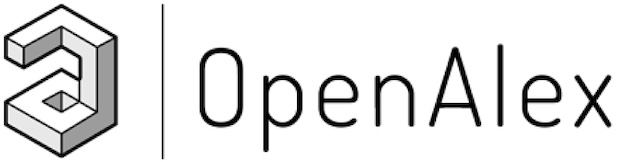
OpenAlex is a bibliographic catalogue of scientific papers, authors and institutions accessible in open access mode, named after the Library of Alexandria. It's citation coverage is excellent and I hope you will find utility in this listing of citing articles!
If you click the article title, you'll navigate to the article, as listed in CrossRef. If you click the Open Access links, you'll navigate to the "best Open Access location". Clicking the citation count will open this listing for that article. Lastly at the bottom of the page, you'll find basic pagination options.
Requested Article:
Applying artificial neural networks to predict the enhanced thermal conductivity of a phase change material with dispersed oxide nanoparticles
Sadegh Motahar, Saeb Sadri
International Journal of Energy Research (2021) Vol. 45, Iss. 10, pp. 15092-15109
Open Access | Times Cited: 20
Sadegh Motahar, Saeb Sadri
International Journal of Energy Research (2021) Vol. 45, Iss. 10, pp. 15092-15109
Open Access | Times Cited: 20
Showing 20 citing articles:
Thermophysical properties prediction of carbon-based nano-enhanced phase change material's using various machine learning methods
Yuguo Gao, Ihab Shigidi, Masood Ashraf Ali, et al.
Journal of the Taiwan Institute of Chemical Engineers (2023) Vol. 148, pp. 104662-104662
Closed Access | Times Cited: 31
Yuguo Gao, Ihab Shigidi, Masood Ashraf Ali, et al.
Journal of the Taiwan Institute of Chemical Engineers (2023) Vol. 148, pp. 104662-104662
Closed Access | Times Cited: 31
A timeline of the phase-change problem for latent thermal energy storage systems: A review of theoretical approaches from the 1970′s to 2022
Thiago Torres Martins Rocha, Paulo V. Trevizoli, Raphael Nunes de Oliveira
Solar Energy (2023) Vol. 250, pp. 248-284
Closed Access | Times Cited: 28
Thiago Torres Martins Rocha, Paulo V. Trevizoli, Raphael Nunes de Oliveira
Solar Energy (2023) Vol. 250, pp. 248-284
Closed Access | Times Cited: 28
Application of an artificial intelligence model for natural convection of nano-encapsulated phase change materials (NEPCMs) confined in a porous square enclosure with an inclined elliptical heated block
Rachid Hidki, Lahcen El Moutaouakil, Mohammed Boukendil, et al.
International Communications in Heat and Mass Transfer (2024) Vol. 155, pp. 107546-107546
Closed Access | Times Cited: 8
Rachid Hidki, Lahcen El Moutaouakil, Mohammed Boukendil, et al.
International Communications in Heat and Mass Transfer (2024) Vol. 155, pp. 107546-107546
Closed Access | Times Cited: 8
Experimental Investigation and Artificial Neural Network-Based Prediction of Thermal Conductivity of Metal Oxide-Enhanced Organic Phase-Change Materials
Man Mohan, Sheetal Kumar Dewangan, K. Raja Rao, et al.
International Journal of Energy Research (2024) Vol. 2024, pp. 1-18
Open Access | Times Cited: 7
Man Mohan, Sheetal Kumar Dewangan, K. Raja Rao, et al.
International Journal of Energy Research (2024) Vol. 2024, pp. 1-18
Open Access | Times Cited: 7
Thermal conductivity prediction of nano enhanced phase change materials: A comparative machine learning approach
Farzad Jaliliantabar
Journal of Energy Storage (2021) Vol. 46, pp. 103633-103633
Closed Access | Times Cited: 34
Farzad Jaliliantabar
Journal of Energy Storage (2021) Vol. 46, pp. 103633-103633
Closed Access | Times Cited: 34
A highly accurate model for prediction of thermal conductivity of carbon-based nano-enhanced PCMs using an artificial neural network
Mojtaba Taheri, Fathollah Pourfayaz, Sara Hemmati
Energy Reports (2023) Vol. 10, pp. 1249-1258
Open Access | Times Cited: 13
Mojtaba Taheri, Fathollah Pourfayaz, Sara Hemmati
Energy Reports (2023) Vol. 10, pp. 1249-1258
Open Access | Times Cited: 13
Investigating the Relationship between Soil Gas Radon and Soil Permeability by Using Artificial Neural Networks
Selin Erzin
Deu Muhendislik Fakultesi Fen ve Muhendislik (2025) Vol. 27, Iss. 79, pp. 147-151
Closed Access
Selin Erzin
Deu Muhendislik Fakultesi Fen ve Muhendislik (2025) Vol. 27, Iss. 79, pp. 147-151
Closed Access
A review of artificial intelligence to thermal energy storage and heat transfer improvement in phase change materials
Artur Nemś, Sindu Daniarta, Magdalena Nemś, et al.
Sustainable materials and technologies (2025), pp. e01348-e01348
Closed Access
Artur Nemś, Sindu Daniarta, Magdalena Nemś, et al.
Sustainable materials and technologies (2025), pp. e01348-e01348
Closed Access
Predicting thermophysical properties enhancement of metal-based phase change materials using various machine learning algorithms
Mohsen Bakouri, Hakim S. Sultan Aljibori, Sarminah Samad, et al.
Journal of the Taiwan Institute of Chemical Engineers (2023) Vol. 148, pp. 104934-104934
Closed Access | Times Cited: 12
Mohsen Bakouri, Hakim S. Sultan Aljibori, Sarminah Samad, et al.
