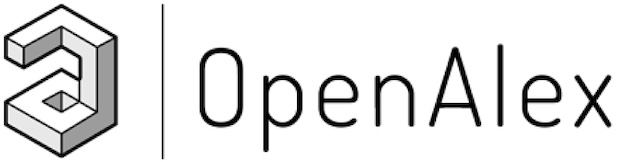
OpenAlex is a bibliographic catalogue of scientific papers, authors and institutions accessible in open access mode, named after the Library of Alexandria. It's citation coverage is excellent and I hope you will find utility in this listing of citing articles!
If you click the article title, you'll navigate to the article, as listed in CrossRef. If you click the Open Access links, you'll navigate to the "best Open Access location". Clicking the citation count will open this listing for that article. Lastly at the bottom of the page, you'll find basic pagination options.
Requested Article:
Differentiation Between Benign and Nonbenign Meningiomas by Using Texture Analysis From Multiparametric MRI
Chao Ke, Haolin Chen, Xiaofei Lv, et al.
Journal of Magnetic Resonance Imaging (2019) Vol. 51, Iss. 6, pp. 1810-1820
Closed Access | Times Cited: 38
Chao Ke, Haolin Chen, Xiaofei Lv, et al.
Journal of Magnetic Resonance Imaging (2019) Vol. 51, Iss. 6, pp. 1810-1820
Closed Access | Times Cited: 38
Showing 1-25 of 38 citing articles:
Meningioma MRI radiomics and machine learning: systematic review, quality score assessment, and meta-analysis
Lorenzo Ugga, Teresa Perillo, Renato Cuocolo, et al.
Neuroradiology (2021) Vol. 63, Iss. 8, pp. 1293-1304
Open Access | Times Cited: 65
Lorenzo Ugga, Teresa Perillo, Renato Cuocolo, et al.
Neuroradiology (2021) Vol. 63, Iss. 8, pp. 1293-1304
Open Access | Times Cited: 65
Machine learning-based radiomics analysis in predicting the meningioma grade using multiparametric MRI
Jianping Hu, Yijing Zhao, Mengcheng Li, et al.
European Journal of Radiology (2020) Vol. 131, pp. 109251-109251
Closed Access | Times Cited: 53
Jianping Hu, Yijing Zhao, Mengcheng Li, et al.
European Journal of Radiology (2020) Vol. 131, pp. 109251-109251
Closed Access | Times Cited: 53
A deep learning radiomics model may help to improve the prediction performance of preoperative grading in meningioma
Liping Yang, Panpan Xu, Ying Zhang, et al.
Neuroradiology (2022) Vol. 64, Iss. 7, pp. 1373-1382
Closed Access | Times Cited: 33
Liping Yang, Panpan Xu, Ying Zhang, et al.
Neuroradiology (2022) Vol. 64, Iss. 7, pp. 1373-1382
Closed Access | Times Cited: 33
A Spotlight on the Role of Radiomics and Machine-Learning Applications in the Management of Intracranial Meningiomas: A New Perspective in Neuro-Oncology: A Review
Lara Brunasso, Gianluca Ferini, Lapo Bonosi, et al.
Life (2022) Vol. 12, Iss. 4, pp. 586-586
Open Access | Times Cited: 27
Lara Brunasso, Gianluca Ferini, Lapo Bonosi, et al.
Life (2022) Vol. 12, Iss. 4, pp. 586-586
Open Access | Times Cited: 27
Deep learning–based automatic segmentation of meningioma from multiparametric MRI for preoperative meningioma differentiation using radiomic features: a multicentre study
Haolin Chen, Shuqi Li, Youming Zhang, et al.
European Radiology (2022) Vol. 32, Iss. 10, pp. 7248-7259
Closed Access | Times Cited: 23
Haolin Chen, Shuqi Li, Youming Zhang, et al.
European Radiology (2022) Vol. 32, Iss. 10, pp. 7248-7259
Closed Access | Times Cited: 23
Research Progress of Artificial Intelligence in the Grading and Classification of Meningiomas
Yuan Gui, Jing Zhang
Academic Radiology (2024) Vol. 31, Iss. 8, pp. 3346-3354
Closed Access | Times Cited: 4
Yuan Gui, Jing Zhang
Academic Radiology (2024) Vol. 31, Iss. 8, pp. 3346-3354
Closed Access | Times Cited: 4
Deep learning–based automatic segmentation of meningioma from T1-weighted contrast-enhanced MRI for preoperative meningioma differentiation using radiomic features
Liping Yang, Tianzuo Wang, Jinling Zhang, et al.
