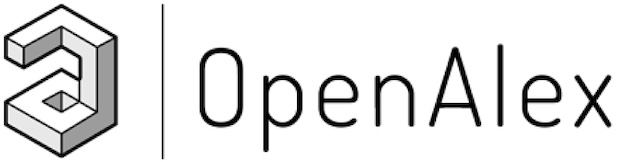
OpenAlex is a bibliographic catalogue of scientific papers, authors and institutions accessible in open access mode, named after the Library of Alexandria. It's citation coverage is excellent and I hope you will find utility in this listing of citing articles!
If you click the article title, you'll navigate to the article, as listed in CrossRef. If you click the Open Access links, you'll navigate to the "best Open Access location". Clicking the citation count will open this listing for that article. Lastly at the bottom of the page, you'll find basic pagination options.
Requested Article:
Integration of A Deep Learning Classifier with A Random Forest Approach for Predicting Malonylation Sites
Zhen Chen, Ningning He, Yu Huang, et al.
Genomics Proteomics & Bioinformatics (2018) Vol. 16, Iss. 6, pp. 451-459
Open Access | Times Cited: 90
Zhen Chen, Ningning He, Yu Huang, et al.
Genomics Proteomics & Bioinformatics (2018) Vol. 16, Iss. 6, pp. 451-459
Open Access | Times Cited: 90
Showing 26-50 of 90 citing articles:
DeepNphos: A deep-learning architecture for prediction of N-phosphorylation sites
Xulin Chang, Yafei Zhu, Yu Chen, et al.
Computers in Biology and Medicine (2024) Vol. 170, pp. 108079-108079
Closed Access | Times Cited: 4
Xulin Chang, Yafei Zhu, Yu Chen, et al.
Computers in Biology and Medicine (2024) Vol. 170, pp. 108079-108079
Closed Access | Times Cited: 4
DeepMal: Accurate prediction of protein malonylation sites by deep neural networks
Minghui Wang, Xiaoqiang Cui, Shan Li, et al.
Chemometrics and Intelligent Laboratory Systems (2020) Vol. 207, pp. 104175-104175
Closed Access | Times Cited: 31
Minghui Wang, Xiaoqiang Cui, Shan Li, et al.
Chemometrics and Intelligent Laboratory Systems (2020) Vol. 207, pp. 104175-104175
Closed Access | Times Cited: 31
iRice-MS: An integrated XGBoost model for detecting multitype post-translational modification sites in rice
Hao Lv, Yang Zhang, Jia-Shu Wang, et al.
Briefings in Bioinformatics (2021) Vol. 23, Iss. 1
Closed Access | Times Cited: 23
Hao Lv, Yang Zhang, Jia-Shu Wang, et al.
Briefings in Bioinformatics (2021) Vol. 23, Iss. 1
Closed Access | Times Cited: 23
O-GlcNAcPRED-DL: Prediction of Protein O-GlcNAcylation Sites Based on an Ensemble Model of Deep Learning
Fengzhu Hu, Weiyu Li, Yaoxiang Li, et al.
Journal of Proteome Research (2023) Vol. 23, Iss. 1, pp. 95-106
Closed Access | Times Cited: 9
Fengzhu Hu, Weiyu Li, Yaoxiang Li, et al.
Journal of Proteome Research (2023) Vol. 23, Iss. 1, pp. 95-106
Closed Access | Times Cited: 9
Accurately Predicting Glutarylation Sites Using Sequential Bi-Peptide-Based Evolutionary Features
Md. Easin Arafat, Md. Wakil Ahmad, S.M. Shovan, et al.
Genes (2020) Vol. 11, Iss. 9, pp. 1023-1023
Open Access | Times Cited: 24
Md. Easin Arafat, Md. Wakil Ahmad, S.M. Shovan, et al.
Genes (2020) Vol. 11, Iss. 9, pp. 1023-1023
Open Access | Times Cited: 24
Formator: Predicting Lysine Formylation Sites Based on the Most Distant Undersampling and Safe-Level Synthetic Minority Oversampling
Cangzhi Jia, Meng Zhang, Cunshuo Fan, et al.
IEEE/ACM Transactions on Computational Biology and Bioinformatics (2019) Vol. 18, Iss. 5, pp. 1937-1945
Closed Access | Times Cited: 25
Cangzhi Jia, Meng Zhang, Cunshuo Fan, et al.
