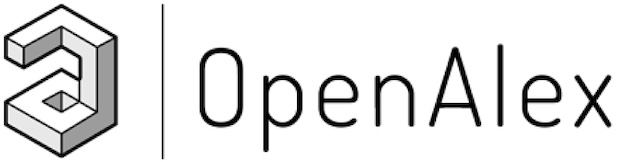
OpenAlex is a bibliographic catalogue of scientific papers, authors and institutions accessible in open access mode, named after the Library of Alexandria. It's citation coverage is excellent and I hope you will find utility in this listing of citing articles!
If you click the article title, you'll navigate to the article, as listed in CrossRef. If you click the Open Access links, you'll navigate to the "best Open Access location". Clicking the citation count will open this listing for that article. Lastly at the bottom of the page, you'll find basic pagination options.
Requested Article:
State-of-the-Art Review of Machine Learning Applications in Constitutive Modeling of Soils
Pin Zhang, Zhen‐Yu Yin, Yin-Fu Jin
Archives of Computational Methods in Engineering (2021) Vol. 28, Iss. 5, pp. 3661-3686
Closed Access | Times Cited: 105
Pin Zhang, Zhen‐Yu Yin, Yin-Fu Jin
Archives of Computational Methods in Engineering (2021) Vol. 28, Iss. 5, pp. 3661-3686
Closed Access | Times Cited: 105
Showing 51-75 of 105 citing articles:
Development of an LSTM-based model for predicting the long-term settlement of land reclamation and a GUI-based tool
Xiaoxuan Chen, Jie Yang, Geng‐Fu He, et al.
Acta Geotechnica (2023) Vol. 18, Iss. 7, pp. 3849-3862
Closed Access | Times Cited: 9
Xiaoxuan Chen, Jie Yang, Geng‐Fu He, et al.
Acta Geotechnica (2023) Vol. 18, Iss. 7, pp. 3849-3862
Closed Access | Times Cited: 9
A unified critical state parameter model for sand and overconsolidated clay in the framework of subloading surface theory
Kai Cui, Xiaowen Wang, Ran Yuan, et al.
Canadian Geotechnical Journal (2023) Vol. 60, Iss. 10, pp. 1461-1474
Closed Access | Times Cited: 9
Kai Cui, Xiaowen Wang, Ran Yuan, et al.
Canadian Geotechnical Journal (2023) Vol. 60, Iss. 10, pp. 1461-1474
Closed Access | Times Cited: 9
Deep learning‐accelerated multiscale approach for granular material modeling
Qingzheng Guan, Z. X. Yang, Ning Guo, et al.
International Journal for Numerical and Analytical Methods in Geomechanics (2024) Vol. 48, Iss. 5, pp. 1372-1389
Closed Access | Times Cited: 3
Qingzheng Guan, Z. X. Yang, Ning Guo, et al.
International Journal for Numerical and Analytical Methods in Geomechanics (2024) Vol. 48, Iss. 5, pp. 1372-1389
Closed Access | Times Cited: 3
Multi-parameter intelligent inverse analysis of a deep excavation considering path-dependent behavior of soils
Wenyuan Liu, Liyuan Tong, Hongjiang Li, et al.
Computers and Geotechnics (2024) Vol. 174, pp. 106597-106597
Closed Access | Times Cited: 3
Wenyuan Liu, Liyuan Tong, Hongjiang Li, et al.
Computers and Geotechnics (2024) Vol. 174, pp. 106597-106597
Closed Access | Times Cited: 3
Prediction model for the compressive strength of rock based on stacking ensemble learning and shapley additive explanations
Luyuan Wu, Jianhui Li, Jianwei Zhang, et al.
Bulletin of Engineering Geology and the Environment (2024) Vol. 83, Iss. 11
Closed Access | Times Cited: 3
Luyuan Wu, Jianhui Li, Jianwei Zhang, et al.
Bulletin of Engineering Geology and the Environment (2024) Vol. 83, Iss. 11
Closed Access | Times Cited: 3
Deep Learning Predicts Stress–Strain Relations of Granular Materials Based on Triaxial Testing Data
Tongming Qu, Shaocheng Di, Y.T. Feng, et al.
Computer Modeling in Engineering & Sciences (2021) Vol. 128, Iss. 1, pp. 129-144
Open Access | Times Cited: 22
Tongming Qu, Shaocheng Di, Y.T. Feng, et al.
Computer Modeling in Engineering & Sciences (2021) Vol. 128, Iss. 1, pp. 129-144
Open Access | Times Cited: 22
Theory-guided machine learning to predict density evolution of sand dynamically compacted under Ko condition
Amir Tophel, Jeffrey P. Walker, Troyee Tanu Dutta, et al.