BMC Medical Imaging (2024) Vol. 24, Iss. 1
Open Access | Times Cited: 4
Liping Yang, Tianzuo Wang, Jinling Zhang, et al.
BMC Medical Imaging (2024) Vol. 24, Iss. 1
Open Access | Times Cited: 4
Quality assessment of meningioma radiomics studies: Bridging the gap between exploratory research and clinical applications
So Yeon Won, Yae Won Park, Sung Soo Ahn, et al.
European Journal of Radiology (2021) Vol. 138, pp. 109673-109673
Closed Access | Times Cited: 24
So Yeon Won, Yae Won Park, Sung Soo Ahn, et al.
European Journal of Radiology (2021) Vol. 138, pp. 109673-109673
Closed Access | Times Cited: 24
Use of advanced neuroimaging and artificial intelligence in meningiomas
Norbert Galldiks, Frank Angenstein, Jan‐Michael Werner, et al.
Brain Pathology (2022) Vol. 32, Iss. 2
Open Access | Times Cited: 16
Norbert Galldiks, Frank Angenstein, Jan‐Michael Werner, et al.
Brain Pathology (2022) Vol. 32, Iss. 2
Open Access | Times Cited: 16
Machine Learning for the Detection and Segmentation of Benign Tumors of the Central Nervous System: A Systematic Review
Paul Windisch, Carole Koechli, Susanne Rogers, et al.
Cancers (2022) Vol. 14, Iss. 11, pp. 2676-2676
Open Access | Times Cited: 15
Paul Windisch, Carole Koechli, Susanne Rogers, et al.
Cancers (2022) Vol. 14, Iss. 11, pp. 2676-2676
Open Access | Times Cited: 15
A radiomics nomogram for predicting the meningioma grade based on enhanced T1WI images
Chongfeng Duan, Xiaoming Zhou, Jiachen Wang, et al.
British Journal of Radiology (2022) Vol. 95, Iss. 1137
Open Access | Times Cited: 14
Chongfeng Duan, Xiaoming Zhou, Jiachen Wang, et al.
British Journal of Radiology (2022) Vol. 95, Iss. 1137
Open Access | Times Cited: 14
An MRI-Based Deep Transfer Learning Radiomics Nomogram to Predict Ki-67 Proliferation Index of Meningioma
Chongfeng Duan, Dapeng Hao, Jiufa Cui, et al.
Deleted Journal (2024) Vol. 37, Iss. 2, pp. 510-519
Open Access | Times Cited: 2
Chongfeng Duan, Dapeng Hao, Jiufa Cui, et al.
Deleted Journal (2024) Vol. 37, Iss. 2, pp. 510-519
Open Access | Times Cited: 2
Deep learning and radiomics-based approach to meningioma grading: exploring the potential value of peritumoral edema regions
Zhuo Zhang, Ying Miao, Jixuan Wu, et al.
Physics in Medicine and Biology (2024) Vol. 69, Iss. 10, pp. 105002-105002
Closed Access | Times Cited: 2
Zhuo Zhang, Ying Miao, Jixuan Wu, et al.
Physics in Medicine and Biology (2024) Vol. 69, Iss. 10, pp. 105002-105002
Closed Access | Times Cited: 2
PDSMNet: Parallel pyramid dual-stream modeling for automatic lung COVID-19 infection segmentations
Ichiro Nakamoto, Weiqing Zhuang, Hua Chen, et al.
Engineering Applications of Artificial Intelligence (2024) Vol. 133, pp. 108541-108541
Closed Access | Times Cited: 2
Ichiro Nakamoto, Weiqing Zhuang, Hua Chen, et al.
Engineering Applications of Artificial Intelligence (2024) Vol. 133, pp. 108541-108541
Closed Access | Times Cited: 2
Cycle-consistent adversarial networks improves generalizability of radiomics model in grading meningiomas on external validation
Yae Won Park, Seo Jeong Shin, Jihwan Eom, et al.
Scientific Reports (2022) Vol. 12, Iss. 1
Open Access | Times Cited: 12
Yae Won Park, Seo Jeong Shin, Jihwan Eom, et al.
Scientific Reports (2022) Vol. 12, Iss. 1
Open Access | Times Cited: 12
Comparison of different radiomic models based on enhanced T1-weighted images to predict the meningioma grade
Chongfeng Duan, N Li, Y Li, et al.
Clinical Radiology (2022) Vol. 77, Iss. 4, pp. e302-e307
Closed Access | Times Cited: 11
Chongfeng Duan, N Li, Y Li, et al.
