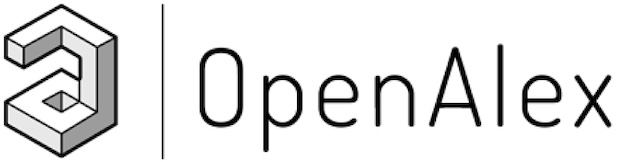
OpenAlex is a bibliographic catalogue of scientific papers, authors and institutions accessible in open access mode, named after the Library of Alexandria. It's citation coverage is excellent and I hope you will find utility in this listing of citing articles!
If you click the article title, you'll navigate to the article, as listed in CrossRef. If you click the Open Access links, you'll navigate to the "best Open Access location". Clicking the citation count will open this listing for that article. Lastly at the bottom of the page, you'll find basic pagination options.
Requested Article:
Learning a Formula of Interpretability to Learn Interpretable Formulas
Marco Virgolin, Andrea De Lorenzo, Eric Medvet, et al.
Lecture notes in computer science (2020), pp. 79-93
Closed Access | Times Cited: 20
Marco Virgolin, Andrea De Lorenzo, Eric Medvet, et al.
Lecture notes in computer science (2020), pp. 79-93
Closed Access | Times Cited: 20
Showing 20 citing articles:
Explainable Artificial Intelligence by Genetic Programming: A Survey
Yi Mei, Qi Chen, Andrew Lensen, et al.
IEEE Transactions on Evolutionary Computation (2022) Vol. 27, Iss. 3, pp. 621-641
Closed Access | Times Cited: 72
Yi Mei, Qi Chen, Andrew Lensen, et al.
IEEE Transactions on Evolutionary Computation (2022) Vol. 27, Iss. 3, pp. 621-641
Closed Access | Times Cited: 72
Contemporary Symbolic Regression Methods and their Relative Performance
William La Cava, Bogdan Burlacu, Marco Virgolin, et al.
arXiv (Cornell University) (2021)
Open Access | Times Cited: 71
William La Cava, Bogdan Burlacu, Marco Virgolin, et al.
arXiv (Cornell University) (2021)
Open Access | Times Cited: 71
Evolutionary Learning of Interpretable Decision Trees
Leonardo Lucio Custode, Giovanni Iacca
IEEE Access (2023) Vol. 11, pp. 6169-6184
Open Access | Times Cited: 34
Leonardo Lucio Custode, Giovanni Iacca
IEEE Access (2023) Vol. 11, pp. 6169-6184
Open Access | Times Cited: 34
Naturally Interpretable Control Policies via Graph-Based Genetic Programming
Giorgia Nadizar, Eric Medvet, Dennis G. Wilson
Lecture notes in computer science (2024), pp. 73-89
Closed Access | Times Cited: 9
Giorgia Nadizar, Eric Medvet, Dennis G. Wilson
Lecture notes in computer science (2024), pp. 73-89
Closed Access | Times Cited: 9
Simplification of genetic programs: a literature survey
Noman Javed, Fernand Gobet, Peter C. R. Lane
Data Mining and Knowledge Discovery (2022) Vol. 36, Iss. 4, pp. 1279-1300
Open Access | Times Cited: 16
Noman Javed, Fernand Gobet, Peter C. R. Lane
Data Mining and Knowledge Discovery (2022) Vol. 36, Iss. 4, pp. 1279-1300
Open Access | Times Cited: 16
An Analysis of the Ingredients for Learning Interpretable Symbolic Regression Models with Human-in-the-loop and Genetic Programming
Giorgia Nadizar, Luigi Rovito, Andrea De Lorenzo, et al.
ACM Transactions on Evolutionary Learning and Optimization (2024) Vol. 4, Iss. 1, pp. 1-30
Closed Access | Times Cited: 3
Giorgia Nadizar, Luigi Rovito, Andrea De Lorenzo, et al.
ACM Transactions on Evolutionary Learning and Optimization (2024) Vol. 4, Iss. 1, pp. 1-30
Closed Access | Times Cited: 3
Model learning with personalized interpretability estimation (ML-PIE)
Marco Virgolin, Andrea De Lorenzo, Francesca Randone, et al.
Proceedings of the Genetic and Evolutionary Computation Conference Companion (2021), pp. 1355-1364
Open Access | Times Cited: 14
Marco Virgolin, Andrea De Lorenzo, Francesca Randone, et al.
Proceedings of the Genetic and Evolutionary Computation Conference Companion (2021), pp. 1355-1364
Open Access | Times Cited: 14
Evolvability degeneration in multi-objective genetic programming for symbolic regression
Dazhuang Liu, Marco Virgolin, Tanja Alderliesten, et al.
Proceedings of the Genetic and Evolutionary Computation Conference (2022)
Open Access | Times Cited: 10
Dazhuang Liu, Marco Virgolin, Tanja Alderliesten, et al.
