jun Li, Hongwei Yang, et al.
Energy Science & Engineering (2022) Vol. 10, Iss. 6, pp. 1886-1901
Open Access | Times Cited: 15
Honglin Huang, Jun Li, Hongwei Yang, et al.
Energy Science & Engineering (2022) Vol. 10, Iss. 6, pp. 1886-1901
Open Access | Times Cited: 15
Pore pressure prediction assisted by machine learning models combined with interpretations: A case study of an HTHP gas field, Yinggehai Basin
Xiaobo Zhao, Xiaojun Chen, Zhangjian Lan, et al.
Geoenergy Science and Engineering (2023) Vol. 229, pp. 212114-212114
Closed Access | Times Cited: 8
Xiaobo Zhao, Xiaojun Chen, Zhangjian Lan, et al.
Geoenergy Science and Engineering (2023) Vol. 229, pp. 212114-212114
Closed Access | Times Cited: 8
Research progress of machine-learning algorithm for formation pore pressure prediction
Haoyu Pan, Song Deng, Chaowei Li, et al.
Petroleum Science and Technology (2024), pp. 1-19
Closed Access | Times Cited: 2
Haoyu Pan, Song Deng, Chaowei Li, et al.
Petroleum Science and Technology (2024), pp. 1-19
Closed Access | Times Cited: 2
Mining fuzzy local periodic activity pattern for Smart home applications
Mohammad Iqbal, Adila Sekarrati Dwi Prayitno, Hsing-Kuo Pao, et al.
Knowledge-Based Systems (2024) Vol. 293, pp. 111629-111629
Closed Access | Times Cited: 2
Mohammad Iqbal, Adila Sekarrati Dwi Prayitno, Hsing-Kuo Pao, et al.
Knowledge-Based Systems (2024) Vol. 293, pp. 111629-111629
Closed Access | Times Cited: 2