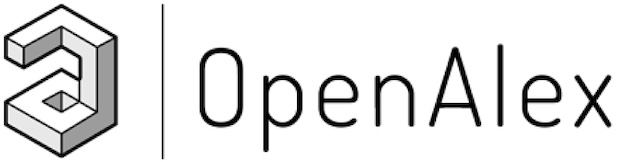
OpenAlex is a bibliographic catalogue of scientific papers, authors and institutions accessible in open access mode, named after the Library of Alexandria. It's citation coverage is excellent and I hope you will find utility in this listing of citing articles!
If you click the article title, you'll navigate to the article, as listed in CrossRef. If you click the Open Access links, you'll navigate to the "best Open Access location". Clicking the citation count will open this listing for that article. Lastly at the bottom of the page, you'll find basic pagination options.
Requested Article:
Finite basis physics-informed neural networks (FBPINNs): a scalable domain decomposition approach for solving differential equations
Ben Moseley, Andrew Markham, Tarje Nissen‐Meyer
Advances in Computational Mathematics (2023) Vol. 49, Iss. 4
Open Access | Times Cited: 129
Ben Moseley, Andrew Markham, Tarje Nissen‐Meyer
Advances in Computational Mathematics (2023) Vol. 49, Iss. 4
Open Access | Times Cited: 129
Showing 1-25 of 129 citing articles:
Transfer learning based physics-informed neural networks for solving inverse problems in engineering structures under different loading scenarios
Xu Chen, Ba Trung Cao, Yong Yuan, et al.
Computer Methods in Applied Mechanics and Engineering (2022) Vol. 405, pp. 115852-115852
Open Access | Times Cited: 113
Xu Chen, Ba Trung Cao, Yong Yuan, et al.
Computer Methods in Applied Mechanics and Engineering (2022) Vol. 405, pp. 115852-115852
Open Access | Times Cited: 113
Augmented Physics-Informed Neural Networks (APINNs): A gating network-based soft domain decomposition methodology
Zheyuan Hu, Ameya D. Jagtap, George Em Karniadakis, et al.
Engineering Applications of Artificial Intelligence (2023) Vol. 126, pp. 107183-107183
Open Access | Times Cited: 72
Zheyuan Hu, Ameya D. Jagtap, George Em Karniadakis, et al.
Engineering Applications of Artificial Intelligence (2023) Vol. 126, pp. 107183-107183
Open Access | Times Cited: 72
Can physics-informed neural networks beat the finite element method?
Tamara G. Grossmann, Urszula Julia Komorowska, Jonas Latz, et al.
IMA Journal of Applied Mathematics (2024) Vol. 89, Iss. 1, pp. 143-174
Open Access | Times Cited: 72
Tamara G. Grossmann, Urszula Julia Komorowska, Jonas Latz, et al.
IMA Journal of Applied Mathematics (2024) Vol. 89, Iss. 1, pp. 143-174
Open Access | Times Cited: 72
Respecting causality for training physics-informed neural networks
Sifan Wang, Shyam Sankaran, Paris Perdikaris
Computer Methods in Applied Mechanics and Engineering (2024) Vol. 421, pp. 116813-116813
Open Access | Times Cited: 60
Sifan Wang, Shyam Sankaran, Paris Perdikaris
Computer Methods in Applied Mechanics and Engineering (2024) Vol. 421, pp. 116813-116813
Open Access | Times Cited: 60
Multilevel domain decomposition-based architectures for physics-informed neural networks
Victorita Dolean, Alexander Heinlein, Siddhartha Mishra, et al.
Computer Methods in Applied Mechanics and Engineering (2024) Vol. 429, pp. 117116-117116
Open Access | Times Cited: 22
Victorita Dolean, Alexander Heinlein, Siddhartha Mishra, et al.
Computer Methods in Applied Mechanics and Engineering (2024) Vol. 429, pp. 117116-117116
Open Access | Times Cited: 22
From PINNs to PIKANs: recent advances in physics-informed machine learning
Juan Diego Toscano, Vivek Oommen, Alan John Varghese, et al.
Machine learning for computational science and engineering (2025) Vol. 1, Iss. 1
Closed Access | Times Cited: 3
Juan Diego Toscano, Vivek Oommen, Alan John Varghese, et al.
Machine learning for computational science and engineering (2025) Vol. 1, Iss. 1
Closed Access | Times Cited: 3
AT-PINN-HC: A refined time-sequential method incorporating hard-constraint strategies for predicting structural behavior under dynamic loads
Zhaolin Chen, S.K. Lai, Zhicheng Yang, et al.