Proceedings of the Genetic and Evolutionary Computation Conference (2022)
Open Access | Times Cited: 10
Genetic programming is naturally suited to evolve bagging ensembles
Marco Virgolin
Proceedings of the Genetic and Evolutionary Computation Conference (2021), pp. 830-839
Open Access | Times Cited: 11
Marco Virgolin
Proceedings of the Genetic and Evolutionary Computation Conference (2021), pp. 830-839
Open Access | Times Cited: 11
Mining Road Traffic Rules with Signal Temporal Logic and Grammar-Based Genetic Programming
Federico Pigozzi, Eric Medvet, Laura Nenzi
Applied Sciences (2021) Vol. 11, Iss. 22, pp. 10573-10573
Open Access | Times Cited: 11
Federico Pigozzi, Eric Medvet, Laura Nenzi
Applied Sciences (2021) Vol. 11, Iss. 22, pp. 10573-10573
Open Access | Times Cited: 11
A co-evolutionary approach to interpretable reinforcement learning in environments with continuous action spaces
Leonardo Lucio Custode, Giovanni Iacca
2021 IEEE Symposium Series on Computational Intelligence (SSCI) (2021), pp. 1-8
Closed Access | Times Cited: 10
Leonardo Lucio Custode, Giovanni Iacca
2021 IEEE Symposium Series on Computational Intelligence (SSCI) (2021), pp. 1-8
Closed Access | Times Cited: 10
Quality–diversity optimization of decision trees for interpretable reinforcement learning
Andrea Ferigo, Leonardo Lucio Custode, Giovanni Iacca
Neural Computing and Applications (2023)
Open Access | Times Cited: 2
Andrea Ferigo, Leonardo Lucio Custode, Giovanni Iacca
Neural Computing and Applications (2023)
Open Access | Times Cited: 2
GP-DMD: a genetic programming variant with dynamic management of diversity
Ricardo Nieto-Fuentes, Carlos Segura
Genetic Programming and Evolvable Machines (2022) Vol. 23, Iss. 2, pp. 279-304
Closed Access | Times Cited: 3
Ricardo Nieto-Fuentes, Carlos Segura
Genetic Programming and Evolvable Machines (2022) Vol. 23, Iss. 2, pp. 279-304
Closed Access | Times Cited: 3
Decomposition based cross-parallel multiobjective genetic programming for symbolic regression
Lei Fan, Zhaobing Su, Xiyang Liu, et al.
Applied Soft Computing (2024) Vol. 167, pp. 112239-112239
Closed Access
Lei Fan, Zhaobing Su, Xiyang Liu, et al.
Applied Soft Computing (2024) Vol. 167, pp. 112239-112239
Closed Access
Model Learning with Personalized Interpretability Estimation (ML-PIE)
Marco Virgolin, Andrea De Lorenzo, Francesca Randone, et al.
arXiv (Cornell University) (2021)
Closed Access | Times Cited: 2
Marco Virgolin, Andrea De Lorenzo, Francesca Randone, et al.
arXiv (Cornell University) (2021)
Closed Access | Times Cited: 2
Evolutionary learning of interpretable decision trees
Leonardo Lucio Custode, Giovanni Iacca
arXiv (Cornell University) (2020)
Closed Access | Times Cited: 1
Leonardo Lucio Custode, Giovanni Iacca
arXiv (Cornell University) (2020)
Closed Access | Times Cited: 1
Genetic Programming is Naturally Suited to Evolve Bagging Ensembles
Marco Virgolin
arXiv (Cornell University) (2020)
Closed Access | Times Cited: 1
Marco Virgolin
arXiv (Cornell University) (2020)
Closed Access | Times Cited: 1
Towards Interpretable Policies in Multi-agent Reinforcement Learning Tasks
Marco Crespi, Leonardo Lucio Custode, Giovanni Iacca
Lecture notes in computer science (2022), pp. 262-276
Closed Access | Times Cited: 1
Marco Crespi, Leonardo Lucio Custode, Giovanni Iacca
Lecture notes in computer science (2022), pp. 262-276
Closed Access | Times Cited: 1
Simple Simultaneous Ensemble Learning in Genetic Programming.
Marco Virgolin
arXiv (Cornell University) (2020)
Closed Access
Marco Virgolin
arXiv (Cornell University) (2020)
Closed Access
Gene-pool Optimal Mixing in Cartesian Genetic Programming
Joe Harrison, Tanja Alderliesten, Peter A. N. Bosman
Lecture notes in computer science (2022), pp. 19-32
Closed Access
Joe Harrison, Tanja Alderliesten, Peter A. N. Bosman
Lecture notes in computer science (2022), pp. 19-32
Closed Access