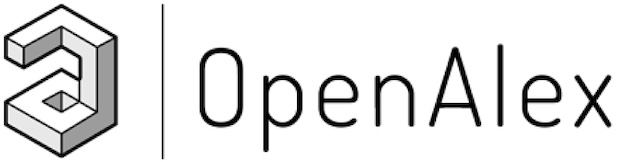
OpenAlex is a bibliographic catalogue of scientific papers, authors and institutions accessible in open access mode, named after the Library of Alexandria. It's citation coverage is excellent and I hope you will find utility in this listing of citing articles!
If you click the article title, you'll navigate to the article, as listed in CrossRef. If you click the Open Access links, you'll navigate to the "best Open Access location". Clicking the citation count will open this listing for that article. Lastly at the bottom of the page, you'll find basic pagination options.
Requested Article:
A survey on multi-agent deep reinforcement learning: from the perspective of challenges and applications
Wei Du, Shifei Ding
Artificial Intelligence Review (2020) Vol. 54, Iss. 5, pp. 3215-3238
Closed Access | Times Cited: 178
Wei Du, Shifei Ding
Artificial Intelligence Review (2020) Vol. 54, Iss. 5, pp. 3215-3238
Closed Access | Times Cited: 178
Showing 1-25 of 178 citing articles:
A survey on deep learning tools dealing with data scarcity: definitions, challenges, solutions, tips, and applications
Laith Alzubaidi, Jinshuai Bai, Aiman Al-Sabaawi, et al.
Journal Of Big Data (2023) Vol. 10, Iss. 1
Open Access | Times Cited: 320
Laith Alzubaidi, Jinshuai Bai, Aiman Al-Sabaawi, et al.
Journal Of Big Data (2023) Vol. 10, Iss. 1
Open Access | Times Cited: 320
Reinforcement learning algorithms: A brief survey
Ashish Kumar Shakya, G. N. Pillai, Sohom Chakrabarty
Expert Systems with Applications (2023) Vol. 231, pp. 120495-120495
Closed Access | Times Cited: 153
Ashish Kumar Shakya, G. N. Pillai, Sohom Chakrabarty
Expert Systems with Applications (2023) Vol. 231, pp. 120495-120495
Closed Access | Times Cited: 153
Machine Learning Applications in Internet-of-Drones: Systematic Review, Recent Deployments, and Open Issues
Arash Heidari, Nima Jafari Navimipour, Mehmet Ünal, et al.
ACM Computing Surveys (2022) Vol. 55, Iss. 12, pp. 1-45
Closed Access | Times Cited: 115
Arash Heidari, Nima Jafari Navimipour, Mehmet Ünal, et al.
ACM Computing Surveys (2022) Vol. 55, Iss. 12, pp. 1-45
Closed Access | Times Cited: 115
Pervasive AI for IoT Applications: A Survey on Resource-Efficient Distributed Artificial Intelligence
Emna Baccour, Naram Mhaisen, Alaa Awad Abdellatif, et al.
IEEE Communications Surveys & Tutorials (2022) Vol. 24, Iss. 4, pp. 2366-2418
Open Access | Times Cited: 81
Emna Baccour, Naram Mhaisen, Alaa Awad Abdellatif, et al.
IEEE Communications Surveys & Tutorials (2022) Vol. 24, Iss. 4, pp. 2366-2418
Open Access | Times Cited: 81
Deep multiagent reinforcement learning: challenges and directions
Annie Wong, Thomas Bäck, Anna V. Kononova, et al.
Artificial Intelligence Review (2022) Vol. 56, Iss. 6, pp. 5023-5056
Open Access | Times Cited: 74
Annie Wong, Thomas Bäck, Anna V. Kononova, et al.
Artificial Intelligence Review (2022) Vol. 56, Iss. 6, pp. 5023-5056
Open Access | Times Cited: 74
Multi-Agent Deep Reinforcement Learning for Multi-Robot Applications: A Survey
James Orr, Ayan Dutta
Sensors (2023) Vol. 23, Iss. 7, pp. 3625-3625
Open Access | Times Cited: 61
James Orr, Ayan Dutta
Sensors (2023) Vol. 23, Iss. 7, pp. 3625-3625
Open Access | Times Cited: 61
Toward Autonomous Multi-UAV Wireless Network: A Survey of Reinforcement Learning-Based Approaches
Yu Bai, Huijun Zhao, X. L. Zhang, et al.
IEEE Communications Surveys & Tutorials (2023) Vol. 25, Iss. 4, pp. 3038-3067
Open Access | Times Cited: 60
Yu Bai, Huijun Zhao, X. L. Zhang, et al.
