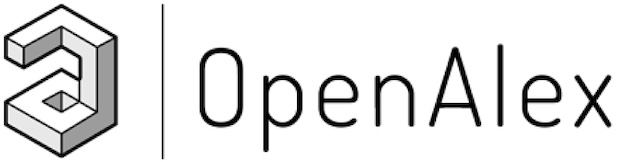
OpenAlex is a bibliographic catalogue of scientific papers, authors and institutions accessible in open access mode, named after the Library of Alexandria. It's citation coverage is excellent and I hope you will find utility in this listing of citing articles!
If you click the article title, you'll navigate to the article, as listed in CrossRef. If you click the Open Access links, you'll navigate to the "best Open Access location". Clicking the citation count will open this listing for that article. Lastly at the bottom of the page, you'll find basic pagination options.
Requested Article:
Multi-step forecasting for wind speed using a modified EMD-based artificial neural network model
Zhenhai Guo, Weigang Zhao, Haiyan Lu, et al.
Renewable Energy (2011) Vol. 37, Iss. 1, pp. 241-249
Closed Access | Times Cited: 501
Zhenhai Guo, Weigang Zhao, Haiyan Lu, et al.
Renewable Energy (2011) Vol. 37, Iss. 1, pp. 241-249
Closed Access | Times Cited: 501
Showing 76-100 of 501 citing articles:
A review of applications of artificial intelligent algorithms in wind farms
Yirui Wang, Yang Yu, Shuyang Cao, et al.
Artificial Intelligence Review (2019) Vol. 53, Iss. 5, pp. 3447-3500
Closed Access | Times Cited: 106
Yirui Wang, Yang Yu, Shuyang Cao, et al.
Artificial Intelligence Review (2019) Vol. 53, Iss. 5, pp. 3447-3500
Closed Access | Times Cited: 106
An adaptive load dispatching and forecasting strategy for a virtual power plant including renewable energy conversion units
Akın Taşçıkaraoğlu, Ozan Erdinç, M. Uzunoglu, et al.
Applied Energy (2014) Vol. 119, pp. 445-453
Closed Access | Times Cited: 105
Akın Taşçıkaraoğlu, Ozan Erdinç, M. Uzunoglu, et al.
Applied Energy (2014) Vol. 119, pp. 445-453
Closed Access | Times Cited: 105
Time series long-term forecasting model based on information granules and fuzzy clustering
Weina Wang, Witold Pedrycz, Xiaodong Liu
Engineering Applications of Artificial Intelligence (2015) Vol. 41, pp. 17-24
Closed Access | Times Cited: 104
Weina Wang, Witold Pedrycz, Xiaodong Liu
Engineering Applications of Artificial Intelligence (2015) Vol. 41, pp. 17-24
Closed Access | Times Cited: 104
A new chaotic time series hybrid prediction method of wind power based on EEMD-SE and full-parameters continued fraction
Cong Wang, Hongli Zhang, Wenhui Fan, et al.
Energy (2017) Vol. 138, pp. 977-990
Closed Access | Times Cited: 103
Cong Wang, Hongli Zhang, Wenhui Fan, et al.
Energy (2017) Vol. 138, pp. 977-990
Closed Access | Times Cited: 103
Prediction interval forecasting of wind speed and wind power using modes decomposition based low rank multi-kernel ridge regression
Jyotirmayee Naik, Ranjeeta Bisoi, P.K. Dash
Renewable Energy (2018) Vol. 129, pp. 357-383
Closed Access | Times Cited: 101
Jyotirmayee Naik, Ranjeeta Bisoi, P.K. Dash
Renewable Energy (2018) Vol. 129, pp. 357-383
Closed Access | Times Cited: 101
A synthesis of feasible control methods for floating offshore wind turbine system dynamics
Kamran Shah, Fantai Meng, Ye Li, et al.
Renewable and Sustainable Energy Reviews (2021) Vol. 151, pp. 111525-111525
Open Access | Times Cited: 100
Kamran Shah, Fantai Meng, Ye Li, et al.
Renewable and Sustainable Energy Reviews (2021) Vol. 151, pp. 111525-111525
Open Access | Times Cited: 100
A novel hybrid deep neural network model for short‐term electricity price forecasting
Chiou‐Jye Huang, Yamin Shen, Yung‐Hsiang Chen, et al.