Computer Methods in Applied Mechanics and Engineering (2025) Vol. 436, pp. 117691-117691
Closed Access | Times Cited: 1
Zhaolin Chen, S.K. Lai, Zhicheng Yang, et al.
Computer Methods in Applied Mechanics and Engineering (2025) Vol. 436, pp. 117691-117691
Closed Access | Times Cited: 1
Physics-informed machine learning model for computational fracture of quasi-brittle materials without labelled data
Bin Zheng, Tongchun Li, Huijun Qi, et al.
International Journal of Mechanical Sciences (2022) Vol. 223, pp. 107282-107282
Closed Access | Times Cited: 64
Bin Zheng, Tongchun Li, Huijun Qi, et al.
International Journal of Mechanical Sciences (2022) Vol. 223, pp. 107282-107282
Closed Access | Times Cited: 64
Gradient-optimized physics-informed neural networks (GOPINNs): a deep learning method for solving the complex modified KdV equation
Jiaheng Li, Junchao Chen, Biao Li
Nonlinear Dynamics (2021) Vol. 107, Iss. 1, pp. 781-792
Open Access | Times Cited: 59
Jiaheng Li, Junchao Chen, Biao Li
Nonlinear Dynamics (2021) Vol. 107, Iss. 1, pp. 781-792
Open Access | Times Cited: 59
Rapid Seismic Waveform Modeling and Inversion With Neural Operators
Yan Yang, Angela F. Gao, Kamyar Azizzadenesheli, et al.
IEEE Transactions on Geoscience and Remote Sensing (2023) Vol. 61, pp. 1-12
Open Access | Times Cited: 22
Yan Yang, Angela F. Gao, Kamyar Azizzadenesheli, et al.
IEEE Transactions on Geoscience and Remote Sensing (2023) Vol. 61, pp. 1-12
Open Access | Times Cited: 22
A practical PINN framework for multi-scale problems with multi-magnitude loss terms
Yong Wang, Yanzhong Yao, Jiawei Guo, et al.
Journal of Computational Physics (2024) Vol. 510, pp. 113112-113112
Open Access | Times Cited: 14
Yong Wang, Yanzhong Yao, Jiawei Guo, et al.
Journal of Computational Physics (2024) Vol. 510, pp. 113112-113112
Open Access | Times Cited: 14
A comprehensive review of advances in physics-informed neural networks and their applications in complex fluid dynamics
Chi Zhao, Feifei Zhang, Wenqiang Lou, et al.
Physics of Fluids (2024) Vol. 36, Iss. 10
Closed Access | Times Cited: 11
Chi Zhao, Feifei Zhang, Wenqiang Lou, et al.
Physics of Fluids (2024) Vol. 36, Iss. 10
Closed Access | Times Cited: 11
Enhancing Training of Physics-Informed Neural Networks Using Domain Decomposition–Based Preconditioning Strategies
Alena Kopaničáková, Hardik Kothari, George Em Karniadakis, et al.
SIAM Journal on Scientific Computing (2024) Vol. 46, Iss. 5, pp. S46-S67
Closed Access | Times Cited: 10
Alena Kopaničáková, Hardik Kothari, George Em Karniadakis, et al.
SIAM Journal on Scientific Computing (2024) Vol. 46, Iss. 5, pp. S46-S67
Closed Access | Times Cited: 10
Physics-infused deep neural network for solution of non-associative Drucker–Prager elastoplastic constitutive model
Arunabha M. Roy, Suman Guha, Veera Sundararaghavan, et al.
Journal of the Mechanics and Physics of Solids (2024) Vol. 185, pp. 105570-105570
Closed Access | Times Cited: 8
Arunabha M. Roy, Suman Guha, Veera Sundararaghavan, et al.
Journal of the Mechanics and Physics of Solids (2024) Vol. 185, pp. 105570-105570
Closed Access | Times Cited: 8
Aircraft Structural Design and Life-Cycle Assessment through Digital Twins
Sérgio M. O. Tavares, João PA Ribeiro, Bruno A. Ribeiro, et al.
Designs (2024) Vol. 8, Iss. 2, pp. 29-29
Open Access | Times Cited: 8
Sérgio M. O. Tavares, João PA Ribeiro, Bruno A. Ribeiro, et al.
Designs (2024) Vol. 8, Iss. 2, pp. 29-29
Open Access | Times Cited: 8
A hierarchically normalized physics-informed neural network for solving differential equations: Application for solid mechanics problems
Thang Le-Duc, Seunghye Lee, H. Nguyen‐Xuan, et al.