IEEE Communications Surveys & Tutorials (2023) Vol. 25, Iss. 4, pp. 3038-3067
Open Access | Times Cited: 60
A novel reinforcement learning based tuna swarm optimization algorithm for autonomous underwater vehicle path planning
Zheping Yan, Jinyu Yan, Yifan Wu, et al.
Mathematics and Computers in Simulation (2023) Vol. 209, pp. 55-86
Closed Access | Times Cited: 42
Zheping Yan, Jinyu Yan, Yifan Wu, et al.
Mathematics and Computers in Simulation (2023) Vol. 209, pp. 55-86
Closed Access | Times Cited: 42
A critical review of safe reinforcement learning strategies in power and energy systems
Van‐Hai Bui, Sina Mohammadi, Srijita Das, et al.
Engineering Applications of Artificial Intelligence (2025) Vol. 143, pp. 110091-110091
Closed Access | Times Cited: 1
Van‐Hai Bui, Sina Mohammadi, Srijita Das, et al.
Engineering Applications of Artificial Intelligence (2025) Vol. 143, pp. 110091-110091
Closed Access | Times Cited: 1
The COVID-19 epidemic analysis and diagnosis using deep learning: A systematic literature review and future directions
Arash Heidari, Nima Jafari Navimipour, Mehmet Ünal, et al.
Computers in Biology and Medicine (2021) Vol. 141, pp. 105141-105141
Open Access | Times Cited: 76
Arash Heidari, Nima Jafari Navimipour, Mehmet Ünal, et al.
Computers in Biology and Medicine (2021) Vol. 141, pp. 105141-105141
Open Access | Times Cited: 76
Dynamic scheduling of tasks in cloud manufacturing with multi-agent reinforcement learning
Xiaohan Wang, Zhang Li, Yongkui Liu, et al.
Journal of Manufacturing Systems (2022) Vol. 65, pp. 130-145
Closed Access | Times Cited: 51
Xiaohan Wang, Zhang Li, Yongkui Liu, et al.
Journal of Manufacturing Systems (2022) Vol. 65, pp. 130-145
Closed Access | Times Cited: 51
Coverage path planning for kiwifruit picking robots based on deep reinforcement learning
Yinchu Wang, Zhi He, Dandan Cao, et al.
Computers and Electronics in Agriculture (2022) Vol. 205, pp. 107593-107593
Closed Access | Times Cited: 48
Yinchu Wang, Zhi He, Dandan Cao, et al.
Computers and Electronics in Agriculture (2022) Vol. 205, pp. 107593-107593
Closed Access | Times Cited: 48
Reinforcement-Learning-Based Vibration Control for a Vehicle Semi-Active Suspension System via the PPO Approach
Shiyuan Han, Tong Liang
Applied Sciences (2022) Vol. 12, Iss. 6, pp. 3078-3078
Open Access | Times Cited: 38
Shiyuan Han, Tong Liang
Applied Sciences (2022) Vol. 12, Iss. 6, pp. 3078-3078
Open Access | Times Cited: 38
Deep Reinforcement Learning Versus Evolution Strategies: A Comparative Survey
Amjad Yousef Majid, Serge Saaybi, Vincent François-Lavet, et al.
IEEE Transactions on Neural Networks and Learning Systems (2023) Vol. 35, Iss. 9, pp. 11939-11957
Open Access | Times Cited: 31
Amjad Yousef Majid, Serge Saaybi, Vincent François-Lavet, et al.
IEEE Transactions on Neural Networks and Learning Systems (2023) Vol. 35, Iss. 9, pp. 11939-11957
Open Access | Times Cited: 31
Machine Learning: Models, Challenges, and Research Directions
Tala Talaei Khoei, Naima Kaabouch
Future Internet (2023) Vol. 15, Iss. 10, pp. 332-332
Open Access | Times Cited: 24
Tala Talaei Khoei, Naima Kaabouch
Future Internet (2023) Vol. 15, Iss. 10, pp. 332-332
Open Access | Times Cited: 24
A Comprehensive Survey on Multi-Agent Reinforcement Learning for Connected and Automated Vehicles
Pamul Yadav, Ashutosh Mishra, Shiho Kim
Sensors (2023) Vol. 23, Iss. 10, pp. 4710-4710
Open Access | Times Cited: 22
Pamul Yadav, Ashutosh Mishra, Shiho Kim
Sensors (2023) Vol. 23, Iss. 10, pp. 4710-4710
Open Access | Times Cited: 22
Multi-Agent Reinforcement Learning With Privacy Preservation for Continuous Double Auction-Based P2P Energy Trading
Jiehui Zheng, Ze-Ting Liang, Yuanzheng Li, et al.