International Journal of Energy Research (2020) Vol. 45, Iss. 2, pp. 2511-2532
Open Access | Times Cited: 99
Chiou‐Jye Huang, Yamin Shen, Yung‐Hsiang Chen, et al.
International Journal of Energy Research (2020) Vol. 45, Iss. 2, pp. 2511-2532
Open Access | Times Cited: 99
Ultra-short-term wind speed forecasting based on EMD-VAR model and spatial correlation
Zheyong Jiang, Jinxing Che, Lina Wang
Energy Conversion and Management (2021) Vol. 250, pp. 114919-114919
Closed Access | Times Cited: 99
Zheyong Jiang, Jinxing Che, Lina Wang
Energy Conversion and Management (2021) Vol. 250, pp. 114919-114919
Closed Access | Times Cited: 99
An effective secondary decomposition approach for wind power forecasting using extreme learning machine trained by crisscross optimization
Hao Yin, Zhen Dong, Yunlong Chen, et al.
Energy Conversion and Management (2017) Vol. 150, pp. 108-121
Closed Access | Times Cited: 96
Hao Yin, Zhen Dong, Yunlong Chen, et al.
Energy Conversion and Management (2017) Vol. 150, pp. 108-121
Closed Access | Times Cited: 96
Interval forecasting of electricity demand: A novel bivariate EMD-based support vector regression modeling framework
Tao Xiong, Yukun Bao, Zhongyi Hu
International Journal of Electrical Power & Energy Systems (2014) Vol. 63, pp. 353-362
Open Access | Times Cited: 95
Tao Xiong, Yukun Bao, Zhongyi Hu
International Journal of Electrical Power & Energy Systems (2014) Vol. 63, pp. 353-362
Open Access | Times Cited: 95
A self-adaptive hybrid approach for wind speed forecasting
Jianzhou Wang, Jianming Hu, Kailiang Ma, et al.
Renewable Energy (2015) Vol. 78, pp. 374-385
Closed Access | Times Cited: 93
Jianzhou Wang, Jianming Hu, Kailiang Ma, et al.
Renewable Energy (2015) Vol. 78, pp. 374-385
Closed Access | Times Cited: 93
Short-term wind speed forecasting approach using Ensemble Empirical Mode Decomposition and Deep Boltzmann Machine
Santhosh Madasthu, Chintham Venkaiah, D. M. Vinod Kumar
Sustainable Energy Grids and Networks (2019) Vol. 19, pp. 100242-100242
Closed Access | Times Cited: 92
Santhosh Madasthu, Chintham Venkaiah, D. M. Vinod Kumar
Sustainable Energy Grids and Networks (2019) Vol. 19, pp. 100242-100242
Closed Access | Times Cited: 92
A Short-Term Wind Speed Forecasting Model by Using Artificial Neural Networks with Stochastic Optimization for Renewable Energy Systems
Chiou‐Jye Huang, Ping‐Huan Kuo
Energies (2018) Vol. 11, Iss. 10, pp. 2777-2777
Open Access | Times Cited: 91
Chiou‐Jye Huang, Ping‐Huan Kuo
Energies (2018) Vol. 11, Iss. 10, pp. 2777-2777
Open Access | Times Cited: 91
Hybrid deep learning and empirical mode decomposition model for time series applications
Haofan Yang, Yi‐Ping Phoebe Chen
Expert Systems with Applications (2018) Vol. 120, pp. 128-138
Closed Access | Times Cited: 90
Haofan Yang, Yi‐Ping Phoebe Chen
Expert Systems with Applications (2018) Vol. 120, pp. 128-138
Closed Access | Times Cited: 90
A cascaded deep learning wind power prediction approach based on a two-layer of mode decomposition
Hao Yin, Zuhong Ou, Shengquan Huang, et al.
Energy (2019) Vol. 189, pp. 116316-116316
Closed Access | Times Cited: 90
Hao Yin, Zuhong Ou, Shengquan Huang, et al.
Energy (2019) Vol. 189, pp. 116316-116316
Closed Access | Times Cited: 90
An analysis-forecast system for uncertainty modeling of wind speed: A case study of large-scale wind farms
Jianzhou Wang, Tong Niu, Haiyan Lu, et al.