Journal of the Taiwan Institute of Chemical Engineers (2023) Vol. 148, pp. 104934-104934
Closed Access | Times Cited: 12
The Contribution of Artificial Intelligence to Phase Change Materials in Thermal Energy Storage: From Prediction to Optimization
Shuli Liu, Junrui Han, Yongliang Shen, et al.
Renewable Energy (2024), pp. 121973-121973
Closed Access | Times Cited: 4
Shuli Liu, Junrui Han, Yongliang Shen, et al.
Renewable Energy (2024), pp. 121973-121973
Closed Access | Times Cited: 4
A machine learning approach to predicting thermal conductivity in nanoparticle-enhanced phase change materials
Abir Msalmi, Fathi Djemal, Abdelkhalak El Hami, et al.
Proceedings of the Institution of Mechanical Engineers Part C Journal of Mechanical Engineering Science (2025)
Closed Access
Abir Msalmi, Fathi Djemal, Abdelkhalak El Hami, et al.
Proceedings of the Institution of Mechanical Engineers Part C Journal of Mechanical Engineering Science (2025)
Closed Access
Prediction of the radon concentration in thermal waters using artificial neural networks
Selin Erzin
International Journal of Environmental Science and Technology (2024) Vol. 21, Iss. 10, pp. 7321-7328
Closed Access | Times Cited: 3
Selin Erzin
International Journal of Environmental Science and Technology (2024) Vol. 21, Iss. 10, pp. 7321-7328
Closed Access | Times Cited: 3
Optimization of Thermal Conductivity and Latent Heat Capacity Using Fractional Factorial Approach for the Synthesis of Nano‐Enhanced High‐Performance Phase‐Change Material
Man Mohan, Sheetal Kumar Dewangan, Kwan Lee, et al.
International Journal of Energy Research (2024) Vol. 2024, Iss. 1
Open Access | Times Cited: 3
Man Mohan, Sheetal Kumar Dewangan, Kwan Lee, et al.
International Journal of Energy Research (2024) Vol. 2024, Iss. 1
Open Access | Times Cited: 3
Integrating artificial Intelligence-Based metaheuristic optimization with Machine learning to enhance Nanomaterial-Containing latent heat thermal energy storage systems
Ali Basem, Hanaa Kadhim Abdulaali, As’ad Alizadeh, et al.
Energy Conversion and Management X (2024), pp. 100835-100835
Open Access | Times Cited: 2
Ali Basem, Hanaa Kadhim Abdulaali, As’ad Alizadeh, et al.
Energy Conversion and Management X (2024), pp. 100835-100835
Open Access | Times Cited: 2
A Comprehensive Review of Predicting the Thermophysical Properties of Nanofluids Using Machine Learning Methods
Helin Wang, Xueye Chen
Industrial & Engineering Chemistry Research (2022) Vol. 61, Iss. 40, pp. 14711-14730
Closed Access | Times Cited: 9
Helin Wang, Xueye Chen
Industrial & Engineering Chemistry Research (2022) Vol. 61, Iss. 40, pp. 14711-14730
Closed Access | Times Cited: 9
Investigation of the thermal conductivity of soil subjected to freeze–thaw cycles using the artificial neural network model
Müge Elif ORAKOĞLU FIRAT, Orhan Atıla
Journal of Thermal Analysis and Calorimetry (2021) Vol. 147, Iss. 14, pp. 8077-8093
Closed Access | Times Cited: 11
Müge Elif ORAKOĞLU FIRAT, Orhan Atıla
Journal of Thermal Analysis and Calorimetry (2021) Vol. 147, Iss. 14, pp. 8077-8093
Closed Access | Times Cited: 11
Decanoic acid-palmitic acid/SiO2@TiO2 phase change microcapsules based on RBF model with excellent photocatalysis performance and humidity control property
Hao Zhang, Tianci Gao, Zhifang Zong, et al.
Environmental Science and Pollution Research (2023) Vol. 30, Iss. 18, pp. 51830-51843
Closed Access | Times Cited: 4
Hao Zhang, Tianci Gao, Zhifang Zong, et al.
Environmental Science and Pollution Research (2023) Vol. 30, Iss. 18, pp. 51830-51843
Closed Access | Times Cited: 4
Enhancing thermophysical properties of phase change material via alumina and copper nanoparticles
Mostafa Jafarian, Mónica Delgado, Mahmoud Omid, et al.
International Journal of Energy Research (2021) Vol. 46, Iss. 5, pp. 6594-6612
Open Access | Times Cited: 10
Mostafa Jafarian, Mónica Delgado, Mahmoud Omid, et al.
International Journal of Energy Research (2021) Vol. 46, Iss. 5, pp. 6594-6612
Open Access | Times Cited: 10
Insights into the Estimation of the Enhanced Thermal Conductivity of Phase Change Material-Containing Oxide Nanoparticles using Gaussian Process Regression Method
Tzu-Chia Chen, Hasan Sh. Majdi, Aras Masood Ismael, et al.
International Journal of Chemical Engineering (2022) Vol. 2022, pp. 1-10
Open Access | Times Cited: 5
Tzu-Chia Chen, Hasan Sh. Majdi, Aras Masood Ismael, et al.
International Journal of Chemical Engineering (2022) Vol. 2022, pp. 1-10
Open Access | Times Cited: 5
A neural network approach to estimate non-Newtonian behavior of nanofluid phase change material containing mesoporous silica particles
Sadegh Motahar
International journal of engineering. Transactions B: Applications (2021) Vol. 34, Iss. 8
Open Access | Times Cited: 4
Sadegh Motahar
International journal of engineering. Transactions B: Applications (2021) Vol. 34, Iss. 8
Open Access | Times Cited: 4