IEEE Transactions on Industrial Informatics (2024) Vol. 20, Iss. 4, pp. 6582-6590
Closed Access | Times Cited: 13
Jiehui Zheng, Ze-Ting Liang, Yuanzheng Li, et al.
IEEE Transactions on Industrial Informatics (2024) Vol. 20, Iss. 4, pp. 6582-6590
Closed Access | Times Cited: 13
A survey on multi-agent reinforcement learning and its application
Zepeng Ning, Lihua Xie
Journal of Automation and Intelligence (2024) Vol. 3, Iss. 2, pp. 73-91
Open Access | Times Cited: 13
Zepeng Ning, Lihua Xie
Journal of Automation and Intelligence (2024) Vol. 3, Iss. 2, pp. 73-91
Open Access | Times Cited: 13
Novel task decomposed multi-agent twin delayed deep deterministic policy gradient algorithm for multi-UAV autonomous path planning
Yatong Zhou, Xiaoran Kong, Kuo-Ping Lin, et al.
Knowledge-Based Systems (2024) Vol. 287, pp. 111462-111462
Closed Access | Times Cited: 11
Yatong Zhou, Xiaoran Kong, Kuo-Ping Lin, et al.
Knowledge-Based Systems (2024) Vol. 287, pp. 111462-111462
Closed Access | Times Cited: 11
Learning safe control for multi-robot systems: Methods, verification, and open challenges
Kunal Garg, Songyuan Zhang, Oswin So, et al.
Annual Reviews in Control (2024) Vol. 57, pp. 100948-100948
Open Access | Times Cited: 10
Kunal Garg, Songyuan Zhang, Oswin So, et al.
Annual Reviews in Control (2024) Vol. 57, pp. 100948-100948
Open Access | Times Cited: 10
Multi-Agent Incentive Communication via Decentralized Teammate Modeling
Lei Yuan, Jianhao Wang, Fuxiang Zhang, et al.
Proceedings of the AAAI Conference on Artificial Intelligence (2022) Vol. 36, Iss. 9, pp. 9466-9474
Open Access | Times Cited: 33
Lei Yuan, Jianhao Wang, Fuxiang Zhang, et al.
Proceedings of the AAAI Conference on Artificial Intelligence (2022) Vol. 36, Iss. 9, pp. 9466-9474
Open Access | Times Cited: 33
Multi-type data fusion framework based on deep reinforcement learning for algorithmic trading
Peipei Liu, Yunfeng Zhang, Fangxun Bao, et al.
Applied Intelligence (2022) Vol. 53, Iss. 2, pp. 1683-1706
Closed Access | Times Cited: 31
Peipei Liu, Yunfeng Zhang, Fangxun Bao, et al.
Applied Intelligence (2022) Vol. 53, Iss. 2, pp. 1683-1706
Closed Access | Times Cited: 31
Advances of machine learning in materials science: Ideas and techniques
Sue Sin Chong, Yi Sheng Ng, Hui‐Qiong Wang, et al.
Frontiers of Physics (2023) Vol. 19, Iss. 1
Open Access | Times Cited: 21
Sue Sin Chong, Yi Sheng Ng, Hui‐Qiong Wang, et al.
Frontiers of Physics (2023) Vol. 19, Iss. 1
Open Access | Times Cited: 21
Recent developments and future trends in fatigue life assessment of additively manufactured metals with particular emphasis on machine learning modeling
Zhixin Zhan, Xiaofan He, Dingcheng Tang, et al.
Fatigue & Fracture of Engineering Materials & Structures (2023) Vol. 46, Iss. 12, pp. 4425-4464
Closed Access | Times Cited: 21
Zhixin Zhan, Xiaofan He, Dingcheng Tang, et al.
Fatigue & Fracture of Engineering Materials & Structures (2023) Vol. 46, Iss. 12, pp. 4425-4464
Closed Access | Times Cited: 21
A deep reinforcement learning method to control chaos synchronization between two identical chaotic systems
Haoxin Cheng, Haihong Li, Qionglin Dai, et al.
Chaos Solitons & Fractals (2023) Vol. 174, pp. 113809-113809
Closed Access | Times Cited: 18
Haoxin Cheng, Haihong Li, Qionglin Dai, et al.
Chaos Solitons & Fractals (2023) Vol. 174, pp. 113809-113809
Closed Access | Times Cited: 18