Applied Energy (2017) Vol. 211, pp. 492-512
Open Access | Times Cited: 88
Jianzhou Wang, Tong Niu, Haiyan Lu, et al.
Applied Energy (2017) Vol. 211, pp. 492-512
Open Access | Times Cited: 88
A hybrid approach for multi-step wind speed forecasting based on two-layer decomposition, improved hybrid DE-HHO optimization and KELM
Wenlong Fu, Kai Zhang, Kai Wang, et al.
Renewable Energy (2020) Vol. 164, pp. 211-229
Closed Access | Times Cited: 86
Wenlong Fu, Kai Zhang, Kai Wang, et al.
Renewable Energy (2020) Vol. 164, pp. 211-229
Closed Access | Times Cited: 86
A novel carbon price prediction model combines the secondary decomposition algorithm and the long short-term memory network
Wei Sun, Chenchen Huang
Energy (2020) Vol. 207, pp. 118294-118294
Closed Access | Times Cited: 85
Wei Sun, Chenchen Huang
Energy (2020) Vol. 207, pp. 118294-118294
Closed Access | Times Cited: 85
Analysis of wind power intermittency based on historical wind power data
Guorui Ren, Jie Wan, Jinfu Liu, et al.
Energy (2018) Vol. 150, pp. 482-492
Closed Access | Times Cited: 83
Guorui Ren, Jie Wan, Jinfu Liu, et al.
Energy (2018) Vol. 150, pp. 482-492
Closed Access | Times Cited: 83
Negative correlation learning-based RELM ensemble model integrated with OVMD for multi-step ahead wind speed forecasting
Peng Tian, Chu Zhang, Jianzhong Zhou, et al.
Renewable Energy (2020) Vol. 156, pp. 804-819
Closed Access | Times Cited: 73
Peng Tian, Chu Zhang, Jianzhong Zhou, et al.
Renewable Energy (2020) Vol. 156, pp. 804-819
Closed Access | Times Cited: 73
Multi-step wind speed forecasting and Hurst analysis using novel hybrid secondary decomposition approach
Cem Emeksiz, Mustafa Tan
Energy (2021) Vol. 238, pp. 121764-121764
Closed Access | Times Cited: 67
Cem Emeksiz, Mustafa Tan
Energy (2021) Vol. 238, pp. 121764-121764
Closed Access | Times Cited: 67
Forecasting carbon prices based on real-time decomposition and causal temporal convolutional networks
Dan Li, Yijun Li, Chaoqun Wang, et al.
Applied Energy (2022) Vol. 331, pp. 120452-120452
Closed Access | Times Cited: 60
Dan Li, Yijun Li, Chaoqun Wang, et al.
Applied Energy (2022) Vol. 331, pp. 120452-120452
Closed Access | Times Cited: 60
Multi-step prediction of photovoltaic power based on two-stage decomposition and BILSTM
Wenshuai Lin, Bin Zhang, Hongyi Li, et al.
Neurocomputing (2022) Vol. 504, pp. 56-67
Closed Access | Times Cited: 51
Wenshuai Lin, Bin Zhang, Hongyi Li, et al.
Neurocomputing (2022) Vol. 504, pp. 56-67
Closed Access | Times Cited: 51
Wind speed estimation using novelty hybrid adaptive estimation model based on decomposition and deep learning methods (ICEEMDAN-CNN)
Cem Emeksiz, Mustafa Tan
Energy (2022) Vol. 249, pp. 123785-123785
Closed Access | Times Cited: 44
Cem Emeksiz, Mustafa Tan
Energy (2022) Vol. 249, pp. 123785-123785
Closed Access | Times Cited: 44
An overview of deterministic and probabilistic forecasting methods of wind energy
Yuying Xie, Chaoshun Li, Mengying Li, et al.
iScience (2022) Vol. 26, Iss. 1, pp. 105804-105804
Open Access | Times Cited: 42
Yuying Xie, Chaoshun Li, Mengying Li, et al.
iScience (2022) Vol. 26, Iss. 1, pp. 105804-105804
Open Access | Times Cited: 42