IEEE/ACM Transactions on Computational Biology and Bioinformatics (2019) Vol. 18, Iss. 5, pp. 1937-1945
Closed Access | Times Cited: 25
RF-MaloSite and DL-Malosite: Methods based on random forest and deep learning to identify malonylation sites
Hussam J. AL-barakati, Niraj Thapa, Hiroto Saigo, et al.
Computational and Structural Biotechnology Journal (2020) Vol. 18, pp. 852-860
Open Access | Times Cited: 21
Hussam J. AL-barakati, Niraj Thapa, Hiroto Saigo, et al.
Computational and Structural Biotechnology Journal (2020) Vol. 18, pp. 852-860
Open Access | Times Cited: 21
ResSUMO: A Deep Learning Architecture Based on Residual Structure for Prediction of Lysine SUMOylation Sites
Yafei Zhu, Yuhai Liu, Yu Chen, et al.
Cells (2022) Vol. 11, Iss. 17, pp. 2646-2646
Open Access | Times Cited: 13
Yafei Zhu, Yuhai Liu, Yu Chen, et al.
Cells (2022) Vol. 11, Iss. 17, pp. 2646-2646
Open Access | Times Cited: 13
iACP-DFSRA: identification of anticancer peptides based on a dual-channel fusion strategy of ResCNN and Attention
Xin Wang, Zimeng Zhang, Chang Liu
Journal of Molecular Biology (2024), pp. 168810-168810
Closed Access | Times Cited: 2
Xin Wang, Zimeng Zhang, Chang Liu
Journal of Molecular Biology (2024), pp. 168810-168810
Closed Access | Times Cited: 2
An energy-cyber-physical system for personalized normative messaging interventions: Identification and classification of behavioral reference groups
Kwonsik Song, Kyle Anderson, Sang Hyun Lee
Applied Energy (2019) Vol. 260, pp. 114237-114237
Open Access | Times Cited: 21
Kwonsik Song, Kyle Anderson, Sang Hyun Lee
Applied Energy (2019) Vol. 260, pp. 114237-114237
Open Access | Times Cited: 21
DeepCSO: A Deep-Learning Network Approach to Predicting Cysteine S-Sulphenylation Sites
Xiaru Lyu, Shuhao Li, Chunyang Jiang, et al.
Frontiers in Cell and Developmental Biology (2020) Vol. 8
Open Access | Times Cited: 20
Xiaru Lyu, Shuhao Li, Chunyang Jiang, et al.
Frontiers in Cell and Developmental Biology (2020) Vol. 8
Open Access | Times Cited: 20
Mal-Light: Enhancing Lysine Malonylation Sites Prediction Problem Using Evolutionary-based Features
Md. Wakil Ahmad, Md. Easin Arafat, Ghazaleh Taherzadeh, et al.
IEEE Access (2020) Vol. 8, pp. 77888-77902
Open Access | Times Cited: 19
Md. Wakil Ahmad, Md. Easin Arafat, Ghazaleh Taherzadeh, et al.
IEEE Access (2020) Vol. 8, pp. 77888-77902
Open Access | Times Cited: 19
DeepKhib: A Deep-Learning Framework for Lysine 2-Hydroxyisobutyrylation Sites Prediction
Luna Zhang, Yang Zou, Ningning He, et al.
Frontiers in Cell and Developmental Biology (2020) Vol. 8
Open Access | Times Cited: 18
Luna Zhang, Yang Zou, Ningning He, et al.
Frontiers in Cell and Developmental Biology (2020) Vol. 8
Open Access | Times Cited: 18
DeepVISP: Deep Learning for Virus Site Integration Prediction and Motif Discovery
Haodong Xu, Peilin Jia, Zhongming Zhao
Advanced Science (2021) Vol. 8, Iss. 9
Open Access | Times Cited: 17
Haodong Xu, Peilin Jia, Zhongming Zhao
Advanced Science (2021) Vol. 8, Iss. 9
Open Access | Times Cited: 17
SEMal: Accurate protein malonylation site predictor using structural and evolutionary information
Shubhashis Roy Dipta, Ghazaleh Taherzadeh, Md. Wakil Ahmad, et al.
Computers in Biology and Medicine (2020) Vol. 125, pp. 104022-104022
Closed Access | Times Cited: 17
Shubhashis Roy Dipta, Ghazaleh Taherzadeh, Md. Wakil Ahmad, et al.
Computers in Biology and Medicine (2020) Vol. 125, pp. 104022-104022
Closed Access | Times Cited: 17
Incorporating hybrid models into lysine malonylation sites prediction on mammalian and plant proteins
Chia‐Ru Chung, Yaping Chang, Yu-Lin Hsu, et al.
