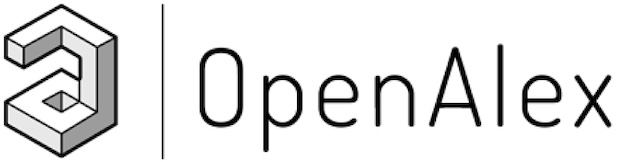
OpenAlex is a bibliographic catalogue of scientific papers, authors and institutions accessible in open access mode, named after the Library of Alexandria. It's citation coverage is excellent and I hope you will find utility in this listing of citing articles!
If you click the article title, you'll navigate to the article, as listed in CrossRef. If you click the Open Access links, you'll navigate to the "best Open Access location". Clicking the citation count will open this listing for that article. Lastly at the bottom of the page, you'll find basic pagination options.
Requested Article:
The promise of artificial intelligence in chemical engineering: Is it here, finally?
Venkat Venkatasubramanian
AIChE Journal (2018) Vol. 65, Iss. 2, pp. 466-478
Open Access | Times Cited: 502
Venkat Venkatasubramanian
AIChE Journal (2018) Vol. 65, Iss. 2, pp. 466-478
Open Access | Times Cited: 502
Showing 26-50 of 502 citing articles:
Embracing data science in catalysis research
Manu Suvarna, Javier Pérez‐Ramírez
Nature Catalysis (2024) Vol. 7, Iss. 6, pp. 624-635
Closed Access | Times Cited: 16
Manu Suvarna, Javier Pérez‐Ramírez
Nature Catalysis (2024) Vol. 7, Iss. 6, pp. 624-635
Closed Access | Times Cited: 16
Explainable AI models for predicting drop coalescence in microfluidics device
Jin-Wei Hu, Kewei Zhu, Sibo Cheng, et al.
Chemical Engineering Journal (2024) Vol. 481, pp. 148465-148465
Open Access | Times Cited: 13
Jin-Wei Hu, Kewei Zhu, Sibo Cheng, et al.
Chemical Engineering Journal (2024) Vol. 481, pp. 148465-148465
Open Access | Times Cited: 13
Machine learning for industrial sensing and control: A survey and practical perspective
Nathan P. Lawrence, Seshu Kumar Damarla, Jong Woo Kim, et al.
Control Engineering Practice (2024) Vol. 145, pp. 105841-105841
Open Access | Times Cited: 13
Nathan P. Lawrence, Seshu Kumar Damarla, Jong Woo Kim, et al.
Control Engineering Practice (2024) Vol. 145, pp. 105841-105841
Open Access | Times Cited: 13
“Inverting” X-ray Absorption Spectra of Catalysts by Machine Learning in Search for Activity Descriptors
Janis Timoshenko, Anatoly I. Frenkel
ACS Catalysis (2019) Vol. 9, Iss. 11, pp. 10192-10211
Open Access | Times Cited: 134
Janis Timoshenko, Anatoly I. Frenkel
ACS Catalysis (2019) Vol. 9, Iss. 11, pp. 10192-10211
Open Access | Times Cited: 134
Machine Learning for Accelerated Discovery of Solar Photocatalysts
Hassan Masood, Cui Ying Toe, Wey Yang Teoh, et al.
ACS Catalysis (2019) Vol. 9, Iss. 12, pp. 11774-11787
Closed Access | Times Cited: 132
Hassan Masood, Cui Ying Toe, Wey Yang Teoh, et al.
ACS Catalysis (2019) Vol. 9, Iss. 12, pp. 11774-11787
Closed Access | Times Cited: 132
The behavior of suspensions and macromolecular solutions in crossflow microfiltration: An update
Jia Wei Chew, James E. Kilduff, Georges Belfort
Journal of Membrane Science (2020) Vol. 601, pp. 117865-117865
Open Access | Times Cited: 119
Jia Wei Chew, James E. Kilduff, Georges Belfort
Journal of Membrane Science (2020) Vol. 601, pp. 117865-117865
Open Access | Times Cited: 119
Deep hybrid modeling of chemical process: Application to hydraulic fracturing
Mohammed Saad Faizan Bangi, Joseph Sang‐Il Kwon
Computers & Chemical Engineering (2019) Vol. 134, pp. 106696-106696
Open Access | Times Cited: 117
Mohammed Saad Faizan Bangi, Joseph Sang‐Il Kwon
Computers & Chemical Engineering (2019) Vol. 134, pp. 106696-106696
Open Access | Times Cited: 117
Machine learning to assist filtered two‐fluid model development for dense gas–particle flows
Li‐Tao Zhu, Steven Tang, Zheng‐Hong Luo
AIChE Journal (2020) Vol. 66, Iss. 6
Closed Access | Times Cited: 111
Li‐Tao Zhu, Steven Tang, Zheng‐Hong Luo
AIChE Journal (2020) Vol. 66, Iss. 6
Closed Access | Times Cited: 111
A Review of Kernel Methods for Feature Extraction in Nonlinear Process Monitoring
Karl Ezra Pilario, Mahmood Shafiee, Yi Cao, et al.