Clinical Radiology (2022) Vol. 77, Iss. 4, pp. e302-e307
Closed Access | Times Cited: 11
Performance comparison of 2D and 3D MRI radiomics features in meningioma grade prediction: A preliminary study
Chongfeng Duan, Nan Li, Xuejun Liu, et al.
Frontiers in Oncology (2023) Vol. 13
Open Access | Times Cited: 6
Chongfeng Duan, Nan Li, Xuejun Liu, et al.
Frontiers in Oncology (2023) Vol. 13
Open Access | Times Cited: 6
Lesion delineation framework for vestibular schwannoma, meningioma and brain metastasis for gamma knife radiosurgery using stereotactic magnetic resonance images
Wei‐Kai Lee, Huai‐Che Yang, Cheng‐Chia Lee, et al.
Computer Methods and Programs in Biomedicine (2022) Vol. 229, pp. 107311-107311
Closed Access | Times Cited: 9
Wei‐Kai Lee, Huai‐Che Yang, Cheng‐Chia Lee, et al.
Computer Methods and Programs in Biomedicine (2022) Vol. 229, pp. 107311-107311
Closed Access | Times Cited: 9
Advanced Meningioma Imaging
Erik K. Loken, Raymond Y. Huang
Neurosurgery Clinics of North America (2023) Vol. 34, Iss. 3, pp. 335-345
Closed Access | Times Cited: 5
Erik K. Loken, Raymond Y. Huang
Neurosurgery Clinics of North America (2023) Vol. 34, Iss. 3, pp. 335-345
Closed Access | Times Cited: 5
Comparative Analysis of the MRI Characteristics of Meningiomas According to the 2016 WHO Pathological Classification
Juan Yu, Fanfan Chen, Hanwen Zhang, et al.
Technology in Cancer Research & Treatment (2020) Vol. 19
Open Access | Times Cited: 14
Juan Yu, Fanfan Chen, Hanwen Zhang, et al.
Technology in Cancer Research & Treatment (2020) Vol. 19
Open Access | Times Cited: 14
Traditional Machine Learning Methods versus Deep Learning for Meningioma Classification, Grading, Outcome Prediction, and Segmentation: A Systematic Review and Meta-Analysis
Krish Maniar, Philipp Lassarén, Aakanksha Rana, et al.
World Neurosurgery (2023) Vol. 179, pp. e119-e134
Closed Access | Times Cited: 4
Krish Maniar, Philipp Lassarén, Aakanksha Rana, et al.
World Neurosurgery (2023) Vol. 179, pp. e119-e134
Closed Access | Times Cited: 4
Prediction of progesterone receptor expression in high-grade meningioma by using radiomics based on enhanced T1WI
Chunyan Duan, N Li, Y Li, et al.
Clinical Radiology (2023) Vol. 78, Iss. 10, pp. e752-e757
Closed Access | Times Cited: 3
Chunyan Duan, N Li, Y Li, et al.
Clinical Radiology (2023) Vol. 78, Iss. 10, pp. e752-e757
Closed Access | Times Cited: 3
A Predictive Nomogram for Atypical Meningioma Based On Preoperative Magnetic Resonance Imaging and Routine Blood Tests
Yaobin Lin, Penggao Dai, Lin Qi, et al.
World Neurosurgery (2022) Vol. 163, pp. e610-e616
Closed Access | Times Cited: 5
Yaobin Lin, Penggao Dai, Lin Qi, et al.
World Neurosurgery (2022) Vol. 163, pp. e610-e616
Closed Access | Times Cited: 5
Base MRI Imaging Characteristics of Meningioma Patients to Discuss the WHO Classification of Brain Invasion Otherwise Benign Meningiomas
Xudan Luo, Hong Jiang, XJ Liu, et al.
Technology in Cancer Research & Treatment (2023) Vol. 22
Open Access | Times Cited: 2
Xudan Luo, Hong Jiang, XJ Liu, et al.
Technology in Cancer Research & Treatment (2023) Vol. 22
Open Access | Times Cited: 2
Preoperative Prediction of Meningioma Subtype by Constructing a Clinical-Radiomics Model Nomogram Based on Magnetic Resonance Imaging
Tao Han, Xianwang Liu, Zhendong Xu, et al.
World Neurosurgery (2023) Vol. 181, pp. e203-e213
Closed Access | Times Cited: 2
Tao Han, Xianwang Liu, Zhendong Xu, et al.
World Neurosurgery (2023) Vol. 181, pp. e203-e213
Closed Access | Times Cited: 2