Scientific Reports (2020) Vol. 10, Iss. 1
Open Access | Times Cited: 16
Chia‐Ru Chung, Yaping Chang, Yu-Lin Hsu, et al.
Scientific Reports (2020) Vol. 10, Iss. 1
Open Access | Times Cited: 16
CNNArginineMe: A CNN structure for training models for predicting arginine methylation sites based on the One-Hot encoding of peptide sequence
Jiaojiao Zhao, Haoqiang Jiang, Guoyang Zou, et al.
Frontiers in Genetics (2022) Vol. 13
Open Access | Times Cited: 10
Jiaojiao Zhao, Haoqiang Jiang, Guoyang Zou, et al.
Frontiers in Genetics (2022) Vol. 13
Open Access | Times Cited: 10
Prediction of post-translational modification sites using multiple kernel support vector machine
BingHua Wang, Minghui Wang, Ao Li
PeerJ (2017) Vol. 5, pp. e3261-e3261
Open Access | Times Cited: 17
BingHua Wang, Minghui Wang, Ao Li
PeerJ (2017) Vol. 5, pp. e3261-e3261
Open Access | Times Cited: 17
Uncovering social-contextual and individual mental health factors associated with violence via computational inference
Hernando Santamaría‐García, Sandra Báez, Diego Mauricio Aponte-Canencio, et al.
Patterns (2021) Vol. 2, Iss. 2, pp. 100176-100176
Open Access | Times Cited: 13
Hernando Santamaría‐García, Sandra Báez, Diego Mauricio Aponte-Canencio, et al.
Patterns (2021) Vol. 2, Iss. 2, pp. 100176-100176
Open Access | Times Cited: 13
DeepKcrot: A Deep-Learning Architecture for General and Species-Specific Lysine Crotonylation Site Prediction
Xilin Wei, Yutong Sha, Yiming Zhao, et al.
IEEE Access (2021) Vol. 9, pp. 49504-49513
Open Access | Times Cited: 13
Xilin Wei, Yutong Sha, Yiming Zhao, et al.
IEEE Access (2021) Vol. 9, pp. 49504-49513
Open Access | Times Cited: 13
ResNetKhib: a novel cell type-specific tool for predicting lysine 2-hydroxyisobutylation sites via transfer learning
Xiaoti Jia, Pei Zhao, Fuyi Li, et al.
Briefings in Bioinformatics (2023) Vol. 24, Iss. 2
Open Access | Times Cited: 5
Xiaoti Jia, Pei Zhao, Fuyi Li, et al.
Briefings in Bioinformatics (2023) Vol. 24, Iss. 2
Open Access | Times Cited: 5
MDC-Kace: A Model for Predicting Lysine Acetylation Sites Based on Modular Densely Connected Convolutional Networks
Huiqing Wang, Zhiliang Yan, Dan Liu, et al.
IEEE Access (2020) Vol. 8, pp. 214469-214480
Open Access | Times Cited: 14
Huiqing Wang, Zhiliang Yan, Dan Liu, et al.
IEEE Access (2020) Vol. 8, pp. 214469-214480
Open Access | Times Cited: 14
DeepSADPr: A hybrid-learning architecture for serine ADP-ribosylation site prediction
Yutong Sha, Chenglong Ma, Xilin Wei, et al.
Methods (2021) Vol. 203, pp. 575-583
Closed Access | Times Cited: 11
Yutong Sha, Chenglong Ma, Xilin Wei, et al.
Methods (2021) Vol. 203, pp. 575-583
Closed Access | Times Cited: 11
Current computational tools for protein lysine acylation site prediction
Zhaohui Qin, Haoran Ren, Pei Zhao, et al.
Briefings in Bioinformatics (2024) Vol. 25, Iss. 6
Closed Access | Times Cited: 1
Zhaohui Qin, Haoran Ren, Pei Zhao, et al.
Briefings in Bioinformatics (2024) Vol. 25, Iss. 6
Closed Access | Times Cited: 1
mUSP: a high-accuracy map of thein situcrosstalk of ubiquitylation and SUMOylation proteome predicted via the feature enhancement approach
Haodong Xu, Ru‐Ping Liang, You‐Gan Wang, et al.
Briefings in Bioinformatics (2020) Vol. 22, Iss. 3
Closed Access | Times Cited: 11
Haodong Xu, Ru‐Ping Liang, You‐Gan Wang, et al.
Briefings in Bioinformatics (2020) Vol. 22, Iss. 3
Closed Access | Times Cited: 11