Acta Geotechnica (2022) Vol. 17, Iss. 8, pp. 3479-3497
Closed Access | Times Cited: 14
Amir Tophel, Jeffrey P. Walker, Troyee Tanu Dutta, et al.
Acta Geotechnica (2022) Vol. 17, Iss. 8, pp. 3479-3497
Closed Access | Times Cited: 14
FE2 Computations with Deep Neural Networks: Algorithmic Structure, Data Generation, and Implementation
Hamidreza Eivazi, Jendrik‐Alexander Tröger, Stefan Wittek, et al.
Mathematical and Computational Applications (2023) Vol. 28, Iss. 4, pp. 91-91
Open Access | Times Cited: 8
Hamidreza Eivazi, Jendrik‐Alexander Tröger, Stefan Wittek, et al.
Mathematical and Computational Applications (2023) Vol. 28, Iss. 4, pp. 91-91
Open Access | Times Cited: 8
Prediction of mechanical behavior of rocks with strong strain-softening effects by a deep-learning approach
Lei Shi, Jin Zhang, Qizhi Zhu, et al.
Computers and Geotechnics (2022) Vol. 152, pp. 105040-105040
Closed Access | Times Cited: 13
Lei Shi, Jin Zhang, Qizhi Zhu, et al.
Computers and Geotechnics (2022) Vol. 152, pp. 105040-105040
Closed Access | Times Cited: 13
Study on triaxial test and constitutive prediction model of frozen silty clay
Zhenchao Teng, Yun-Chao Teng, Jialin Liu, et al.
Frontiers in Earth Science (2023) Vol. 10
Open Access | Times Cited: 7
Zhenchao Teng, Yun-Chao Teng, Jialin Liu, et al.
Frontiers in Earth Science (2023) Vol. 10
Open Access | Times Cited: 7
Functional order-reduced Gaussian Processes based machine-learning emulators for probabilistic constitutive modelling
Chensen Ding, Yang Chen, Hussein Rappel, et al.
Composites Part A Applied Science and Manufacturing (2023) Vol. 173, pp. 107695-107695
Closed Access | Times Cited: 7
Chensen Ding, Yang Chen, Hussein Rappel, et al.
Composites Part A Applied Science and Manufacturing (2023) Vol. 173, pp. 107695-107695
Closed Access | Times Cited: 7
A combined machine learning/search algorithm-based method for the identification of constitutive parameters from laboratory tests and in-situ tests
Changjian Zhou, Bin Gao, Bin Yan, et al.
Computers and Geotechnics (2024) Vol. 170, pp. 106268-106268
Closed Access | Times Cited: 2
Changjian Zhou, Bin Gao, Bin Yan, et al.
Computers and Geotechnics (2024) Vol. 170, pp. 106268-106268
Closed Access | Times Cited: 2
Identification of failure behaviors of underground structures under dynamic loading using machine learning
Chun Zhu, Yingze Xu, Manchao He, et al.
Journal of Rock Mechanics and Geotechnical Engineering (2024)
Open Access | Times Cited: 2
Chun Zhu, Yingze Xu, Manchao He, et al.
Journal of Rock Mechanics and Geotechnical Engineering (2024)
Open Access | Times Cited: 2
Machine Learning Methods for Geotechnical Site Characterization and Scour Assessment
Negin Yousefpour, Zhongqiang Liu, Chao Zhao
Transportation Research Record Journal of the Transportation Research Board (2024)
Open Access | Times Cited: 2
Negin Yousefpour, Zhongqiang Liu, Chao Zhao
Transportation Research Record Journal of the Transportation Research Board (2024)
Open Access | Times Cited: 2
An improved digital soil mapping approach to predict total N by combining machine learning algorithms and open environmental data
Alessandro Auzzas, Gian Franco Capra, Arun Dilipkumar Jani, et al.
Modeling Earth Systems and Environment (2024) Vol. 10, Iss. 5, pp. 6519-6538
Open Access | Times Cited: 2
Alessandro Auzzas, Gian Franco Capra, Arun Dilipkumar Jani, et al.
Modeling Earth Systems and Environment (2024) Vol. 10, Iss. 5, pp. 6519-6538
Open Access | Times Cited: 2
Physics-Informed Neural Network (PINN) model for predicting subgrade settlement induced by shield tunnelling beneath an existing railway subgrade
Guankai Wang, Shan Yao, Bettina Detmann, et al.