Processes (2019) Vol. 8, Iss. 1, pp. 24-24
Open Access | Times Cited: 102
Karl Ezra Pilario, Mahmood Shafiee, Yi Cao, et al.
Processes (2019) Vol. 8, Iss. 1, pp. 24-24
Open Access | Times Cited: 102
Understanding and optimization of thin film nanocomposite membranes for reverse osmosis with machine learning
Chester Su Hern Yeo, Qian Xie, Xiaonan Wang, et al.
Journal of Membrane Science (2020) Vol. 606, pp. 118135-118135
Closed Access | Times Cited: 98
Chester Su Hern Yeo, Qian Xie, Xiaonan Wang, et al.
Journal of Membrane Science (2020) Vol. 606, pp. 118135-118135
Closed Access | Times Cited: 98
Machine Learning in Thermodynamics: Prediction of Activity Coefficients by Matrix Completion
Fabian Jirasek, Rodrigo Alves, Julie Damay, et al.
The Journal of Physical Chemistry Letters (2020) Vol. 11, Iss. 3, pp. 981-985
Open Access | Times Cited: 77
Fabian Jirasek, Rodrigo Alves, Julie Damay, et al.
The Journal of Physical Chemistry Letters (2020) Vol. 11, Iss. 3, pp. 981-985
Open Access | Times Cited: 77
A multi-layered view of chemical and biochemical engineering
Rafiqul Gani, J. Bałdyga, Béatrice Biscans, et al.
Process Safety and Environmental Protection (2020) Vol. 155, pp. A133-A145
Open Access | Times Cited: 73
Rafiqul Gani, J. Bałdyga, Béatrice Biscans, et al.
Process Safety and Environmental Protection (2020) Vol. 155, pp. A133-A145
Open Access | Times Cited: 73
A computational workflow to study particle transport and filtration in porous media: Coupling CFD and deep learning
Agnese Marcato, Gianluca Boccardo, Daniele Marchisio
Chemical Engineering Journal (2021) Vol. 417, pp. 128936-128936
Open Access | Times Cited: 66
Agnese Marcato, Gianluca Boccardo, Daniele Marchisio
Chemical Engineering Journal (2021) Vol. 417, pp. 128936-128936
Open Access | Times Cited: 66
Conventional anddata‐driven modeling of filtered drag, heat transfer, and reaction rate ingas–particle flows
Li‐Tao Zhu, Bo Ouyang, He Lei, et al.
AIChE Journal (2021) Vol. 67, Iss. 8
Closed Access | Times Cited: 66
Li‐Tao Zhu, Bo Ouyang, He Lei, et al.
AIChE Journal (2021) Vol. 67, Iss. 8
Closed Access | Times Cited: 66
Optimization of Formulations Using Robotic Experiments Driven by Machine Learning DoE
Liwei Cao, Danilo Russo, Kobi Felton, et al.
Cell Reports Physical Science (2021) Vol. 2, Iss. 1, pp. 100295-100295
Open Access | Times Cited: 61
Liwei Cao, Danilo Russo, Kobi Felton, et al.
Cell Reports Physical Science (2021) Vol. 2, Iss. 1, pp. 100295-100295
Open Access | Times Cited: 61
An extended Tennessee Eastman simulation dataset for fault-detection and decision support systems
Christopher Reinartz, Murat Külahçı, Ole Ravn
Computers & Chemical Engineering (2021) Vol. 149, pp. 107281-107281
Closed Access | Times Cited: 61
Christopher Reinartz, Murat Külahçı, Ole Ravn
Computers & Chemical Engineering (2021) Vol. 149, pp. 107281-107281
Closed Access | Times Cited: 61
Industrial data science – a review of machine learning applications for chemical and process industries
Max Mowbray, Mattia Vallerio, Carlos Perez-Galvan, et al.