Engineering Applications of Artificial Intelligence (2024) Vol. 133, pp. 108400-108400
Closed Access | Times Cited: 8
Thang Le-Duc, Seunghye Lee, H. Nguyen‐Xuan, et al.
Engineering Applications of Artificial Intelligence (2024) Vol. 133, pp. 108400-108400
Closed Access | Times Cited: 8
Adaptive Deep Fourier Residual method via overlapping domain decomposition
Jamie M. Taylor, Manuela Bastidas, Victor M. Calo, et al.
Computer Methods in Applied Mechanics and Engineering (2024) Vol. 427, pp. 116997-116997
Open Access | Times Cited: 8
Jamie M. Taylor, Manuela Bastidas, Victor M. Calo, et al.
Computer Methods in Applied Mechanics and Engineering (2024) Vol. 427, pp. 116997-116997
Open Access | Times Cited: 8
Phase-field modeling of fracture with physics-informed deep learning
M. Manav, Roberto Molinaro, Siddhartha Mishra, et al.
Computer Methods in Applied Mechanics and Engineering (2024) Vol. 429, pp. 117104-117104
Open Access | Times Cited: 8
M. Manav, Roberto Molinaro, Siddhartha Mishra, et al.
Computer Methods in Applied Mechanics and Engineering (2024) Vol. 429, pp. 117104-117104
Open Access | Times Cited: 8
Integrated Finite Element Neural Network (I-FENN) for non-local continuum damage mechanics
Panos Pantidis, Mostafa E. Mobasher
Computer Methods in Applied Mechanics and Engineering (2022) Vol. 404, pp. 115766-115766
Closed Access | Times Cited: 35
Panos Pantidis, Mostafa E. Mobasher
Computer Methods in Applied Mechanics and Engineering (2022) Vol. 404, pp. 115766-115766
Closed Access | Times Cited: 35
AT-PINN: Advanced time-marching physics-informed neural network for structural vibration analysis
Zhaolin Chen, S.K. Lai, Zhichun Yang
Thin-Walled Structures (2023) Vol. 196, pp. 111423-111423
Closed Access | Times Cited: 21
Zhaolin Chen, S.K. Lai, Zhichun Yang
Thin-Walled Structures (2023) Vol. 196, pp. 111423-111423
Closed Access | Times Cited: 21
A comparative investigation of a time‐dependent mesh method and physics‐informed neural networks to analyze the generalized Kolmogorov–Petrovsky–Piskunov equation
Saad Sultan, Zhengce Zhang
International Journal for Numerical Methods in Fluids (2024) Vol. 96, Iss. 5, pp. 651-669
Closed Access | Times Cited: 5
Saad Sultan, Zhengce Zhang
International Journal for Numerical Methods in Fluids (2024) Vol. 96, Iss. 5, pp. 651-669
Closed Access | Times Cited: 5
Seismic Wave Propagation and Inversion with Neural Operators
Yan Yang, Angela F. Gao, Jorge C. Castellanos, et al.
The Seismic Record (2021) Vol. 1, Iss. 3, pp. 126-134
Open Access | Times Cited: 39
Yan Yang, Angela F. Gao, Jorge C. Castellanos, et al.
The Seismic Record (2021) Vol. 1, Iss. 3, pp. 126-134
Open Access | Times Cited: 39
Learning Specialized Activation Functions for Physics-Informed Neural Networks
Han Wang, Lu Lu, Shiji Song, et al.
Communications in Computational Physics (2023) Vol. 34, Iss. 4, pp. 869-906
Open Access | Times Cited: 13
Han Wang, Lu Lu, Shiji Song, et al.
Communications in Computational Physics (2023) Vol. 34, Iss. 4, pp. 869-906
Open Access | Times Cited: 13
Unified finite-volume physics informed neural networks to solve the heterogeneous partial differential equations
Di Mei, Kangcheng Zhou, Chun‐Ho Liu
Knowledge-Based Systems (2024) Vol. 295, pp. 111831-111831
Closed Access | Times Cited: 5
Di Mei, Kangcheng Zhou, Chun‐Ho Liu
Knowledge-Based Systems (2024) Vol. 295, pp. 111831-111831
Closed Access | Times Cited: 5
Physics-Informed Machine Learning of Argon Gas-Driven Melt Pool Dynamics
Rahul Sharma, Y. B. Guo, Maziar Raissi, et al.
Journal of Manufacturing Science and Engineering (2024) Vol. 146, Iss. 8
Open Access | Times Cited: 5
Rahul Sharma, Y. B. Guo, Maziar Raissi, et al.
Journal of Manufacturing Science and Engineering (2024) Vol. 146, Iss. 8
Open Access | Times Cited: 5