Transportation Geotechnics (2024), pp. 101409-101409
Closed Access | Times Cited: 2
Guankai Wang, Shan Yao, Bettina Detmann, et al.
Transportation Geotechnics (2024), pp. 101409-101409
Closed Access | Times Cited: 2
International Distribution and Development of Rock Mass Classification: A Review
Georg H. Erharter, Neil Bar, Tom F. Hansen, et al.
Rock Mechanics and Rock Engineering (2024)
Open Access | Times Cited: 2
Georg H. Erharter, Neil Bar, Tom F. Hansen, et al.
Rock Mechanics and Rock Engineering (2024)
Open Access | Times Cited: 2
Machine learning-based constitutive modelling for material non-linearity: A review
Arif Hussain, Amir Hosein Sakhaei, Mahmood Shafiee
Mechanics of Advanced Materials and Structures (2024), pp. 1-19
Open Access | Times Cited: 2
Arif Hussain, Amir Hosein Sakhaei, Mahmood Shafiee
Mechanics of Advanced Materials and Structures (2024), pp. 1-19
Open Access | Times Cited: 2
Machine learning models to estimate stress wave velocities of cohesionless soils during triaxial compression influenced by particle characteristics
Amir Tophel, Troyee Tanu Dutta, M. Otsubo, et al.
Soil Dynamics and Earthquake Engineering (2022) Vol. 165, pp. 107649-107649
Closed Access | Times Cited: 11
Amir Tophel, Troyee Tanu Dutta, M. Otsubo, et al.
Soil Dynamics and Earthquake Engineering (2022) Vol. 165, pp. 107649-107649
Closed Access | Times Cited: 11
Multi-fidelity data-driven modelling of rate-dependent behaviour of soft clays
Geng‐Fu He, Pin Zhang, Zhen‐Yu Yin, et al.
Georisk Assessment and Management of Risk for Engineered Systems and Geohazards (2022) Vol. 17, Iss. 1, pp. 64-76
Closed Access | Times Cited: 9
Geng‐Fu He, Pin Zhang, Zhen‐Yu Yin, et al.
Georisk Assessment and Management of Risk for Engineered Systems and Geohazards (2022) Vol. 17, Iss. 1, pp. 64-76
Closed Access | Times Cited: 9
Deep transfer learning-aided constitutive modelling of granular soils considering out-of-range particle morphology
Wei Xiong, Jianfeng Wang
Tunnelling and Underground Space Technology (2023) Vol. 144, pp. 105547-105547
Closed Access | Times Cited: 5
Wei Xiong, Jianfeng Wang
Tunnelling and Underground Space Technology (2023) Vol. 144, pp. 105547-105547
Closed Access | Times Cited: 5
Comparative Study of Machine Learning Approaches for Predicting Creep Behavior of Polyurethane Elastomer
Chunhao Yang, Wuning Ma, Jianlin Zhong, et al.
Polymers (2021) Vol. 13, Iss. 11, pp. 1768-1768
Open Access | Times Cited: 12
Chunhao Yang, Wuning Ma, Jianlin Zhong, et al.
Polymers (2021) Vol. 13, Iss. 11, pp. 1768-1768
Open Access | Times Cited: 12
Back-calculation of soil parameters from displacement-controlled cavity expansion under geostatic stress by FEM and machine learning
Fernando Patino-Ramirez, Zijie Jay Wang, Duen Horng Chau, et al.
Acta Geotechnica (2022) Vol. 18, Iss. 4, pp. 1755-1768
Open Access | Times Cited: 8
Fernando Patino-Ramirez, Zijie Jay Wang, Duen Horng Chau, et al.
Acta Geotechnica (2022) Vol. 18, Iss. 4, pp. 1755-1768
Open Access | Times Cited: 8
Cyclic shear stress-strain prediction of saturated sand based on the unrolled seq2seq model and scheduled sampling
Fanhua Meng, Huafu Pei
Soil Dynamics and Earthquake Engineering (2022) Vol. 165, pp. 107665-107665
Closed Access | Times Cited: 8
Fanhua Meng, Huafu Pei
Soil Dynamics and Earthquake Engineering (2022) Vol. 165, pp. 107665-107665
Closed Access | Times Cited: 8
Machine Learning in Computer Aided Engineering
Francisco J. Montáns, Elías Cueto, Klaus‐Jürgen Bathe
Computational methods in engineering & the sciences (2023), pp. 1-83
Open Access | Times Cited: 4
Francisco J. Montáns, Elías Cueto, Klaus‐Jürgen Bathe
Computational methods in engineering & the sciences (2023), pp. 1-83
Open Access | Times Cited: 4