Reaction Chemistry & Engineering (2022) Vol. 7, Iss. 7, pp. 1471-1509
Open Access | Times Cited: 59
Max Mowbray, Mattia Vallerio, Carlos Perez-Galvan, et al.
Reaction Chemistry & Engineering (2022) Vol. 7, Iss. 7, pp. 1471-1509
Open Access | Times Cited: 59
Maximizing information from chemical engineering data sets: Applications to machine learning
Alexander Thebelt, Johannes Wiebe, Jan Kronqvist, et al.
Chemical Engineering Science (2022) Vol. 252, pp. 117469-117469
Open Access | Times Cited: 57
Alexander Thebelt, Johannes Wiebe, Jan Kronqvist, et al.
Chemical Engineering Science (2022) Vol. 252, pp. 117469-117469
Open Access | Times Cited: 57
Hybrid differential equations: Integrating mechanistic and data-driven techniques for modelling of water systems
Ward Quaghebeur, Elena Torfs, Bernard De Baets, et al.
Water Research (2022) Vol. 213, pp. 118166-118166
Closed Access | Times Cited: 50
Ward Quaghebeur, Elena Torfs, Bernard De Baets, et al.
Water Research (2022) Vol. 213, pp. 118166-118166
Closed Access | Times Cited: 50
Review of interpretable machine learning for process industries
A. Carter, Syed Imtiaz, G.F. Naterer
Process Safety and Environmental Protection (2022) Vol. 170, pp. 647-659
Closed Access | Times Cited: 48
A. Carter, Syed Imtiaz, G.F. Naterer
Process Safety and Environmental Protection (2022) Vol. 170, pp. 647-659
Closed Access | Times Cited: 48
Artificial intelligence enabled efficient power generation and emissions reduction underpinning net-zero goal from the coal-based power plants
Waqar Muhammad Ashraf, Ghulam Moeen Uddin, Hassan Afroze Ahmad, et al.
Energy Conversion and Management (2022) Vol. 268, pp. 116025-116025
Open Access | Times Cited: 42
Waqar Muhammad Ashraf, Ghulam Moeen Uddin, Hassan Afroze Ahmad, et al.
Energy Conversion and Management (2022) Vol. 268, pp. 116025-116025
Open Access | Times Cited: 42
Integration of machine learning and first principles models
Lokesh K. Rajulapati, Sivadurgaprasad Chinta, Bala Shyamala, et al.
AIChE Journal (2022) Vol. 68, Iss. 6
Closed Access | Times Cited: 40
Lokesh K. Rajulapati, Sivadurgaprasad Chinta, Bala Shyamala, et al.
AIChE Journal (2022) Vol. 68, Iss. 6
Closed Access | Times Cited: 40
From Computational Fluid Dynamics to Structure Interpretation via Neural Networks: An Application to Flow and Transport in Porous Media
Agnese Marcato, Gianluca Boccardo, Daniele Marchisio
Industrial & Engineering Chemistry Research (2022) Vol. 61, Iss. 24, pp. 8530-8541
Open Access | Times Cited: 38
Agnese Marcato, Gianluca Boccardo, Daniele Marchisio
Industrial & Engineering Chemistry Research (2022) Vol. 61, Iss. 24, pp. 8530-8541
Open Access | Times Cited: 38
Optimization and Scale-Up of Fermentation Processes Driven by Models
Yuan‐Hang Du, Min-Yu Wang, Linhui Yang, et al.
Bioengineering (2022) Vol. 9, Iss. 9, pp. 473-473
Open Access | Times Cited: 38
Yuan‐Hang Du, Min-Yu Wang, Linhui Yang, et al.
Bioengineering (2022) Vol. 9, Iss. 9, pp. 473-473
Open Access | Times Cited: 38
Learning from flowsheets: A generative transformer model for autocompletion of flowsheets
Gabriel Vogel, Lukas Schulze Balhorn, Artur M. Schweidtmann
Computers & Chemical Engineering (2023) Vol. 171, pp. 108162-108162
Open Access | Times Cited: 35
Gabriel Vogel, Lukas Schulze Balhorn, Artur M. Schweidtmann
Computers & Chemical Engineering (2023) Vol. 171, pp. 108162-108162
Open Access | Times Cited: 35